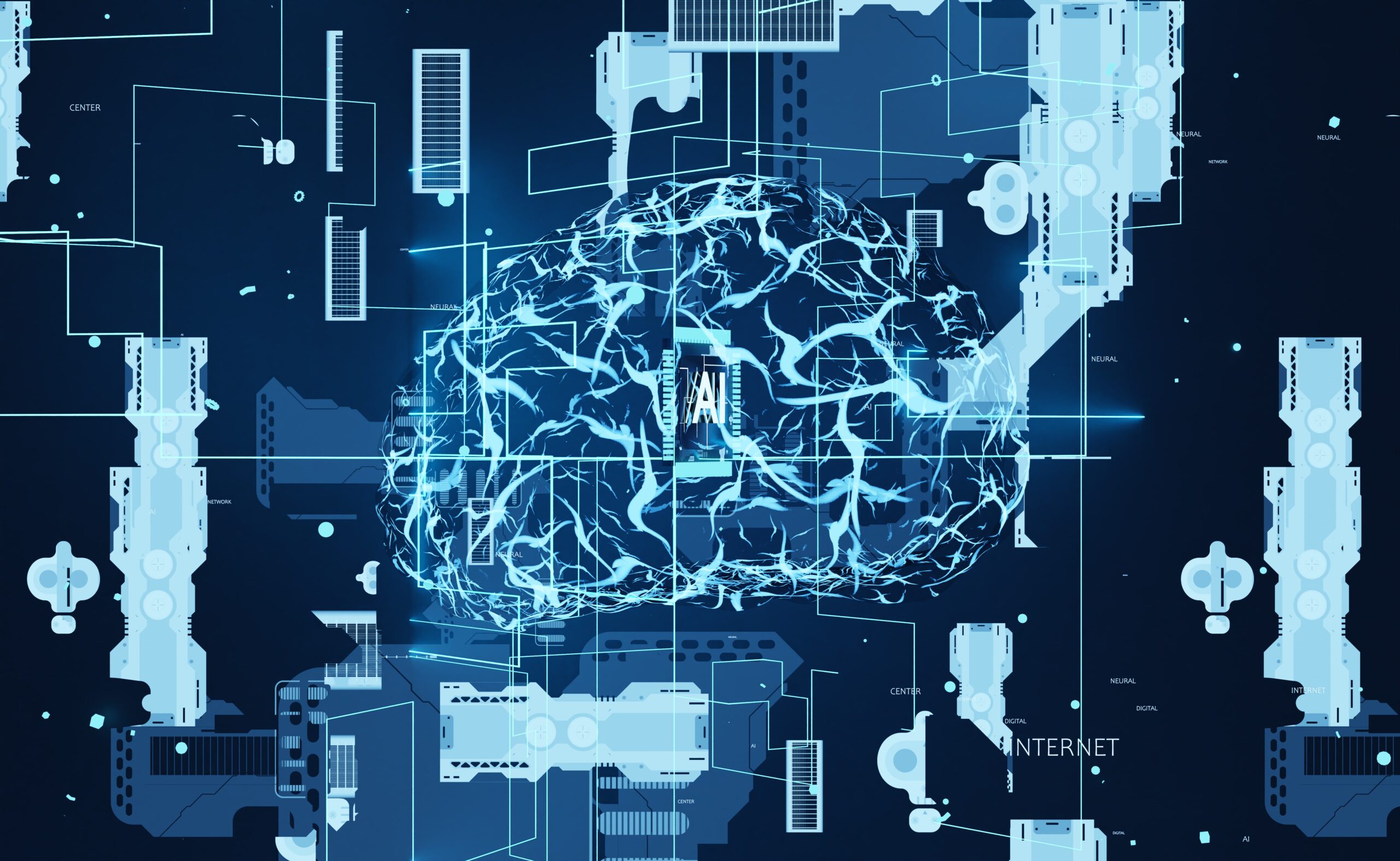
EMERGENCES
Near-physics emerging models for embedded AI
Preview
A breakthrough in emerging physics-based models and the use of different computational models and properties from different physical devices.
Marina Reyboz, Research Director at CEA
Gilles Sassatelli, Research Director at CNRS
The EMERGENCES project aims to advance the state-of-the-art in emerging physics-based models by collaboratively exploring various computational models using the properties of different physical devices. The project will focus on bio-inspired event-driven models, physics-inspired models and innovative physics-based machine learning solutions. Emergences also intends to extend collaborative research activities beyond the consortium’s perimeter, in conjunction with other PEPR projects and beyond other laboratories.
Key words: Emerging AI models, embedded AI, Near-physics AI, energy efficiency, Edge AI
Project web site : Emergences.lirmm.fr
Missions
Our researches
Spiking neural networks and event-based models
Define efficient hardware implementations, the use of fusion with neuromorphic sensors and multimodality:
– demonstrate the growing maturity of SNN with training and design flow for energy-proportional spiking hardware
– exploite the sparsity intrinsic to event-driven sensors that natively output sparse event activity for energy efficiency- investigate the use of multimodality to improve accuracy in link with the neuroscience community
Disruptive Physics-Inspired models
Develop more efficient and accurate training methods for probalistic/Bayesian neural networks by exploring algorithms inspired by the brain and physics.
- Explore Energy Based Models implementation opportunities at the technological level exploiting analog and emerging memory technologies.
- Investigate brain – and physics- inspired algorithms for such models
Near-physics design for machine learning
Improve the energy efficiency of deep learning models for inference and learning.
Two aspects will be taken into account:
- the hardware/software co-optimization of emerging algorithms : such as attentional layers or incremental learning
- the hardware architecture investigations for leveraging the benefits of emerging technologies.
Consortium
CEA, CNRS, Université Côte d’Azur, Université Aix-Marseille, Université de Bordeaux, Université de Lille, Institut National Polytechnique de Bordeaux, Ecole Centrale Lyon
- Advancing state-of-the-art in Spiking Neural network in multimodality, sensor fusion, hardware implementation, and training
- Advances in physics-inspired models (probabilistic models, Energy Based Models etc) and training algorithms exploiting physical devices properties
- Novels approaches and algorithms for edge deep-learning hardware (on-chip learning, IMC etc.) and software exploiting NVM properties
EMERGENCES aims to develop very low-power embedded AI models for responsible and virtuous applications at the edge such as environmental observation, health,… Emergences will therefore be attentive to the sustainability issues of the models developed, in particular by defining indicators to measure these aspects
Because of the unavoidable societal and philosophical implications of AI as a whole, Emergences will concurrently to the research activities run a track aimed at analyzing and anticipating the impact of its contributions.
A community of 60 researchers, teaching researchers and permanents engineers mobilizing 19 doctoral students, 12 post-doctoral students and 4 contractual researcher engineers as the project progresses.
