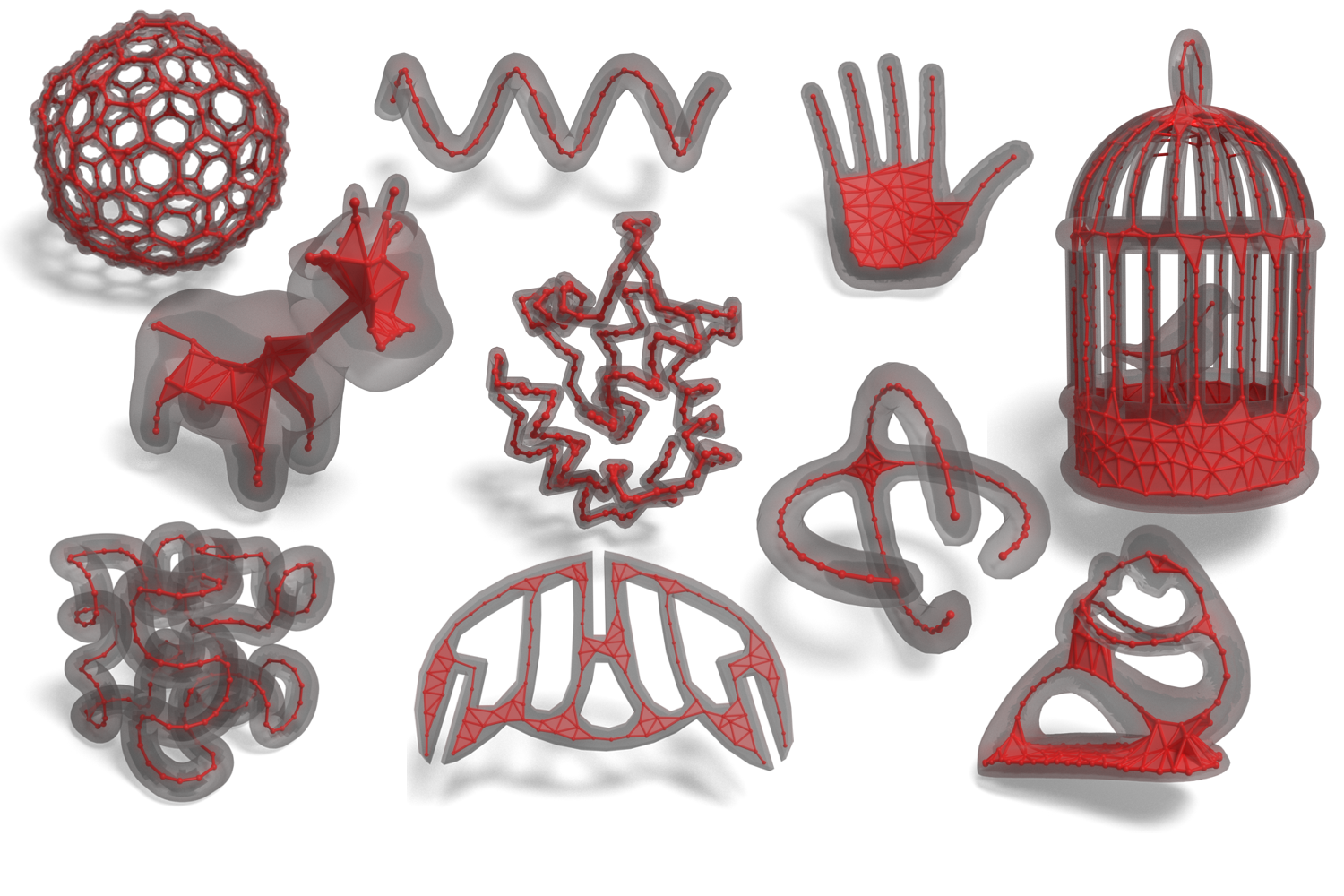
PDE-AI
Numerical analysis, optimal control and optimal transport for AI : new architectures for machine learning
Preview
The aim is to develop mathematical analysis methods (numerical analysis, control, optimal transport) for the study and the improvement of artificial intelligence systems, and to develop rigorous, reliable AI for numerical analysis and simulation.
Antonin Chambolle, Research director at CNRS
The main aim of the FOUNDRY project is to support the creation of a group of applied mathematicians specializing in machine learning issues, and to stimulate the cross-over between mathematical analysis, optimal control and optimal transport, leading to new architectures for machine learning models. Three main themes are being investigated: a first theme focuses on the analysis of learning methods, a second on new deep architectures (where specific architectures for numerical simulation will be studied in particular), and a final research angle focusing on generative methods and diffusion from an analytical point of view.
