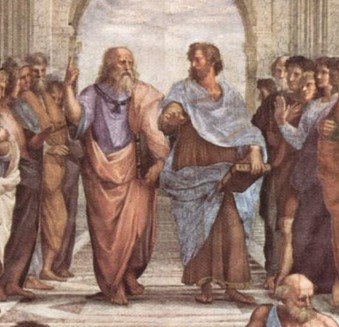
CAUSALI-T-AI
When causality and AI teams up to enhance interpretability and robustness of AI algorithms
Preview
Develop causal based approaches, to enhance AI algorithms and to make it more robust and more explainable.
Marianne Clausel, Professor at Université de Lorraine
The CAUSALI-T-AI project aims at promoting the idea that causal modeling can significantly contribute as a methodology to guarantee the soundness of the AI cycle: from data to models, from models to decisions, from decisions to data. And make AI algorithms more robust and more explainable.
The main scientific challenges are causal representation earning, as well as causal inference. The targeted applications are multiple and range from personalized medicine to the economy, including energy and the environment.
Keywords : AI, causal modelling, machine learning (ML)
Project web site : https://sites.google.com/view/causali-t-ai/home?authuser=0
Missions
Our researches
Investigate causal representation learning
Discover latent structures and define causal relevant representation to enhance the performance of ML tasks