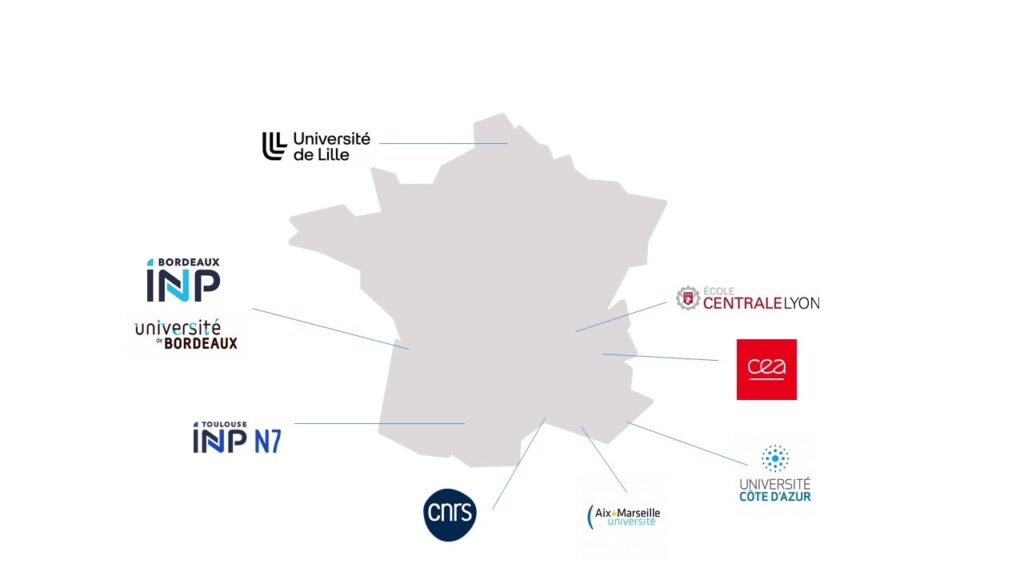
Publication
Autres projets
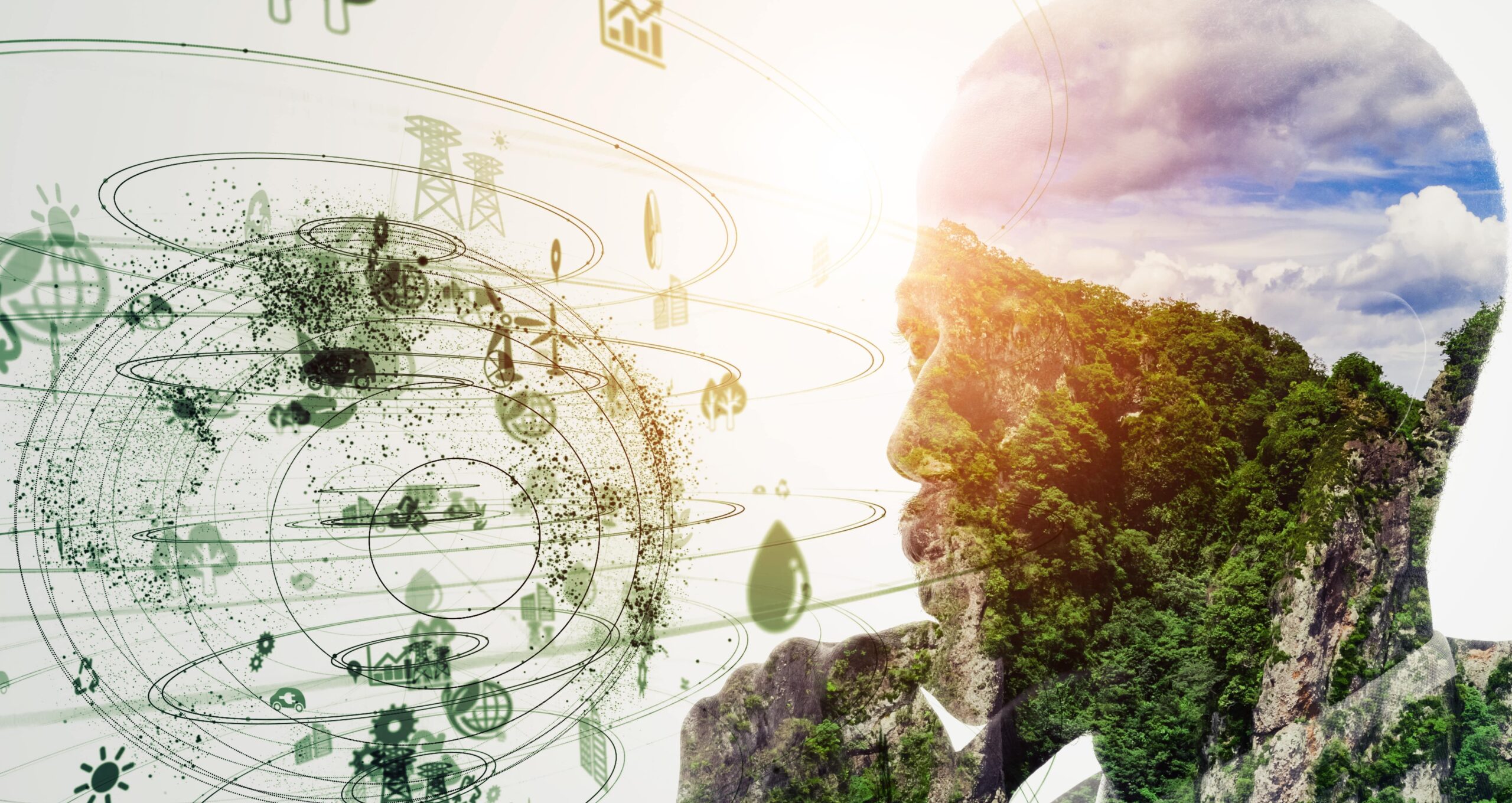