Keywords : Nonlinear analysis, partial differential equations (PDE), numerical analysis, control, optimization, machine learning, neural networks
Project web site : https://pde-ai.math.cnrs.fr/
Missions
Our researches
Study and better understand neural network training dynamics and top-down gradient optimizations chemes
Study the various networks (e.g. residuals, transformers) seen as approximations to Partial Differential Equations (PDEs) that emerge as mean-field equations for neural networks with a large number of neurons. Work towards a better understanding of learning dynamics, based on gradient flows
Design new deep architectures, based on the analysis of PDEs, including the development/analysis of AI techniques for simulation and numerical analysis
Analyze the approximation and stability properties of networks with techniques drawn from the analysis of PDE discretization schemes, while developing the use of AI for numerical simulation
Study generative methods and diffusion from an analytical point of view, exploiting the analogy with dynamical systems and partial differential equations
Understand the ability of generative networks to model disconnected or multimodal distributions, in order to define statistical bounds on the optimal transport distance to the target distribution. In addition to theoretical guarantees, new sampling strategies can be developed based on gradient stream theory for particle ensembles, in optimal transport metrics
Consortium
Université Paris Sciences et Lettres, Université Paris-Dauphine, CNRS, INRIA (Nice), Université Paris-Cité, Sorbonne Université, Université