Combine causal inference and domain adaptation
Develop methods allowing to adapt causal models from a source domain to target ones, propose targeted interventions, (algorithmic recourse).
Investigate causal inference in uncertain environment
Develop methods allowing to answer counterfactual questions in uncertain environment
Define causal benchmarks for research purpose
Reference all open source codes implemented during the project
Consortium
Université Grenoble Alpes, Université de Lorraine, Université Paris-Saclay, CNRS, INRIA
The main goal of the project is to develop new causal approaches, integrating the latest advances in the field of AI.
The members consortium intend to seize the opportunity offered by the project to create synergy between researchers in the field of causality and practitioners.
Causal approaches allow to deep understanding of the phenomena that are observed, paving the way to the exploration of alternative – called counterfactuel scenarios – to what has been observed.
These characteristics of causal modeling make it a fundamental question at the heart of many scientific fields, notably in physics, medicine and economics.
The aim is also to promote the interaction of different academic and industrial communities in Europe around causality.
A scientific community of 6 researchers, 7 university staff, 8 PhD students and 4 post-doctoral students as the project progresses.
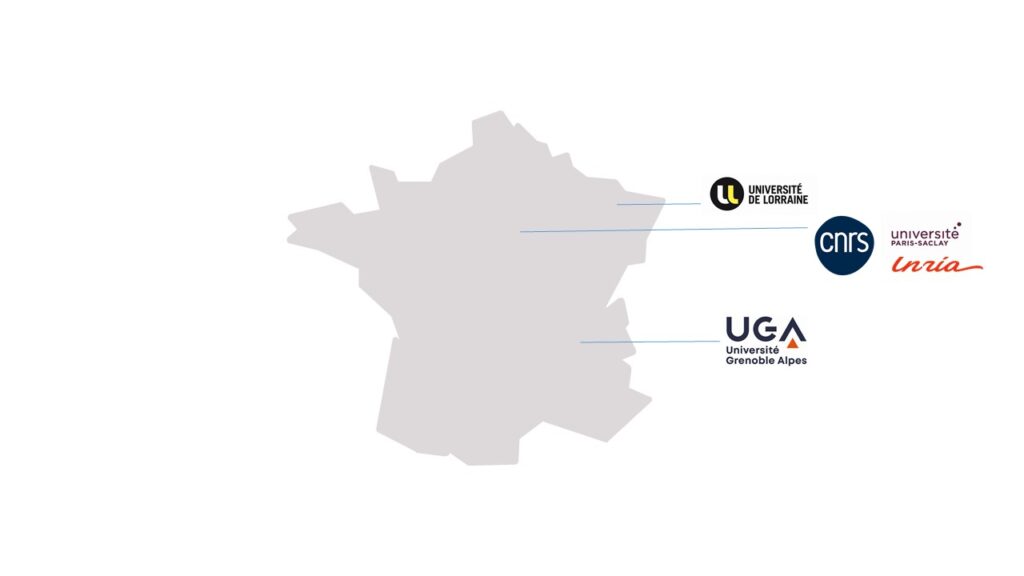
Publication
Autres projets
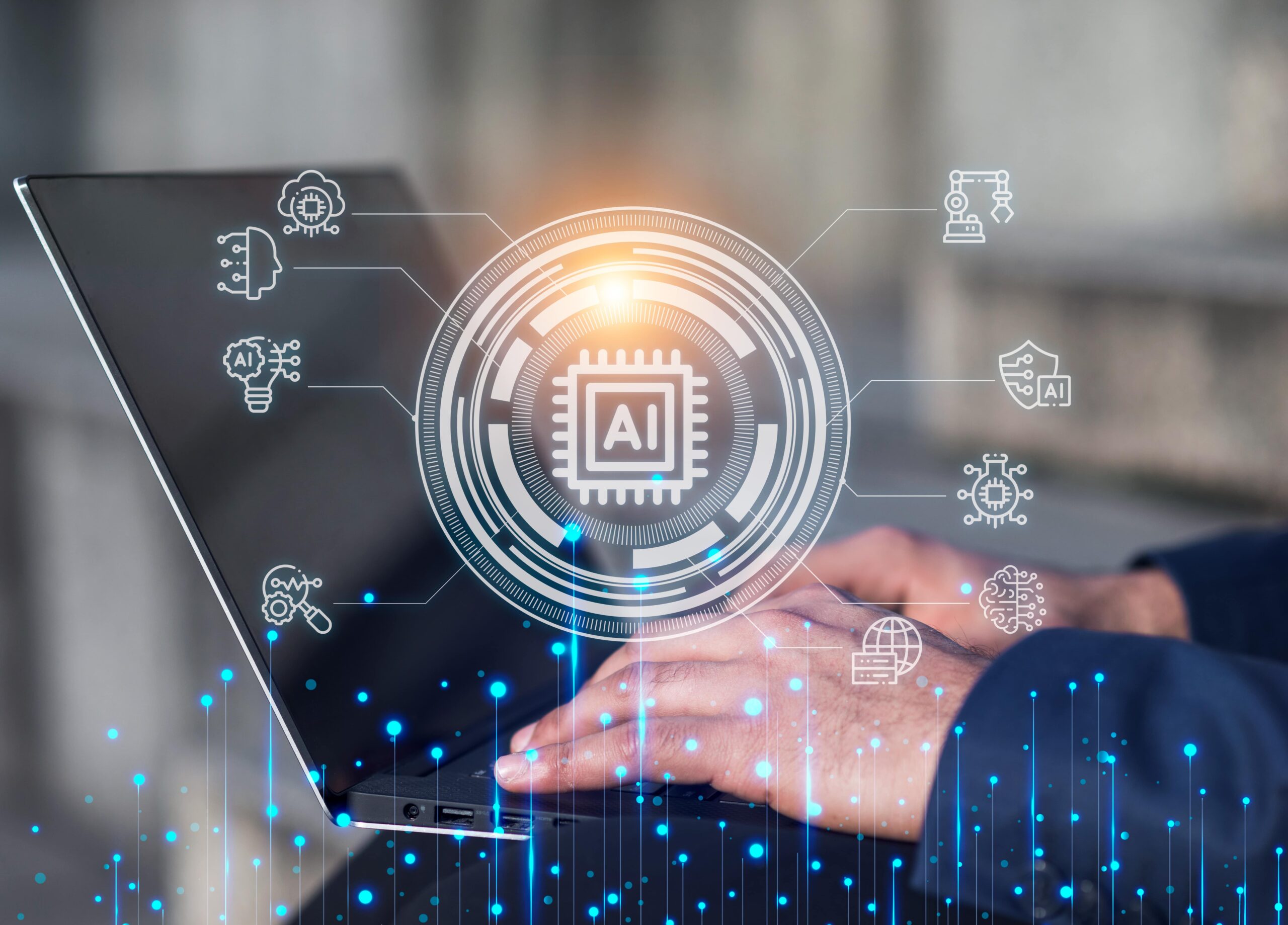
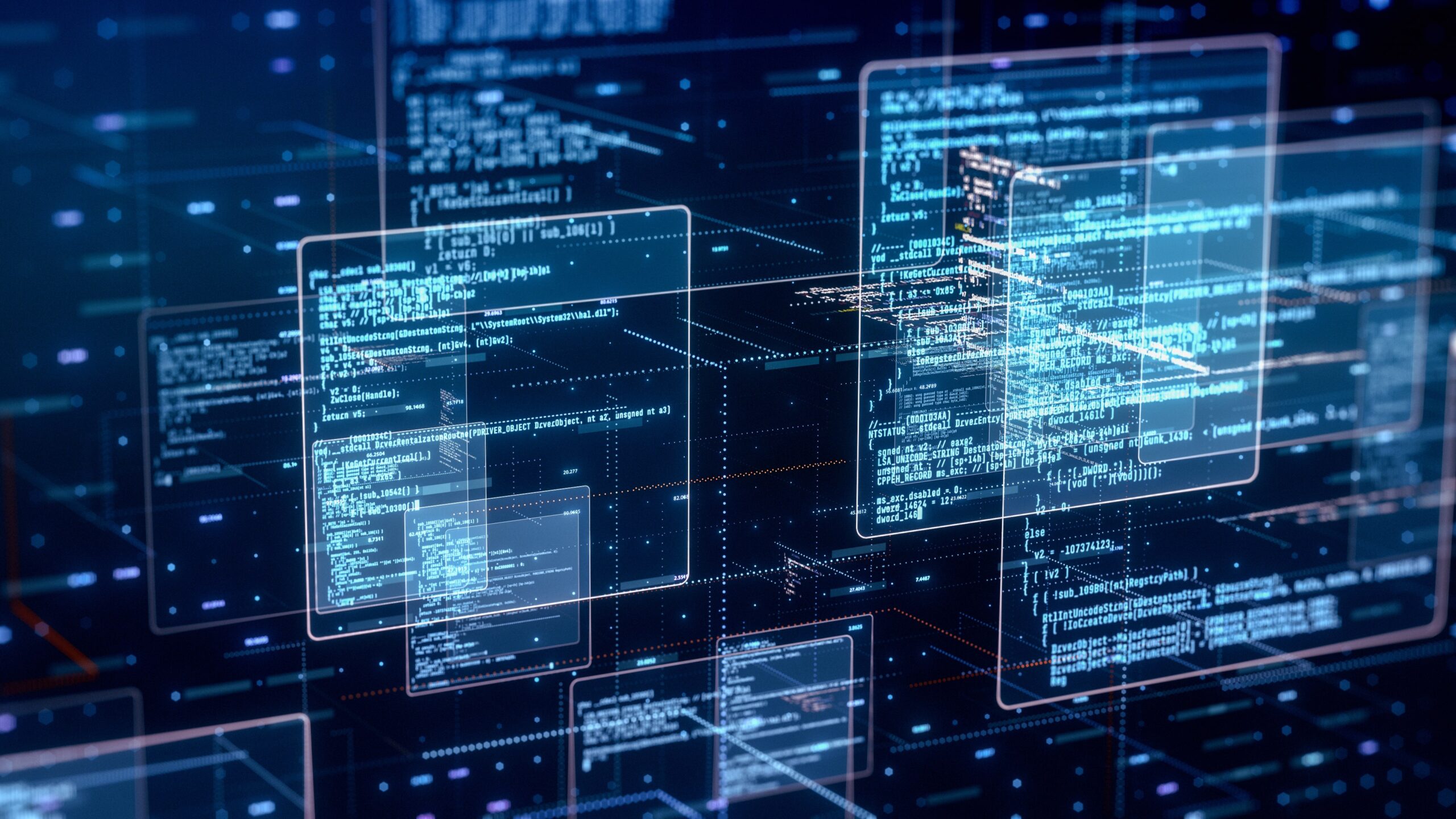