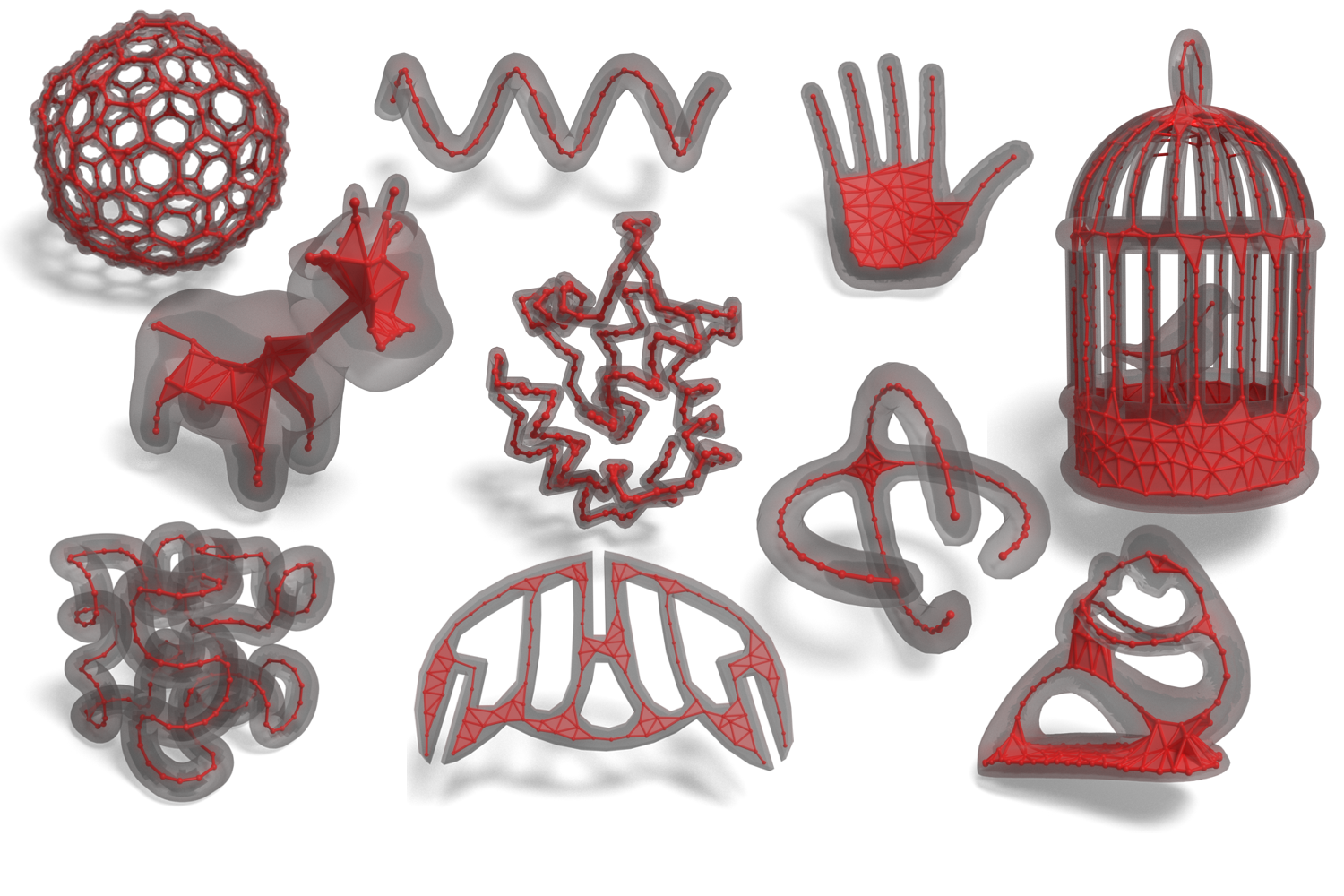
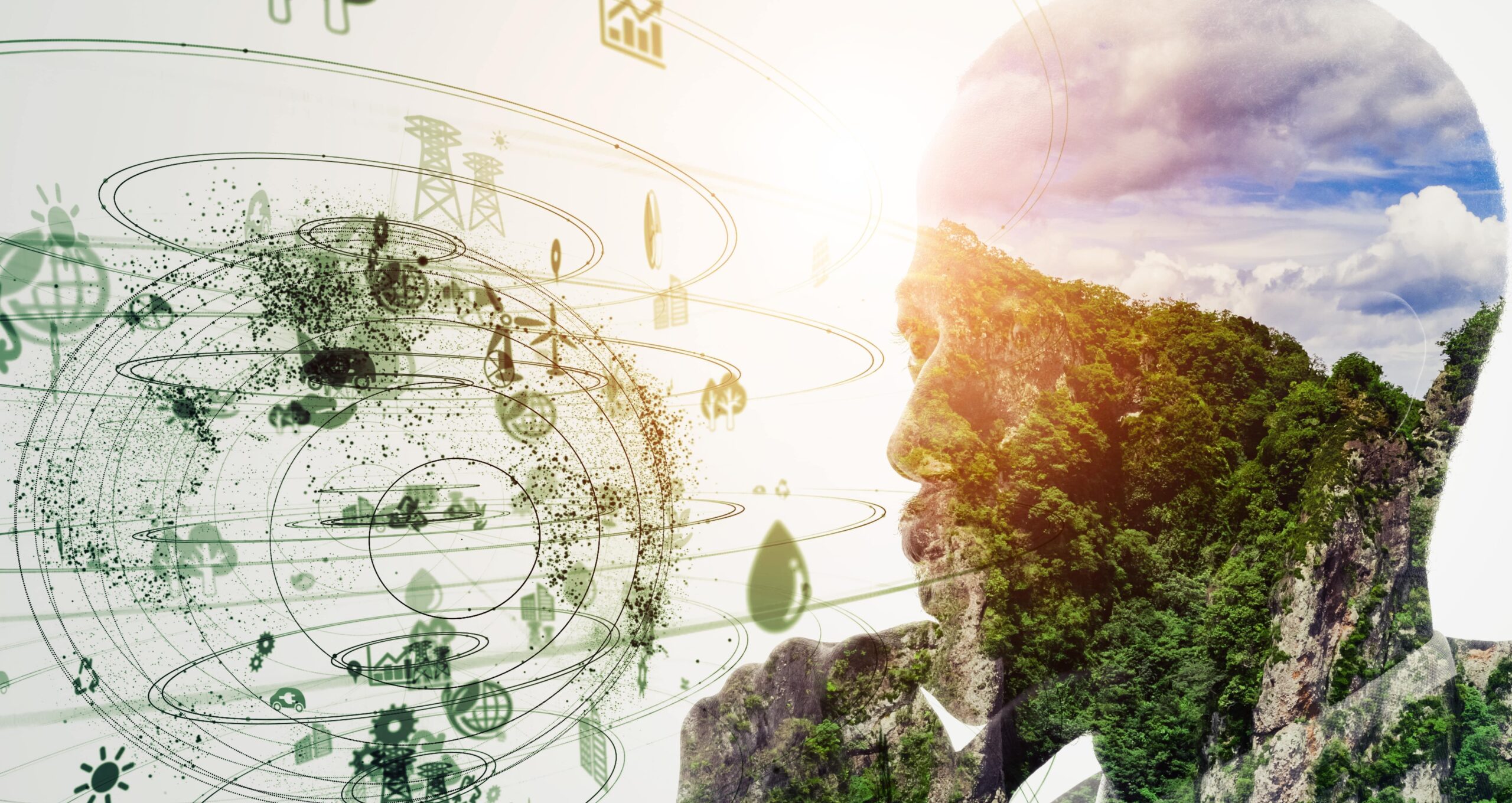