Paris-Saclay, Institut National Polytechnique de Toulouse, Université de Bordeaux, CREST-GENEST (ENSAE), Université de Strasbourg, Université Paris 1 Panthéon-Sorbonne, Ecole Nationale de l’aviation civile, Université Toulouse Capitole, Université Toulouse 3 Paul Sabatier, Université de Lyon 1, INSA Lyon, Université de Côte d’Azur
This project is cross-disciplinary in nature: it will develop theoretical and algorithmic tools that will find applications in other fields covered by PEPR IA. Indeed, the aim is to build new mathematical approaches at the interface between numerical analysis, optimal transport and optimal control to study the dynamics of neural networks for learning and sampling. These theoretical advances will also lead to the design of new architectures with mathematical guarantees. The project also aims, more generally, to develop the use of and interest in AI in the French mathematical analysis community, and to facilitate exchanges on AI topics within this community.
The French mathematical analysis community is one of the strongest and most recognized in the world. It therefore seems important that it should not remain on the sidelines of advances in AI and machine learning. The aim of the project is to ensure that AI techniques become part of the general culture of specialists in numerical analysis and simulation, so that they can apply part of their skills to the development of these techniques. In particular, this will enable the development of safer and more reliable AI systems in all fields (computer vision, natural language processing, robotics, as well as physics, chemistry and biology).