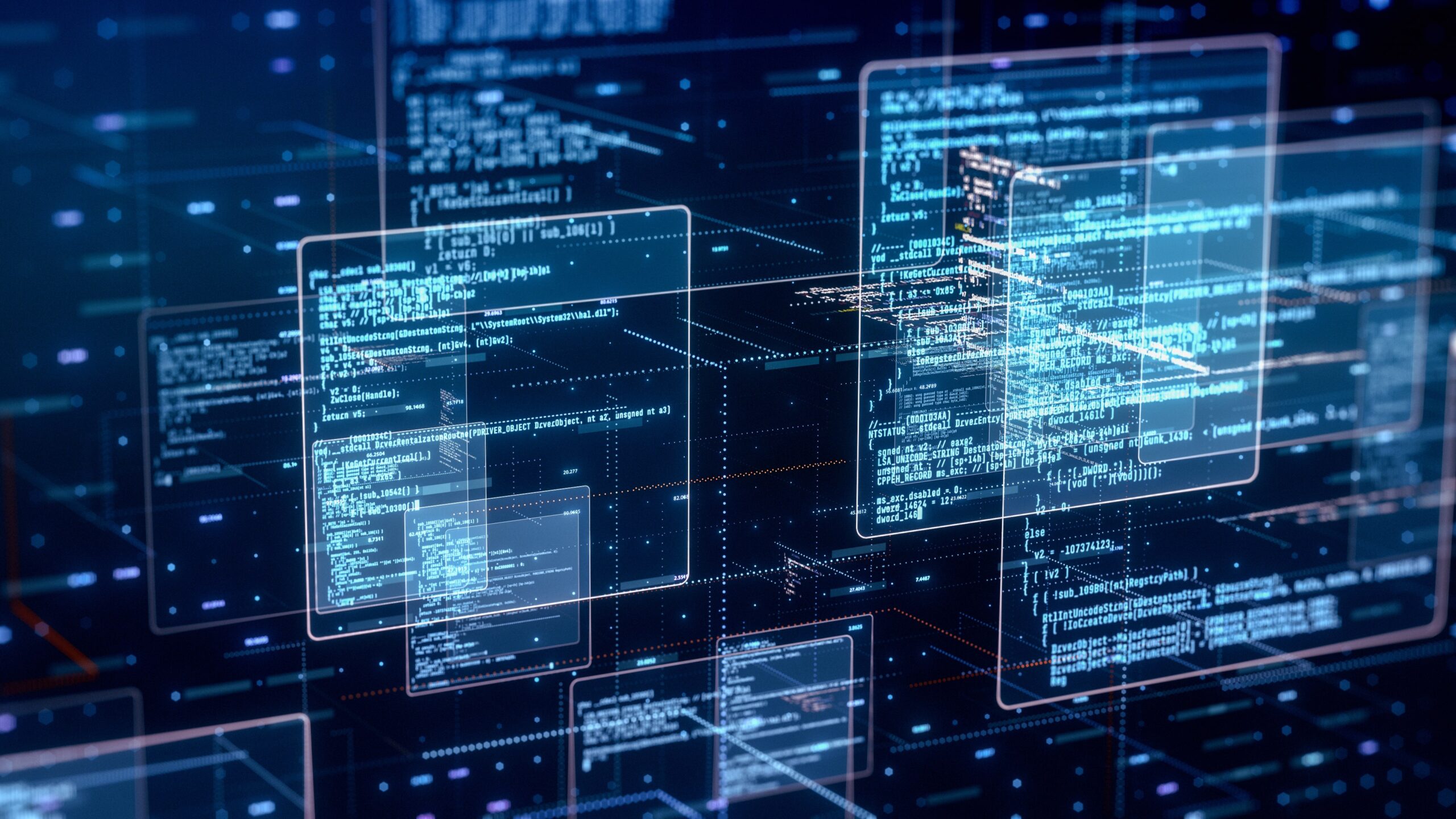
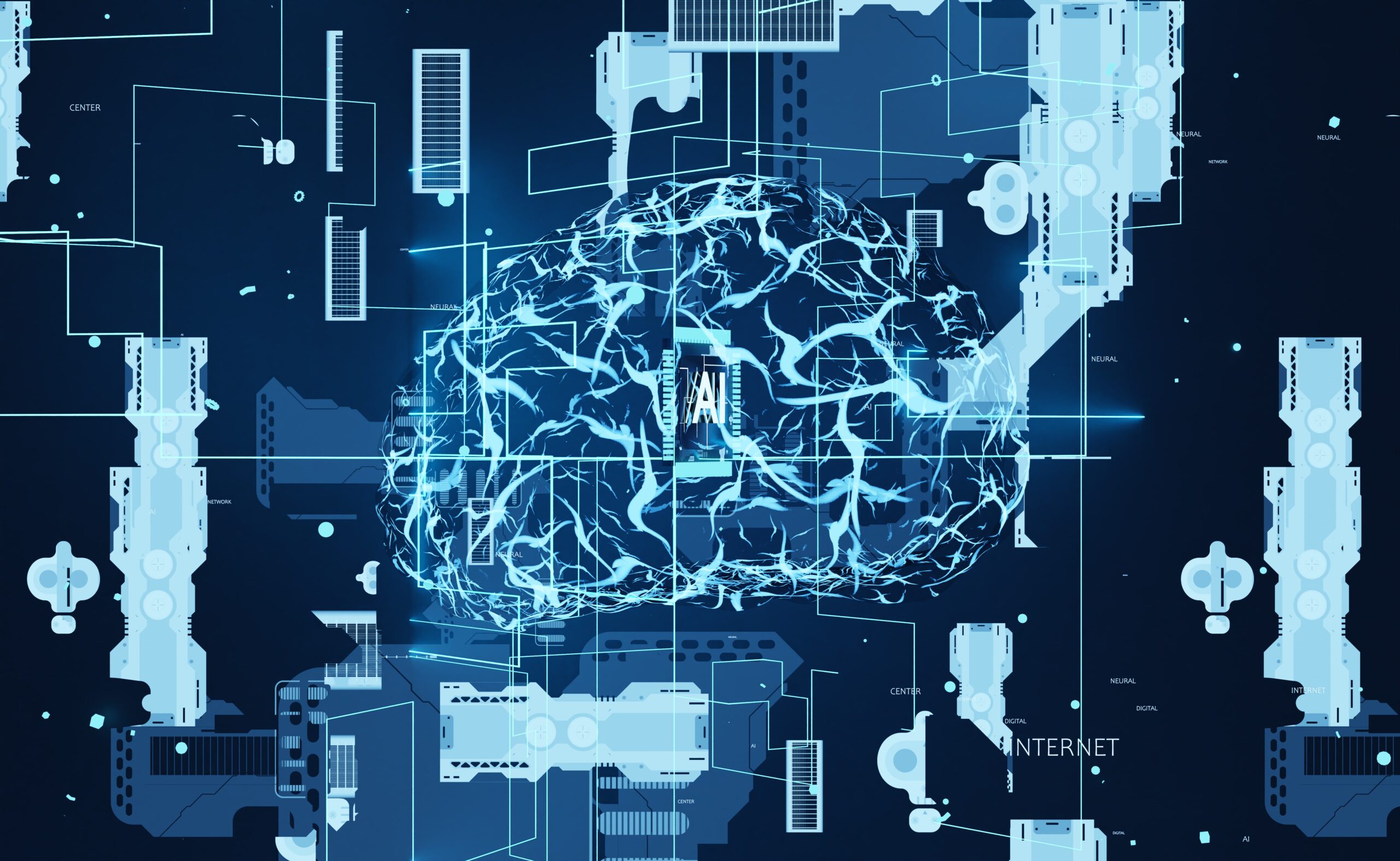
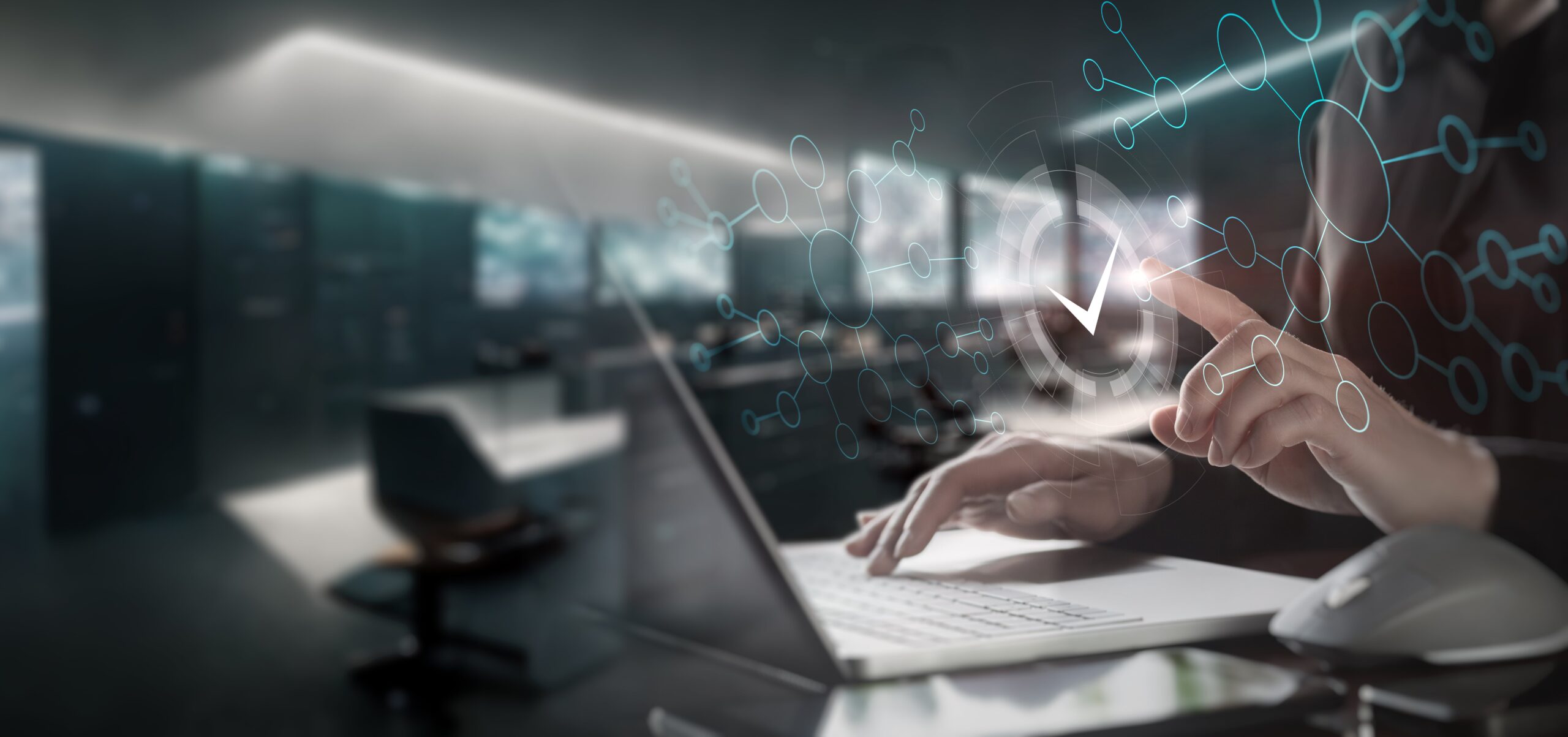
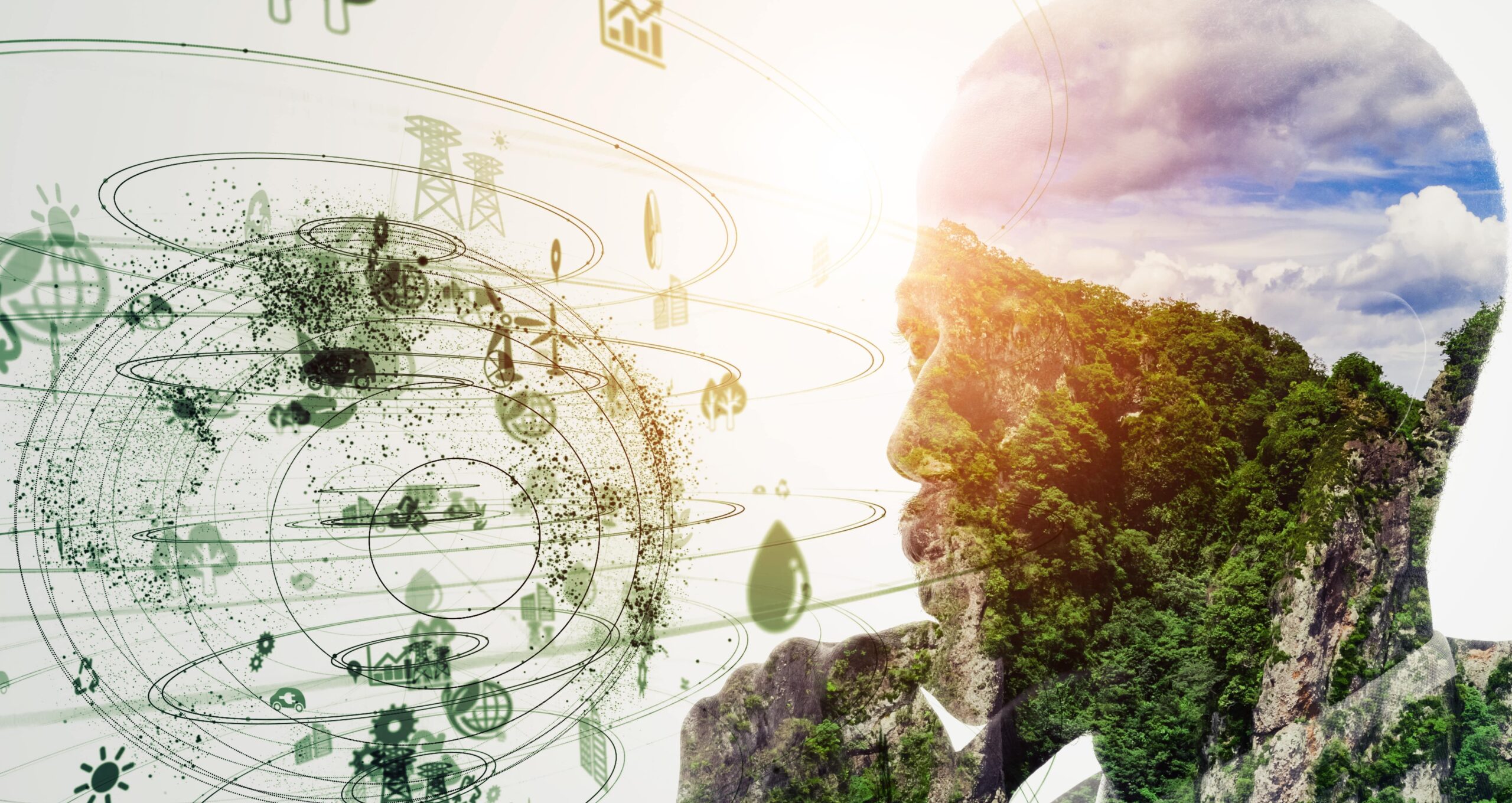
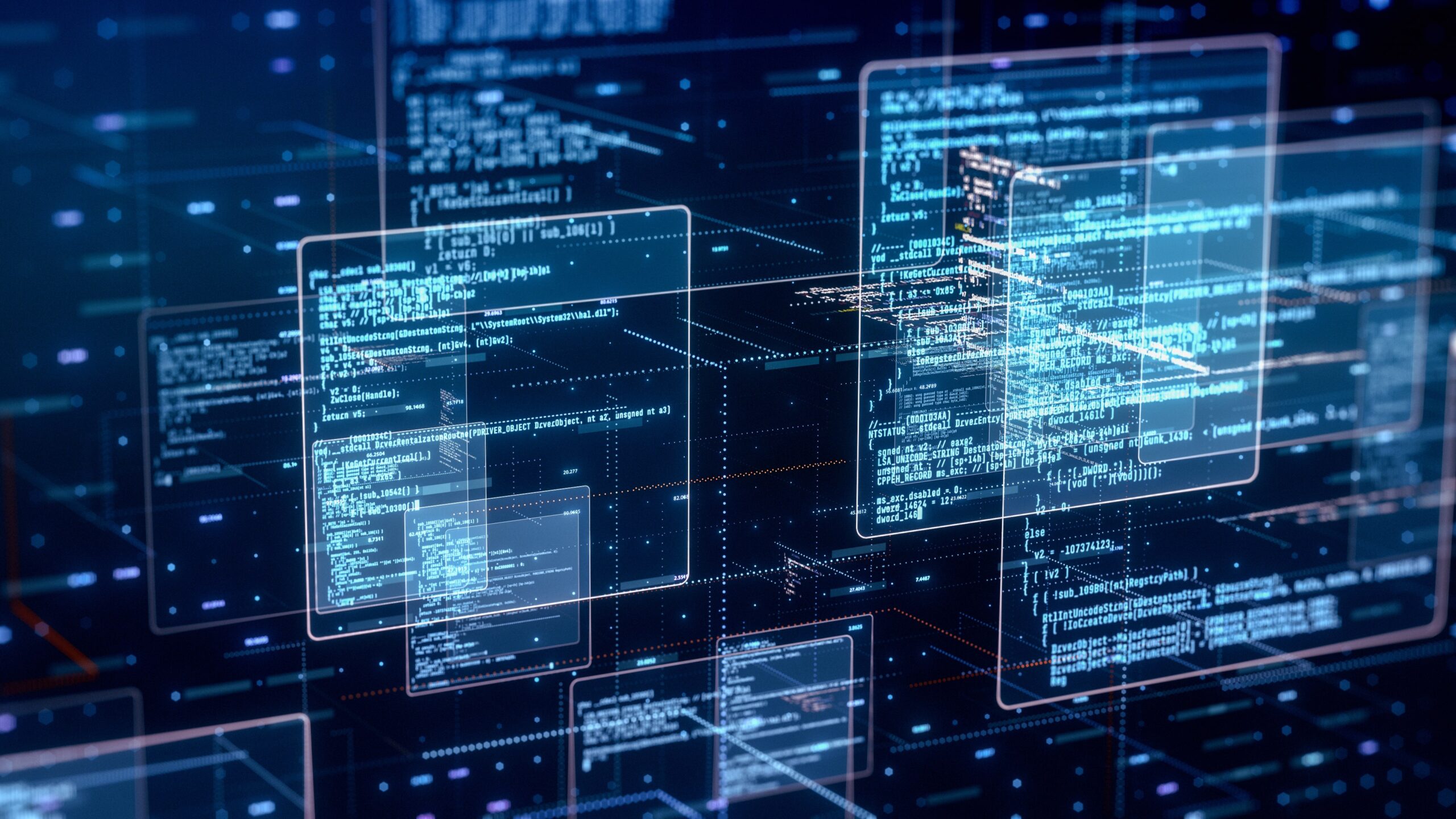
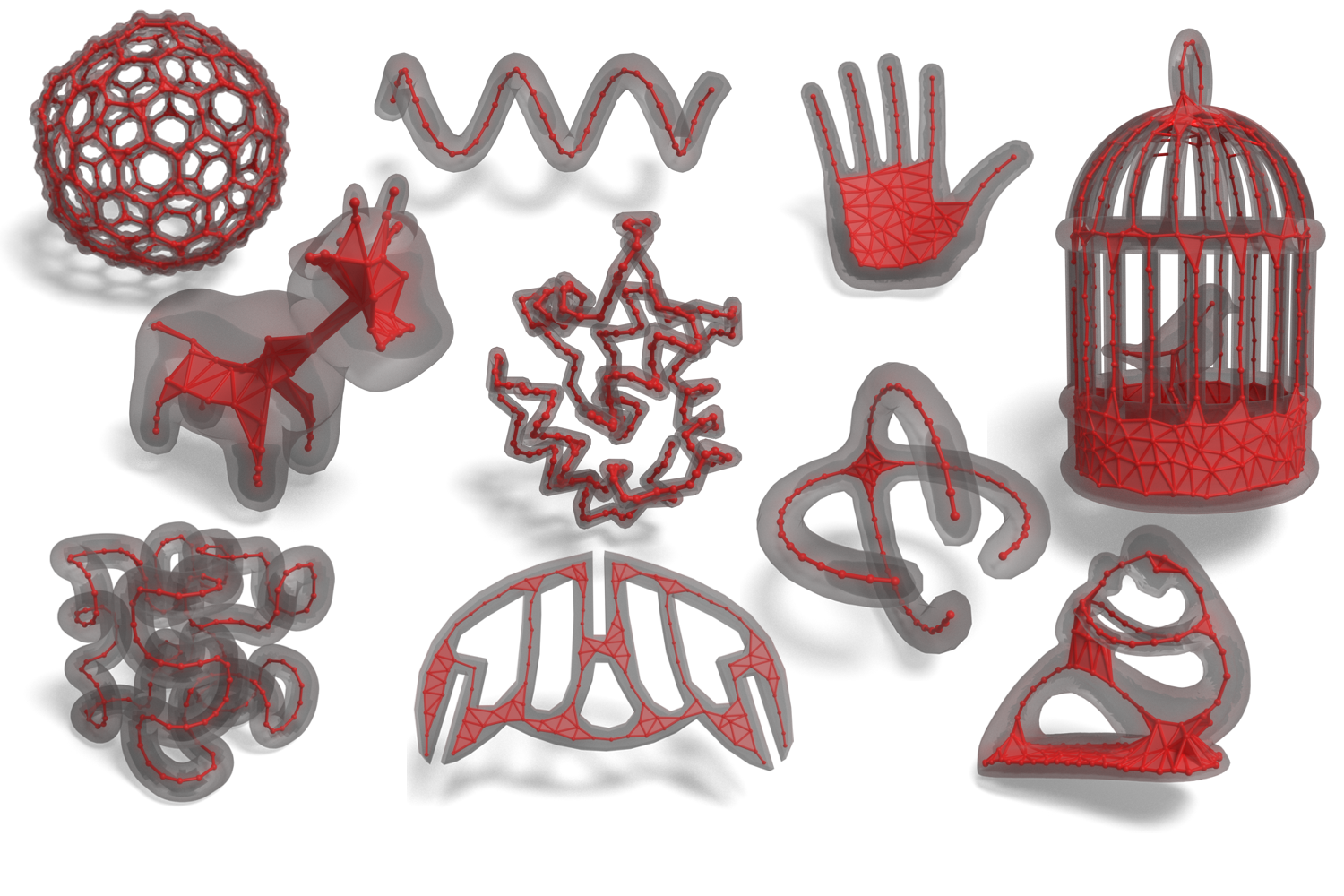
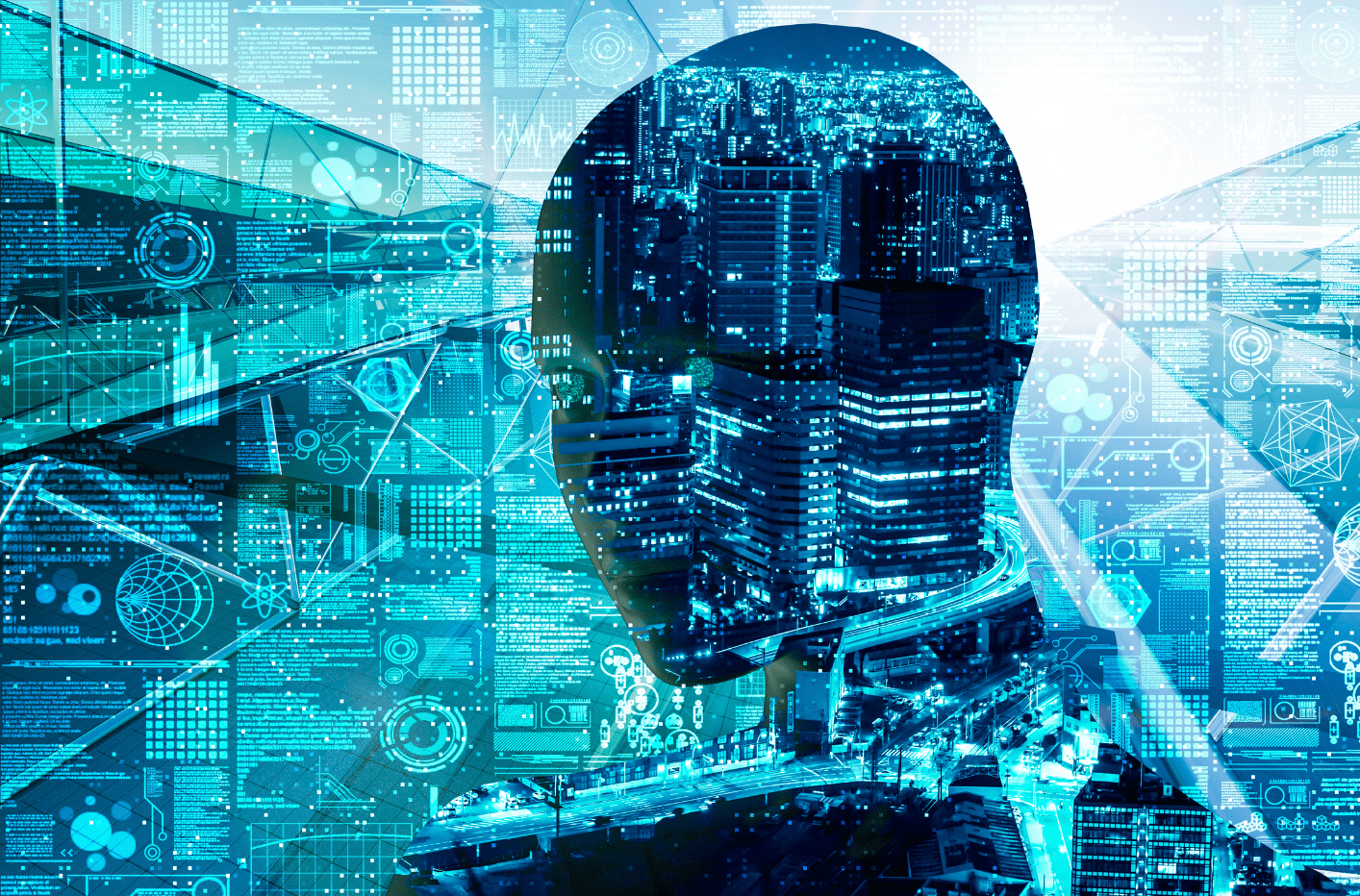
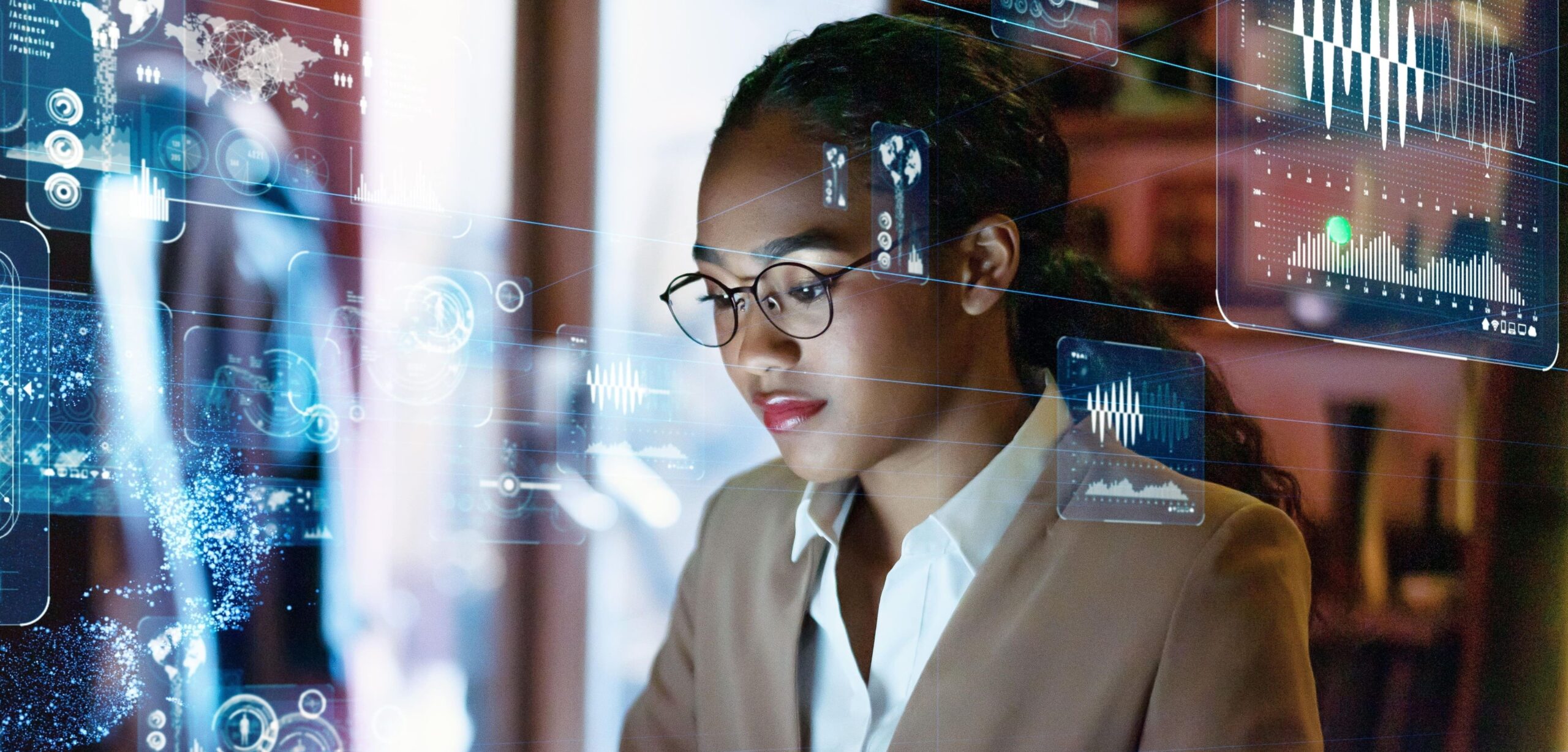
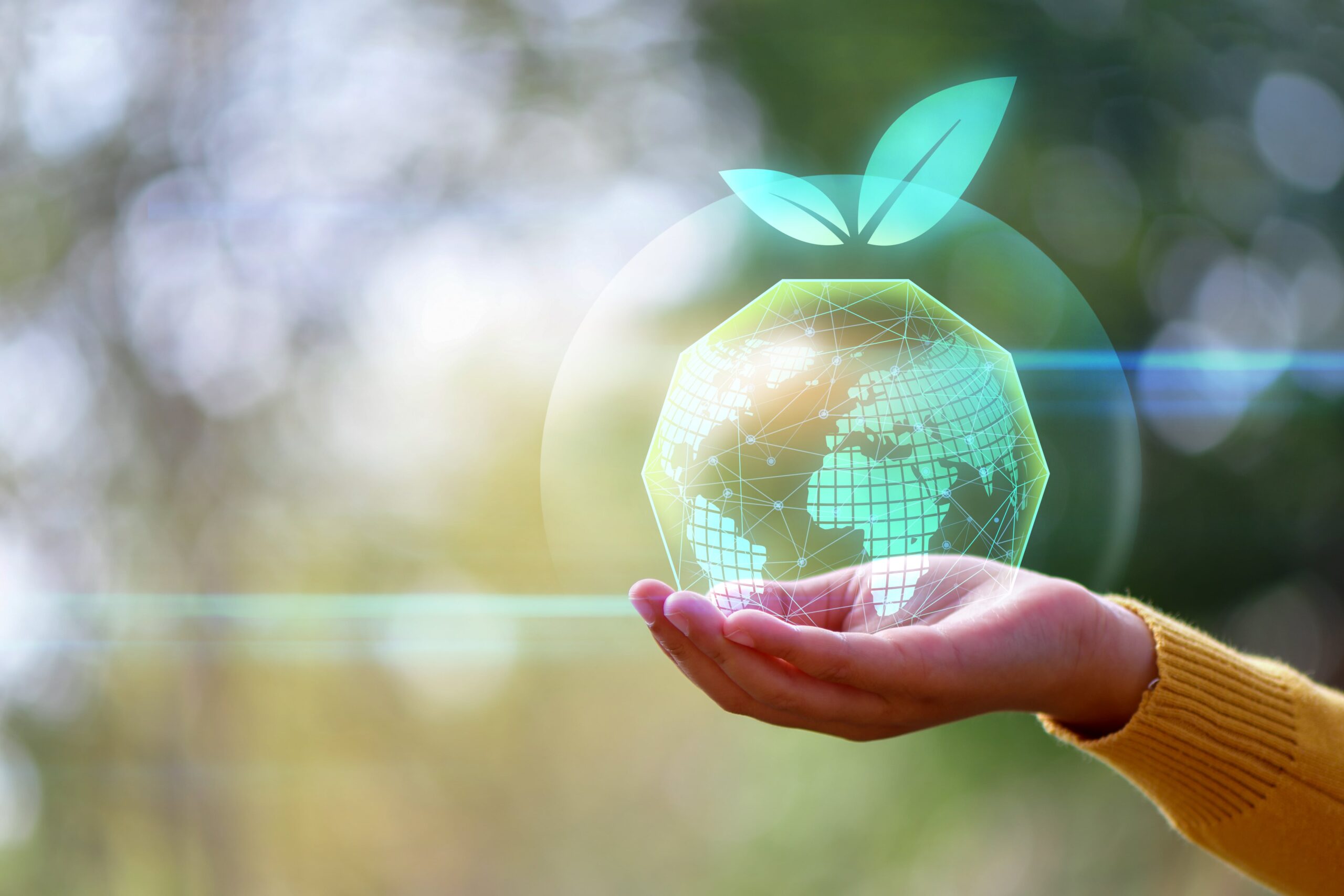