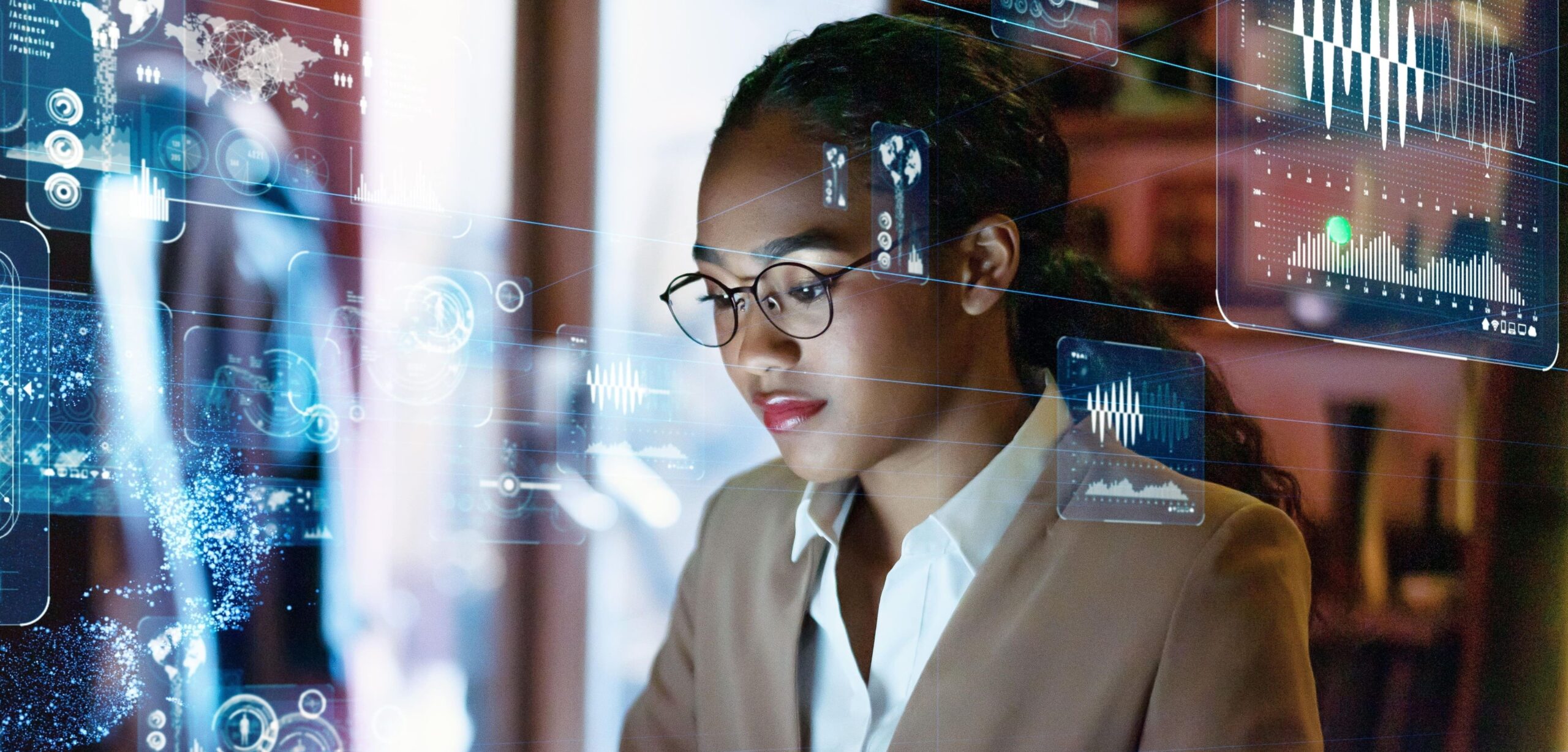
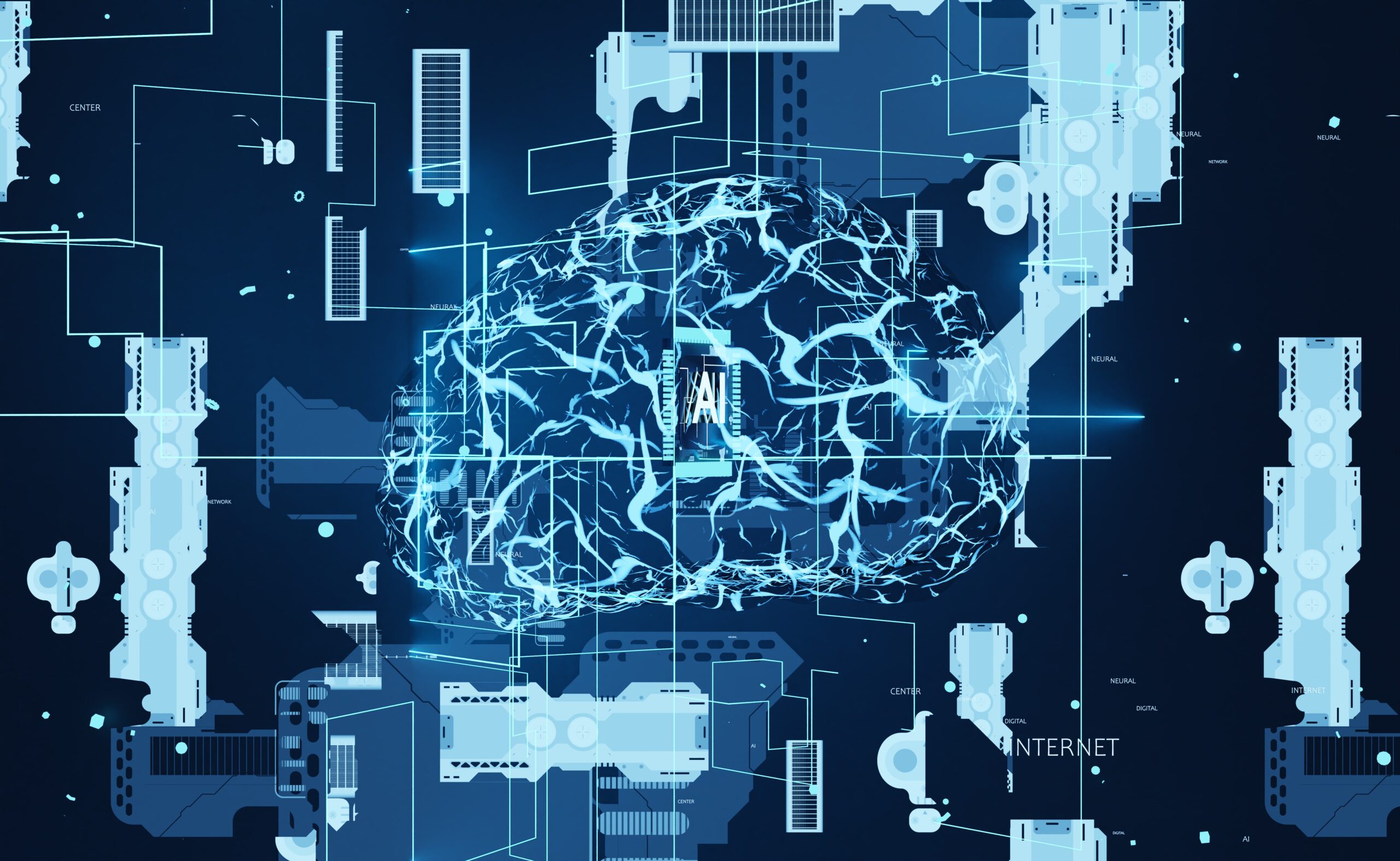