A community of 54 permanent researchers, teacher-researchers and engineers, including around 15 PhD students, 20 post-docs and two contract research engineers.
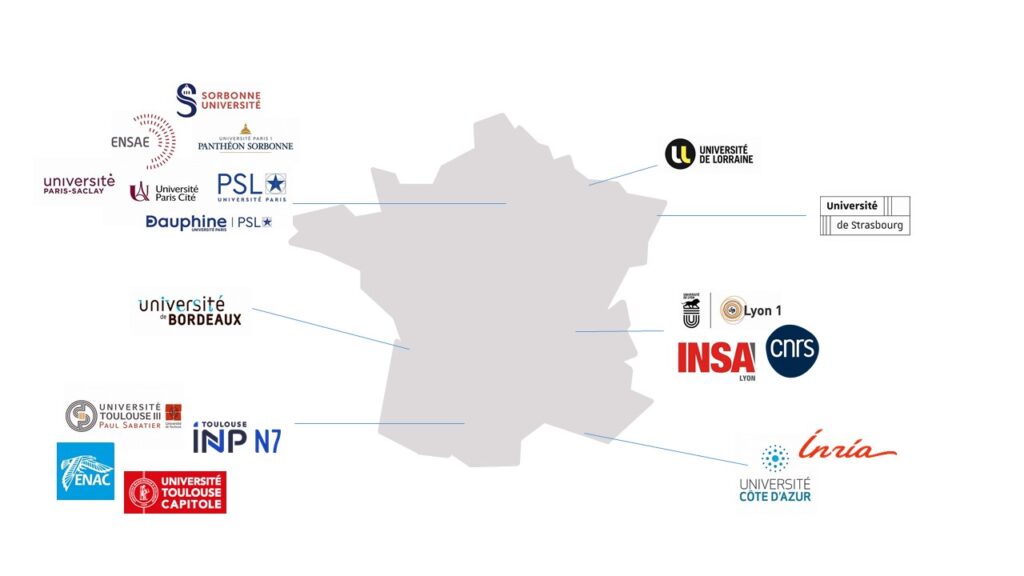
Autres projets
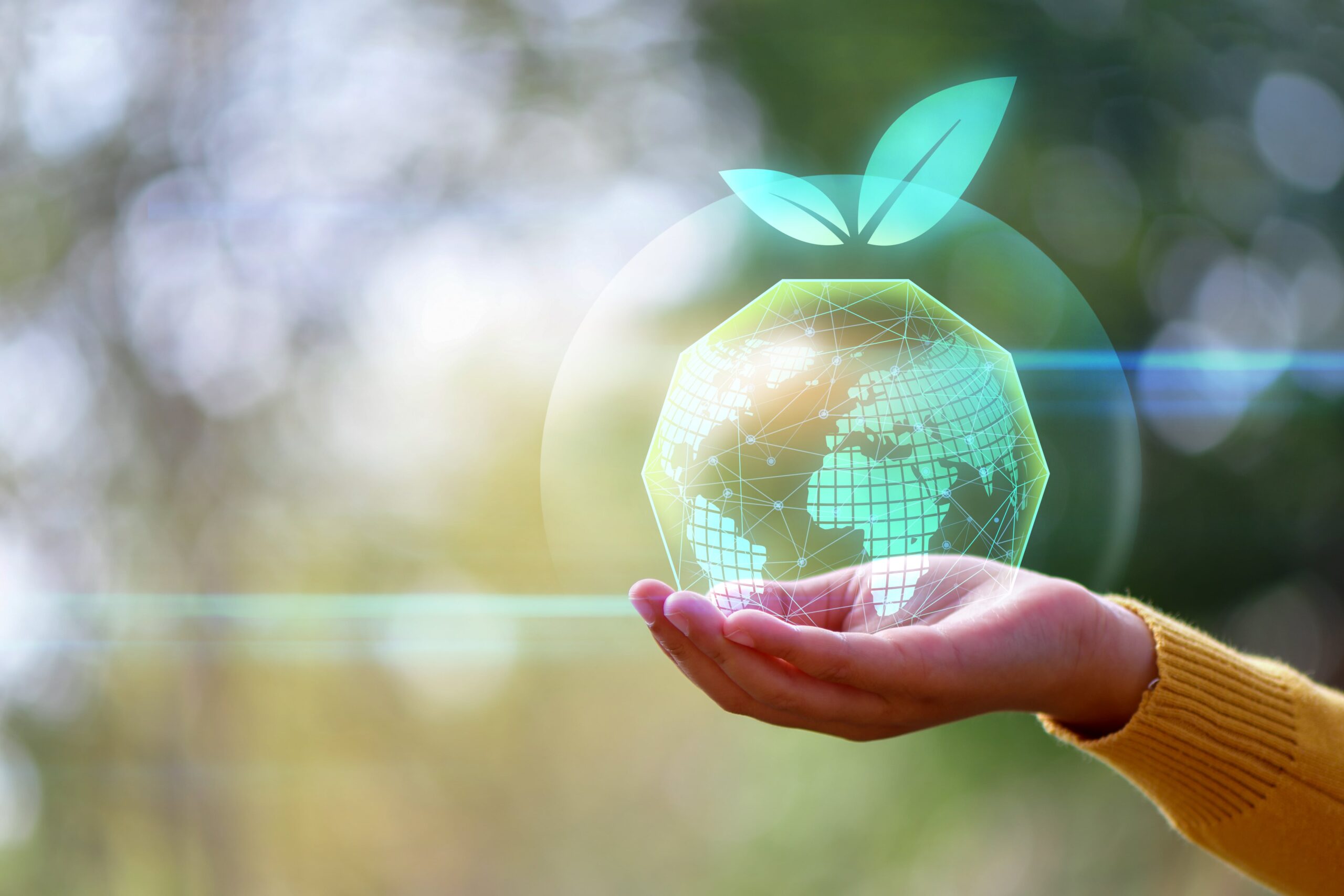
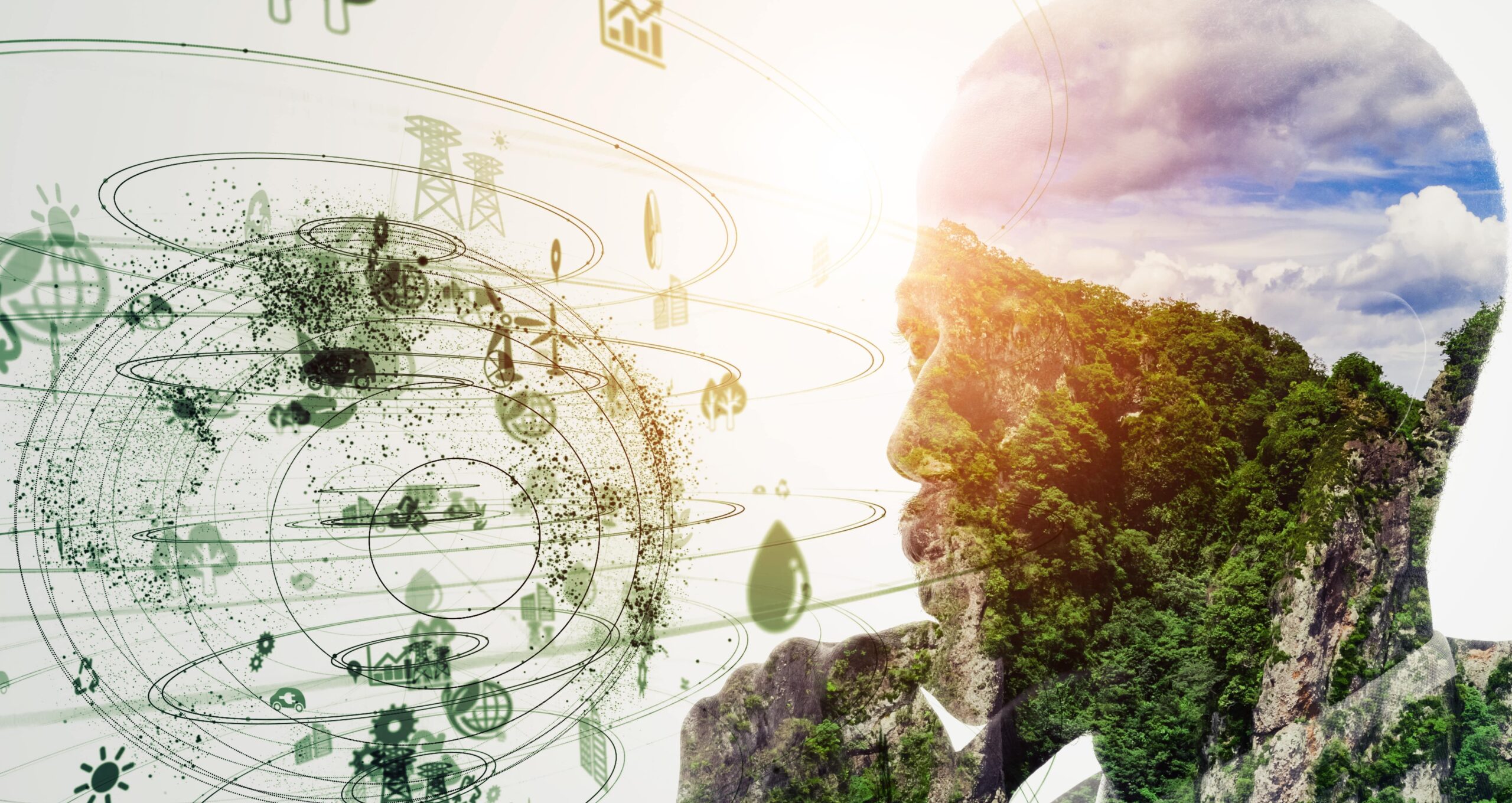
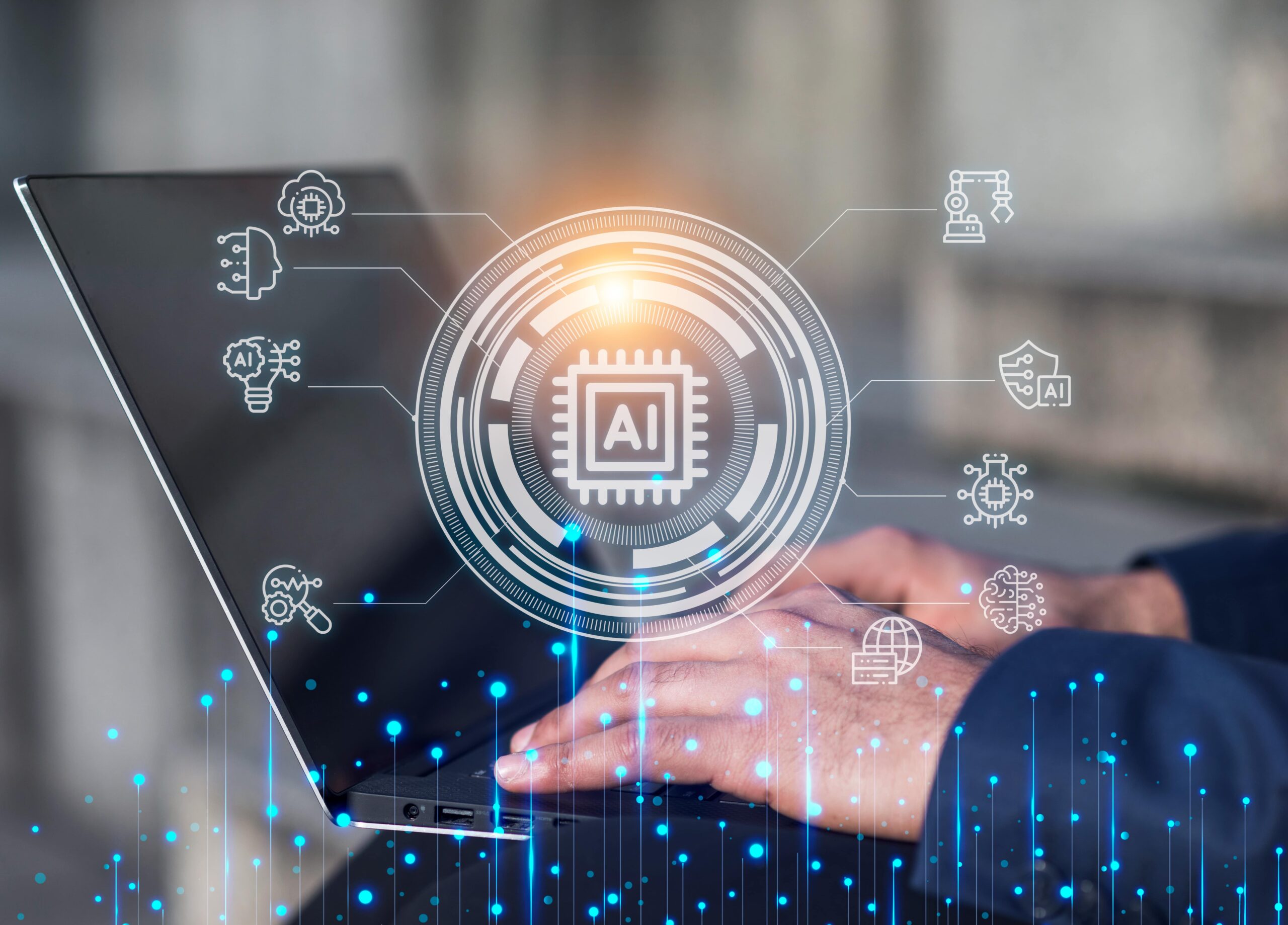
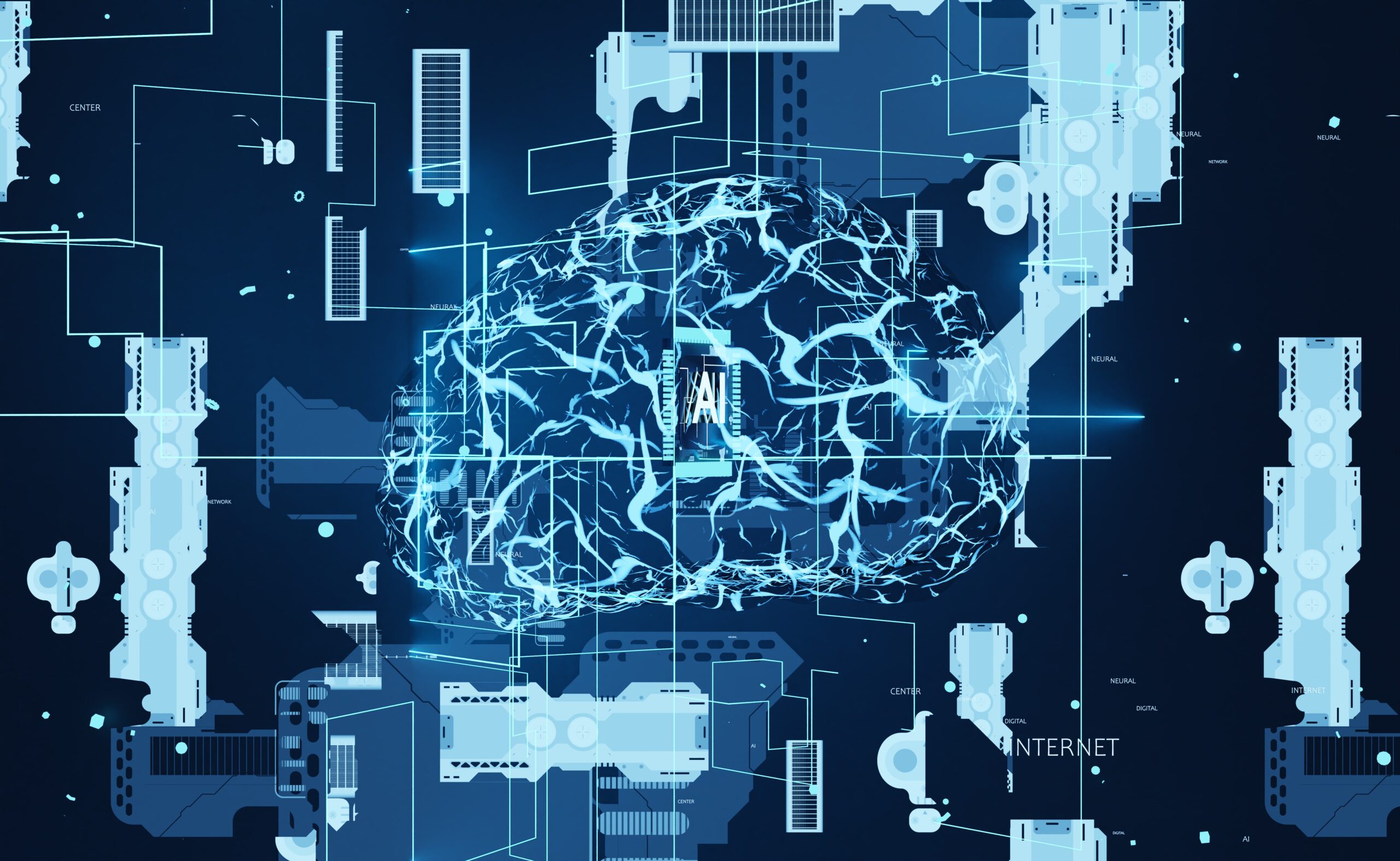
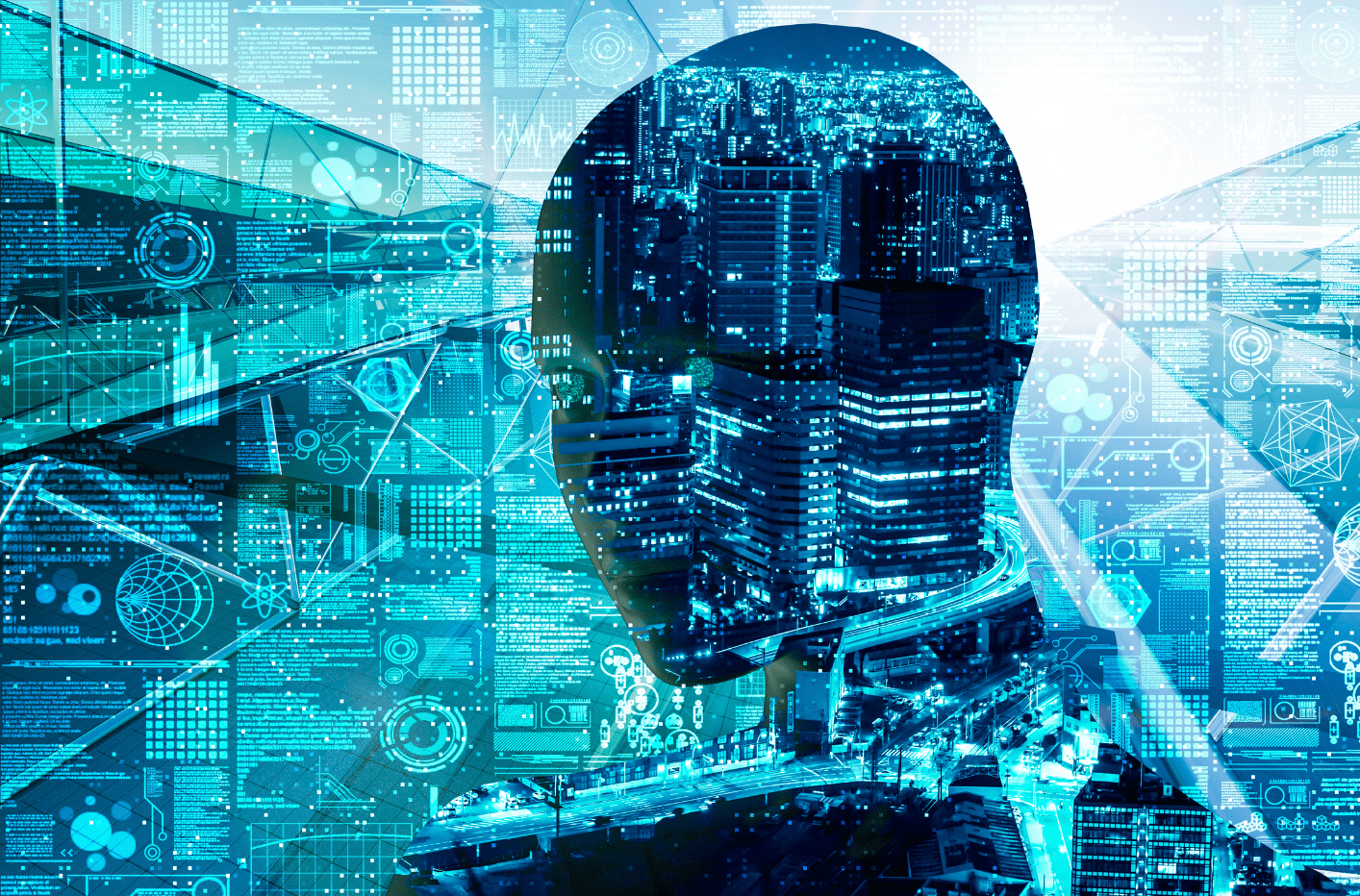
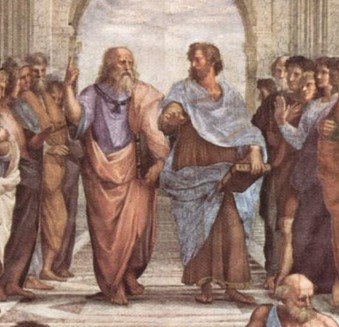
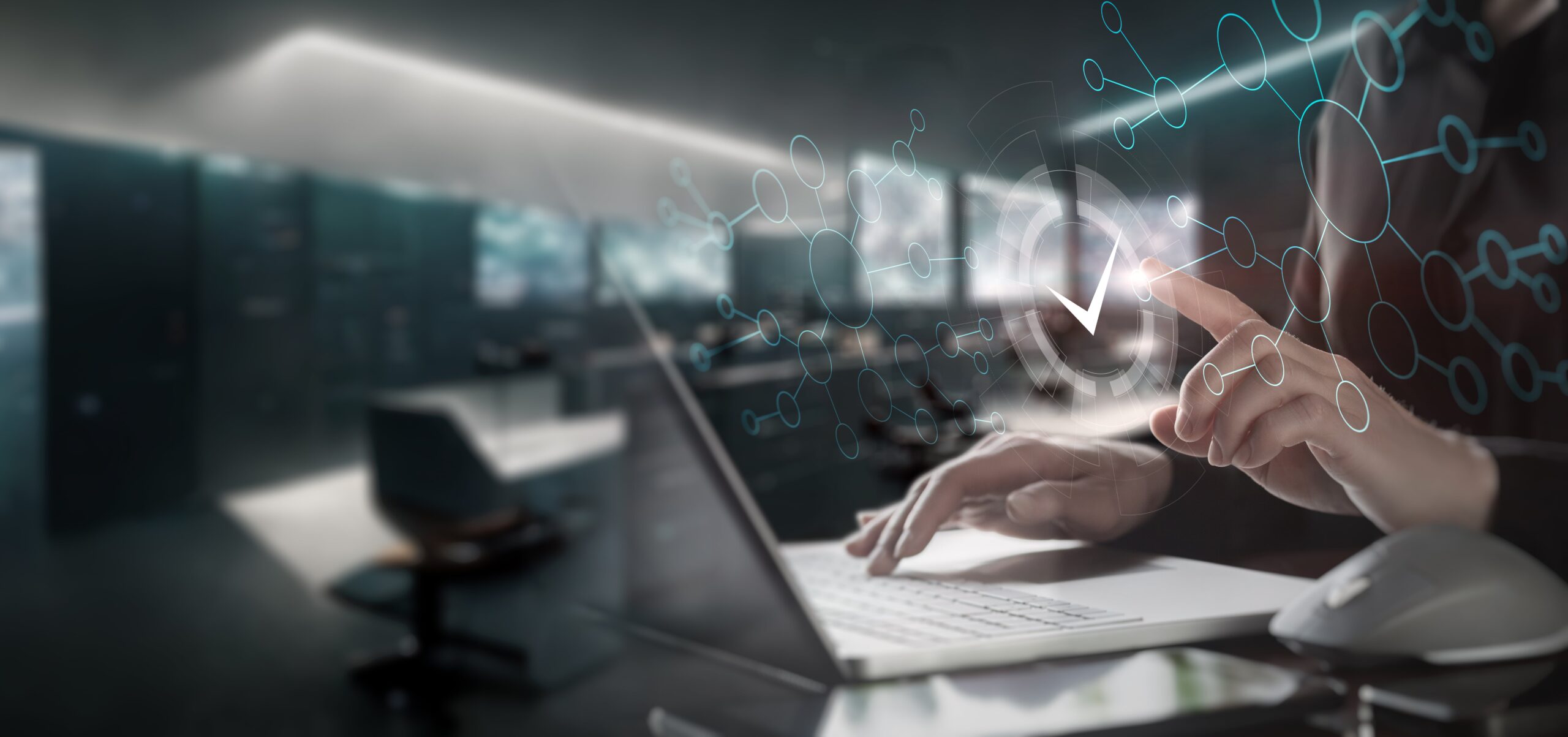
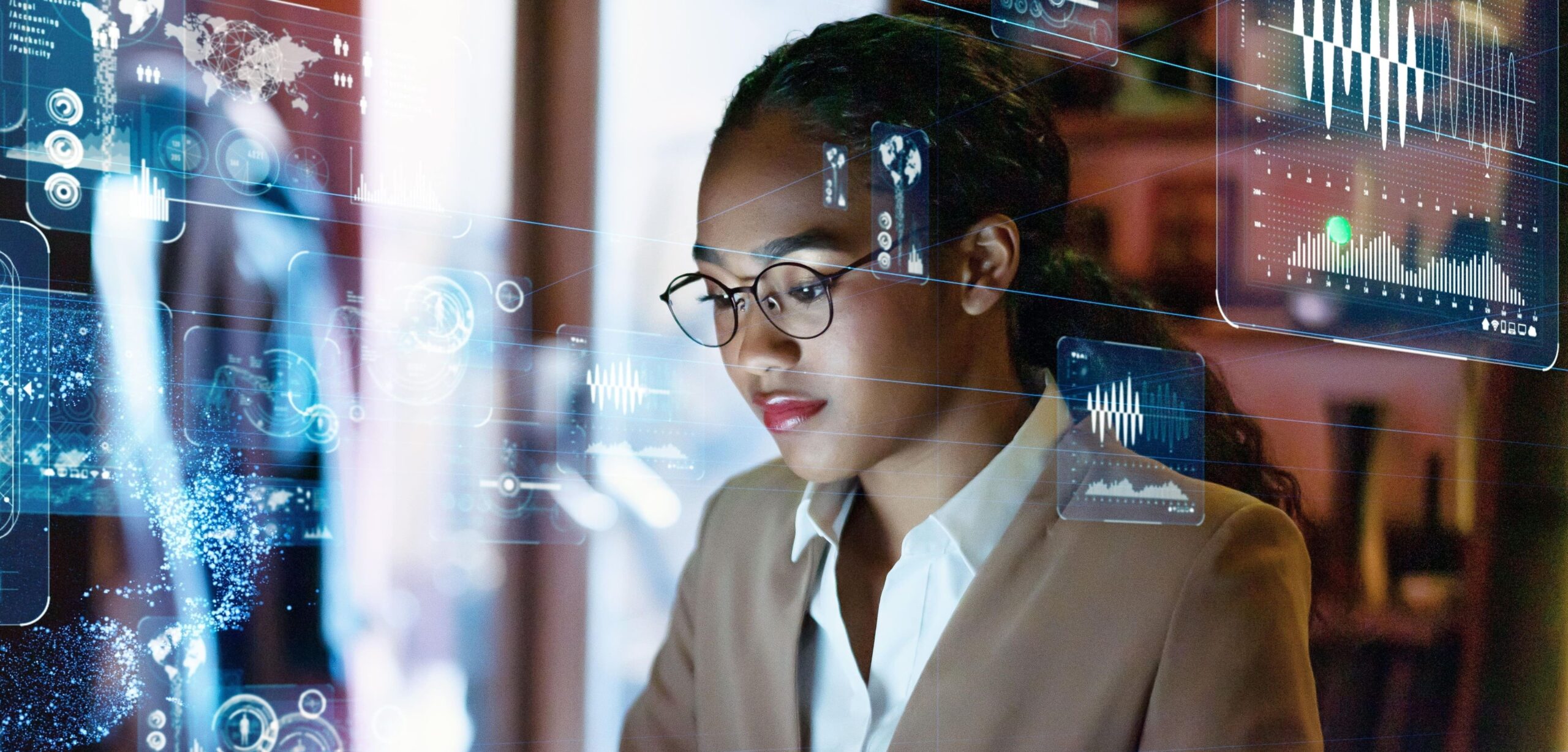