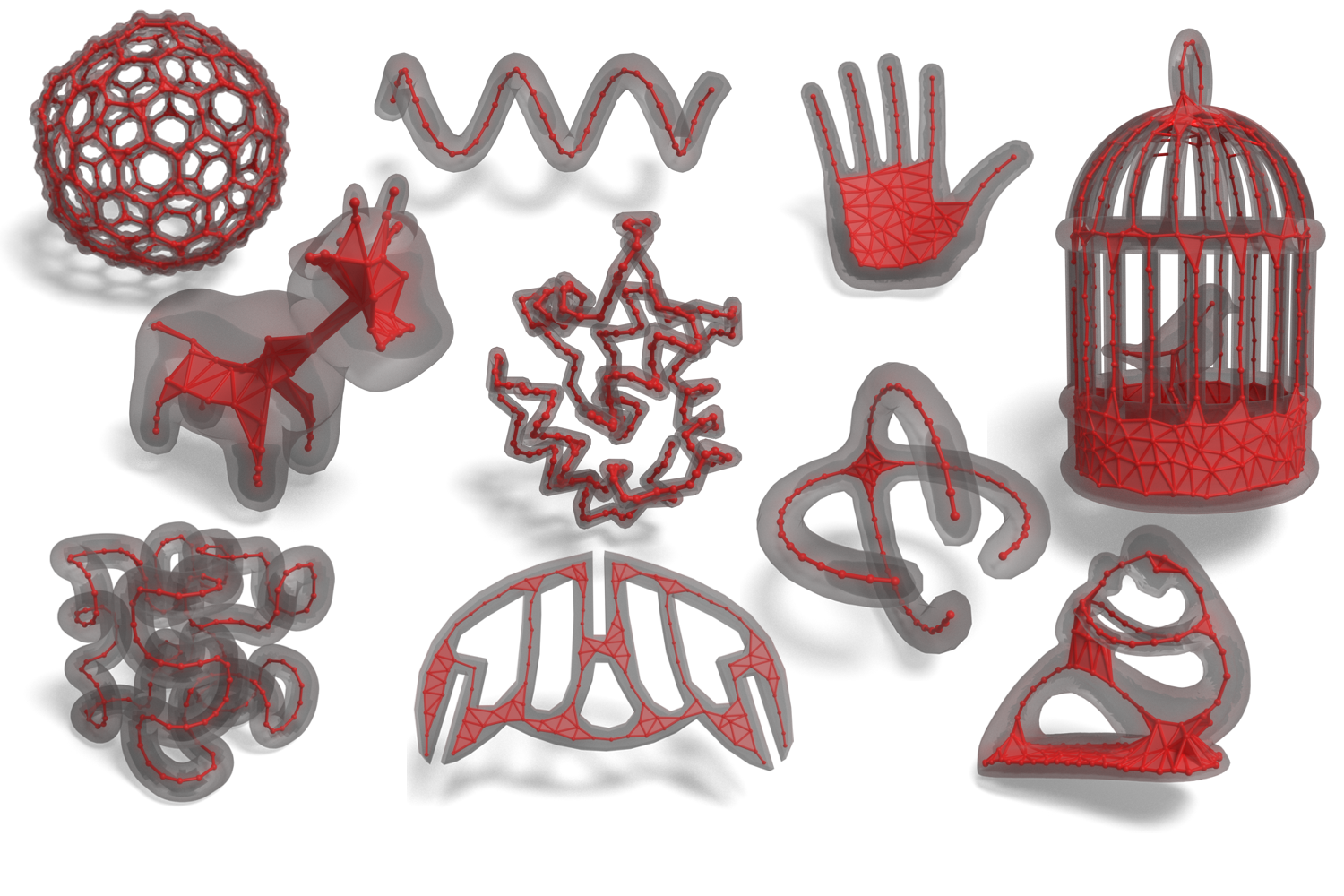
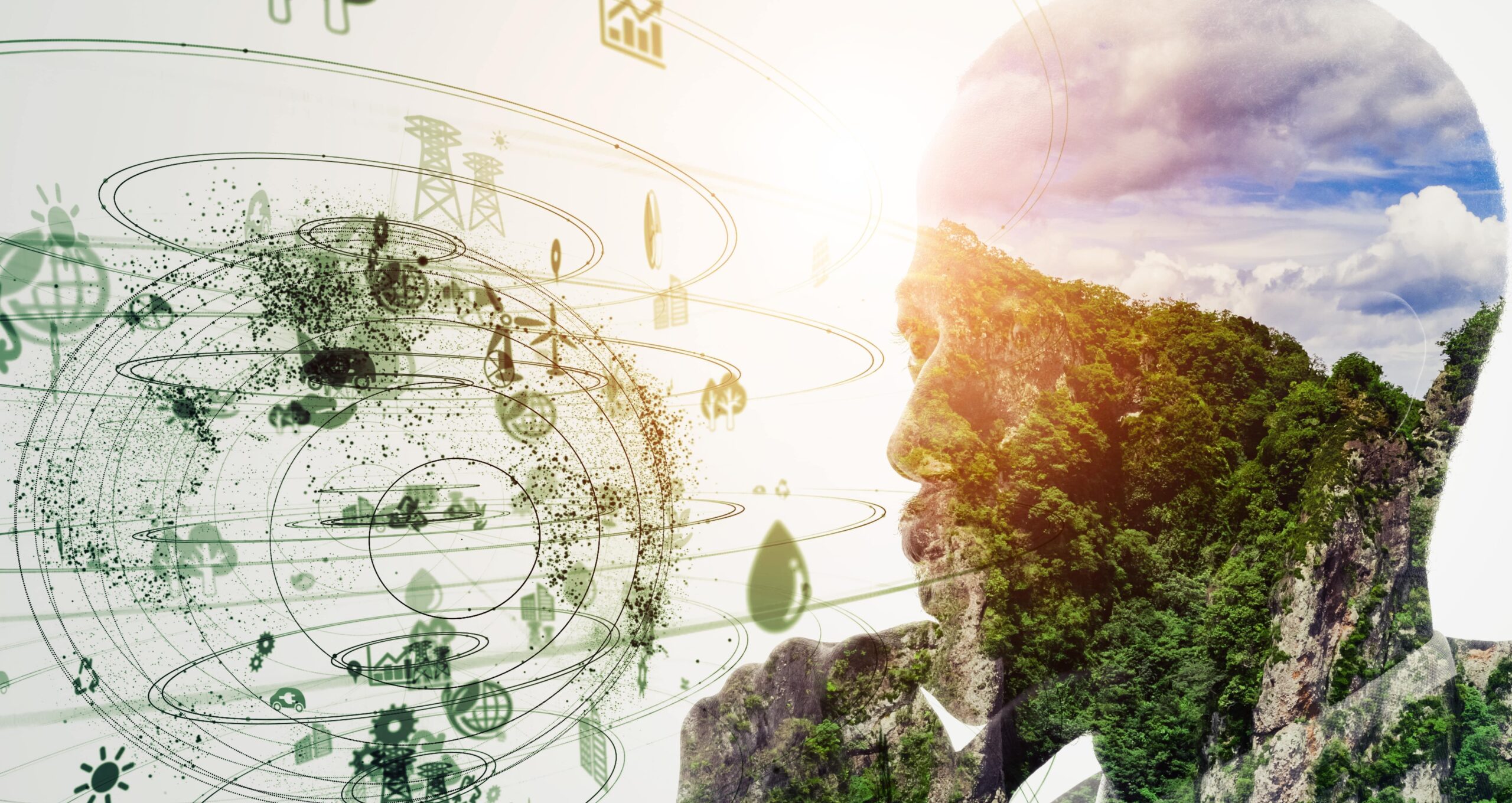
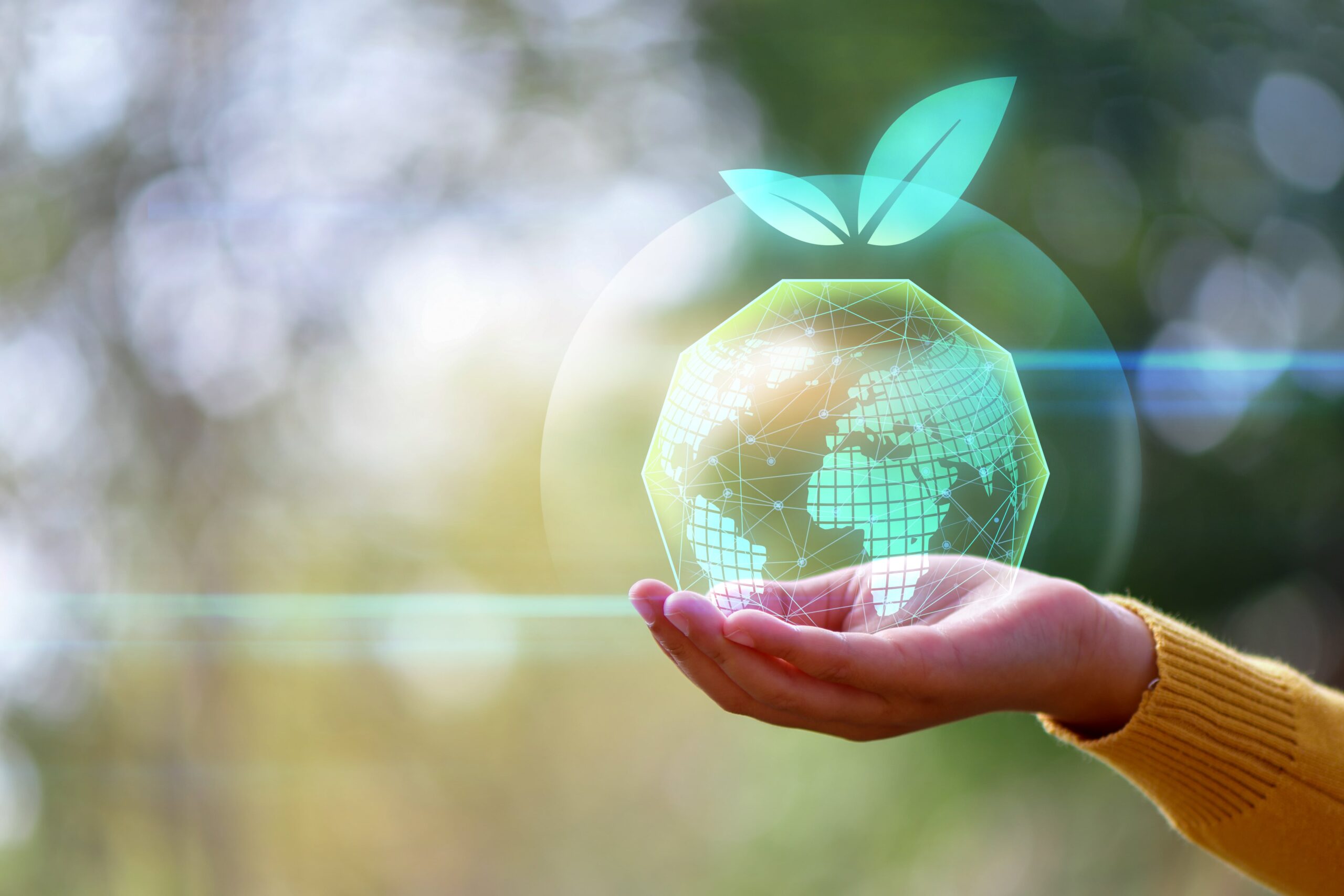
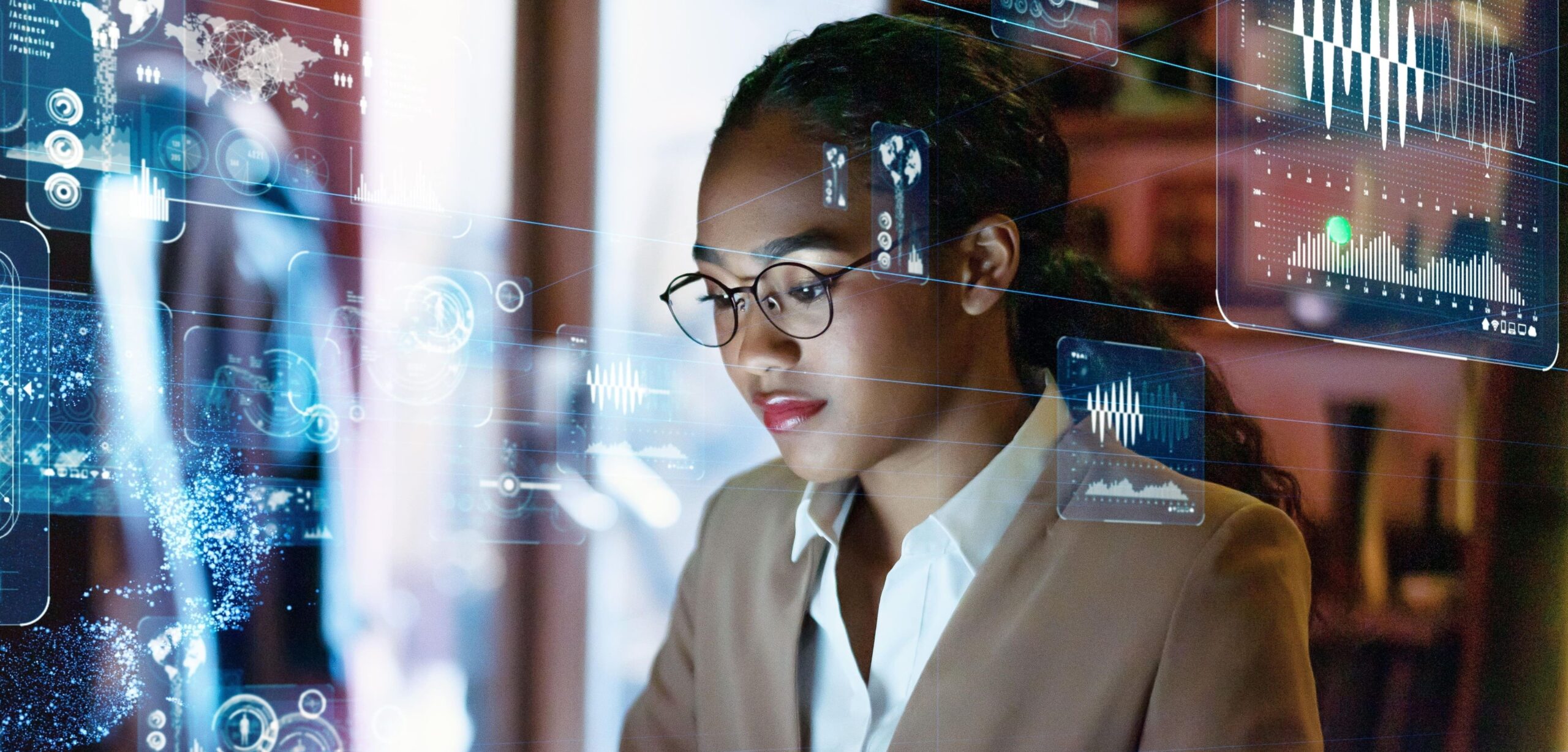
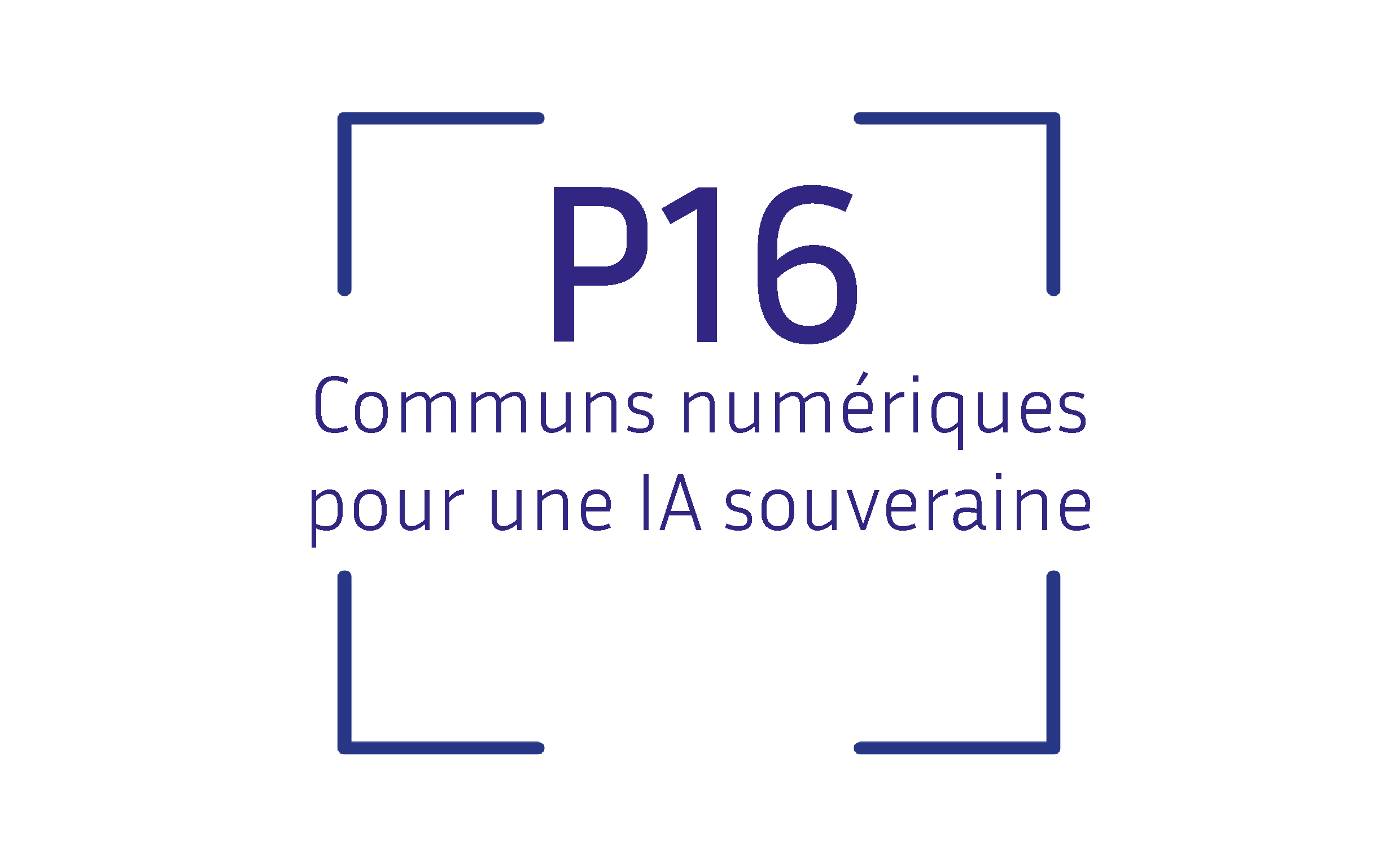
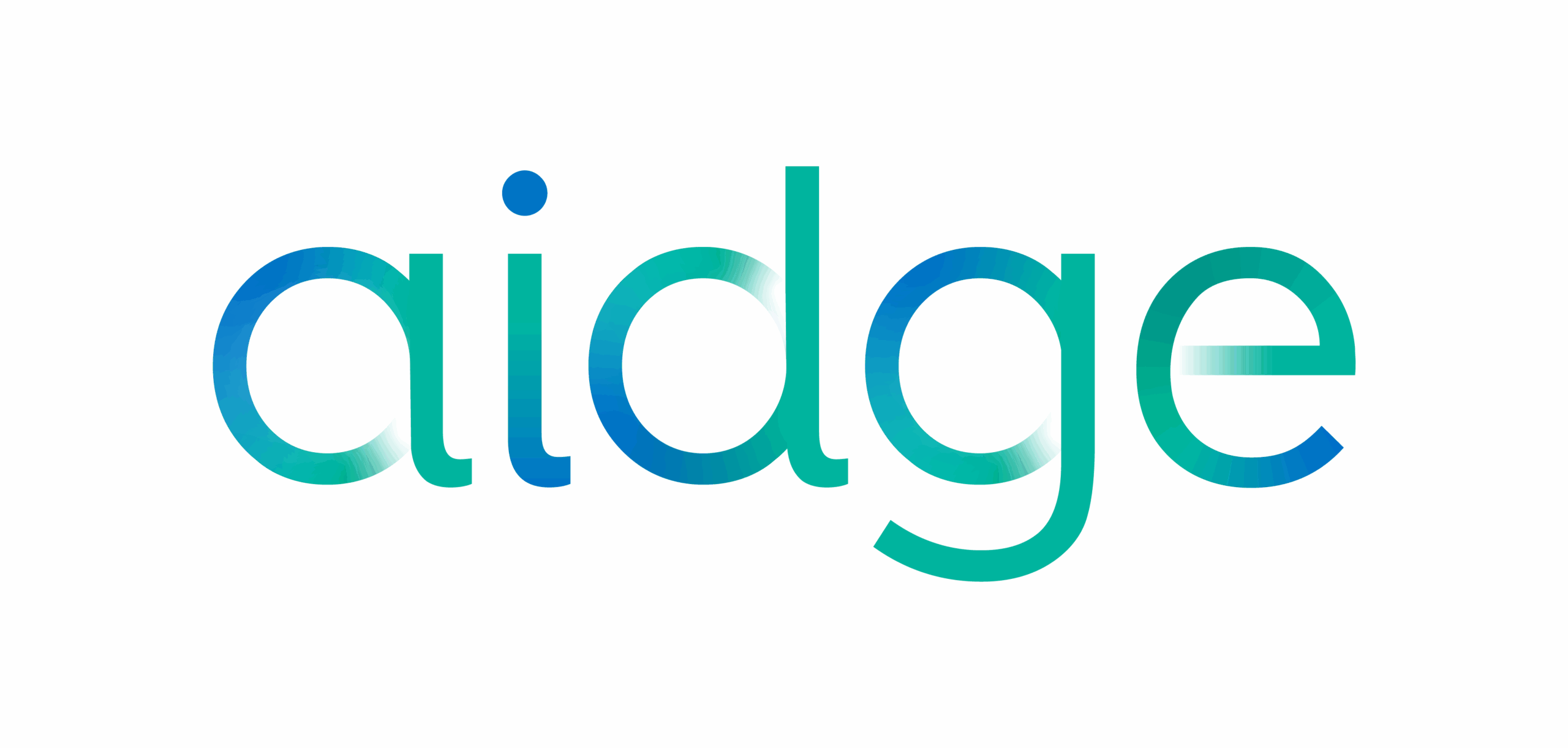
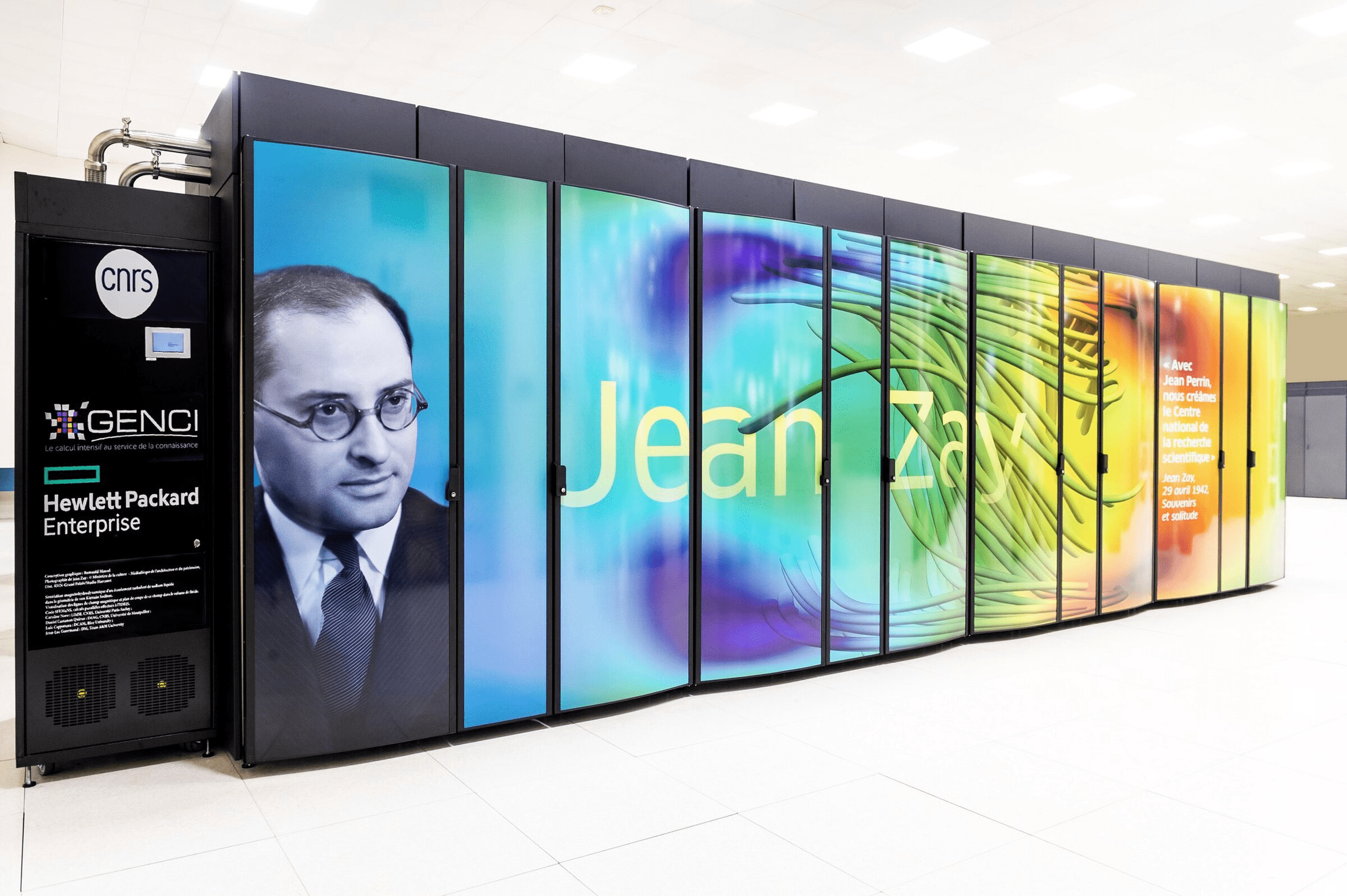
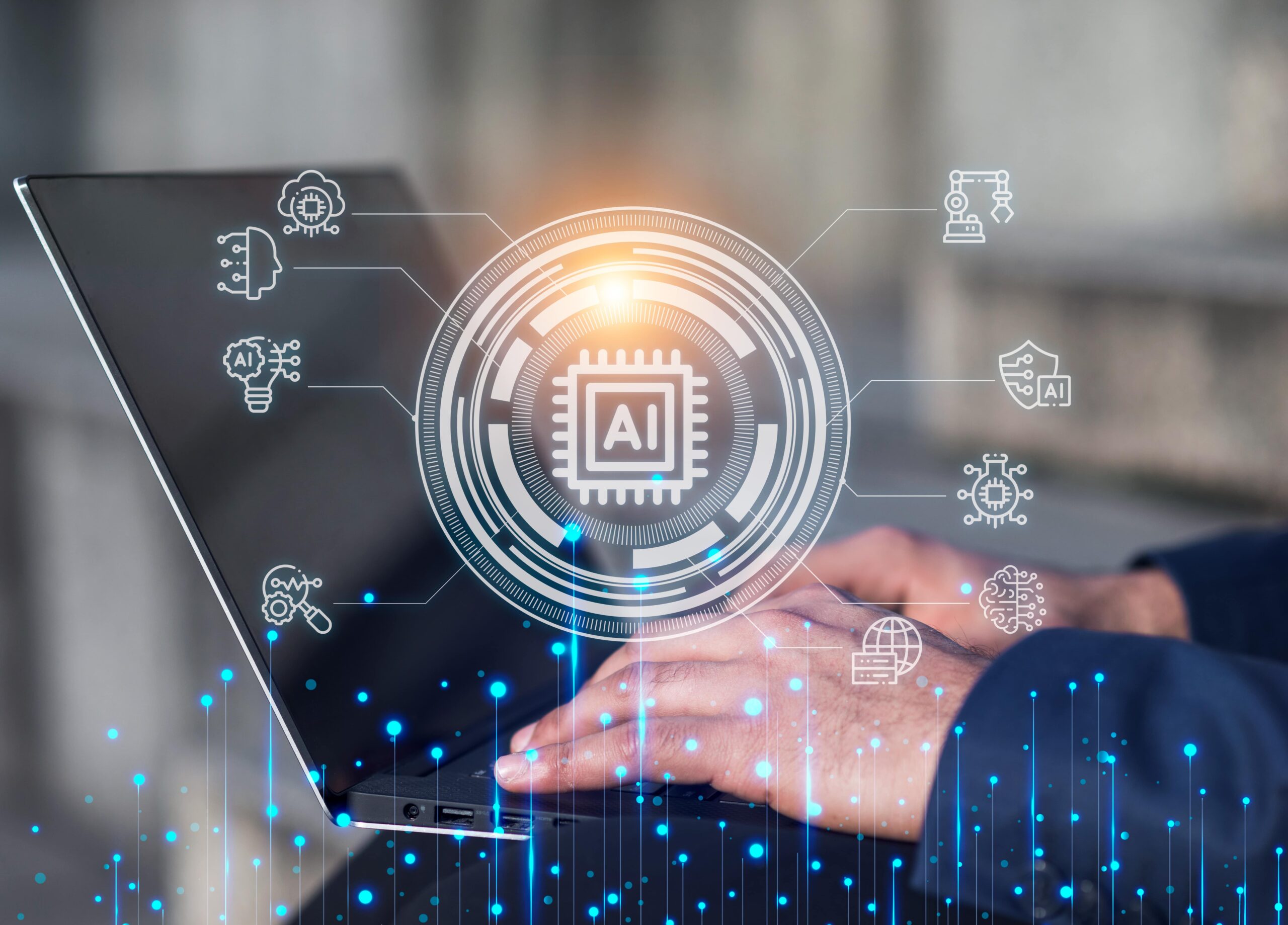
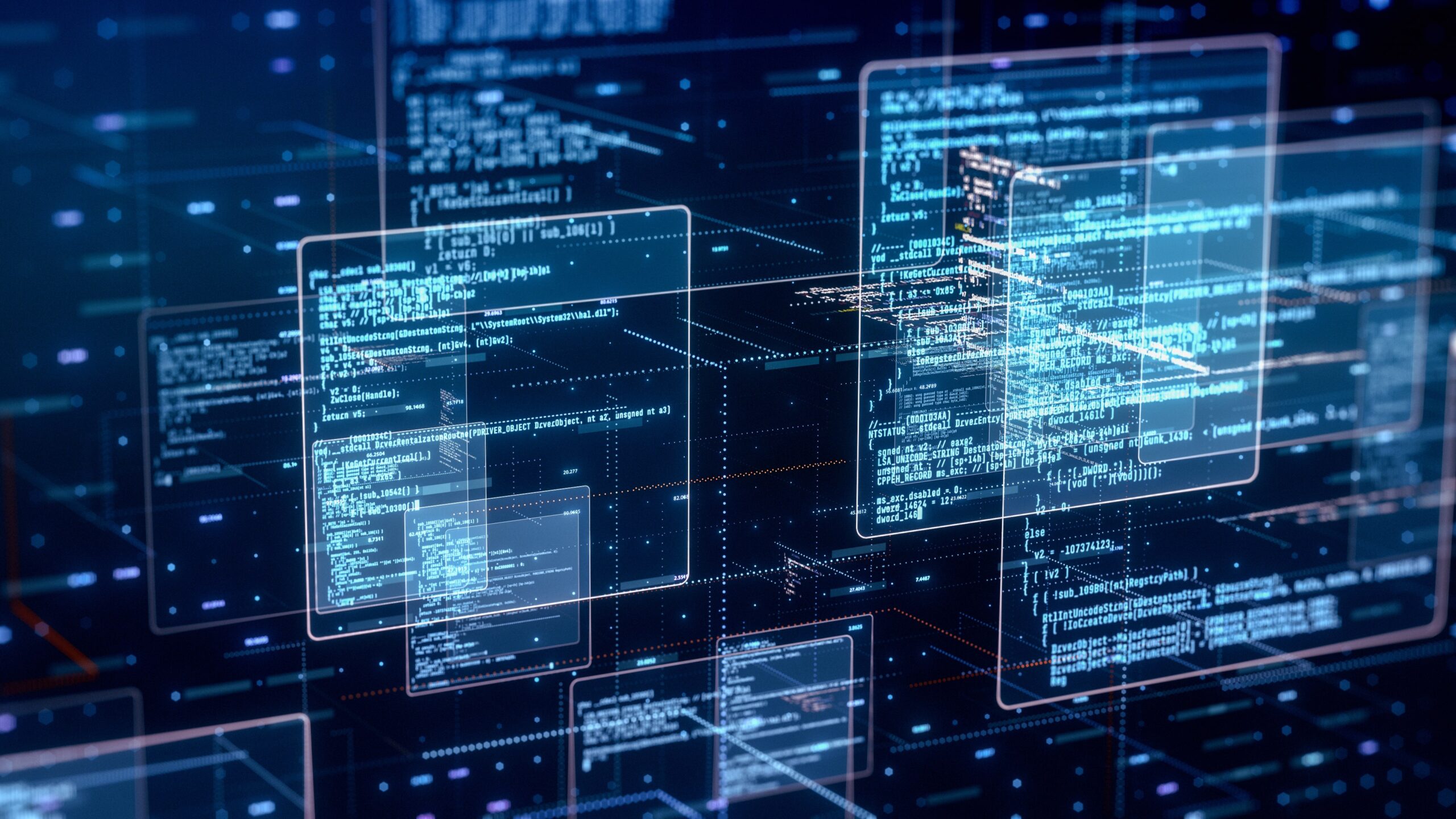
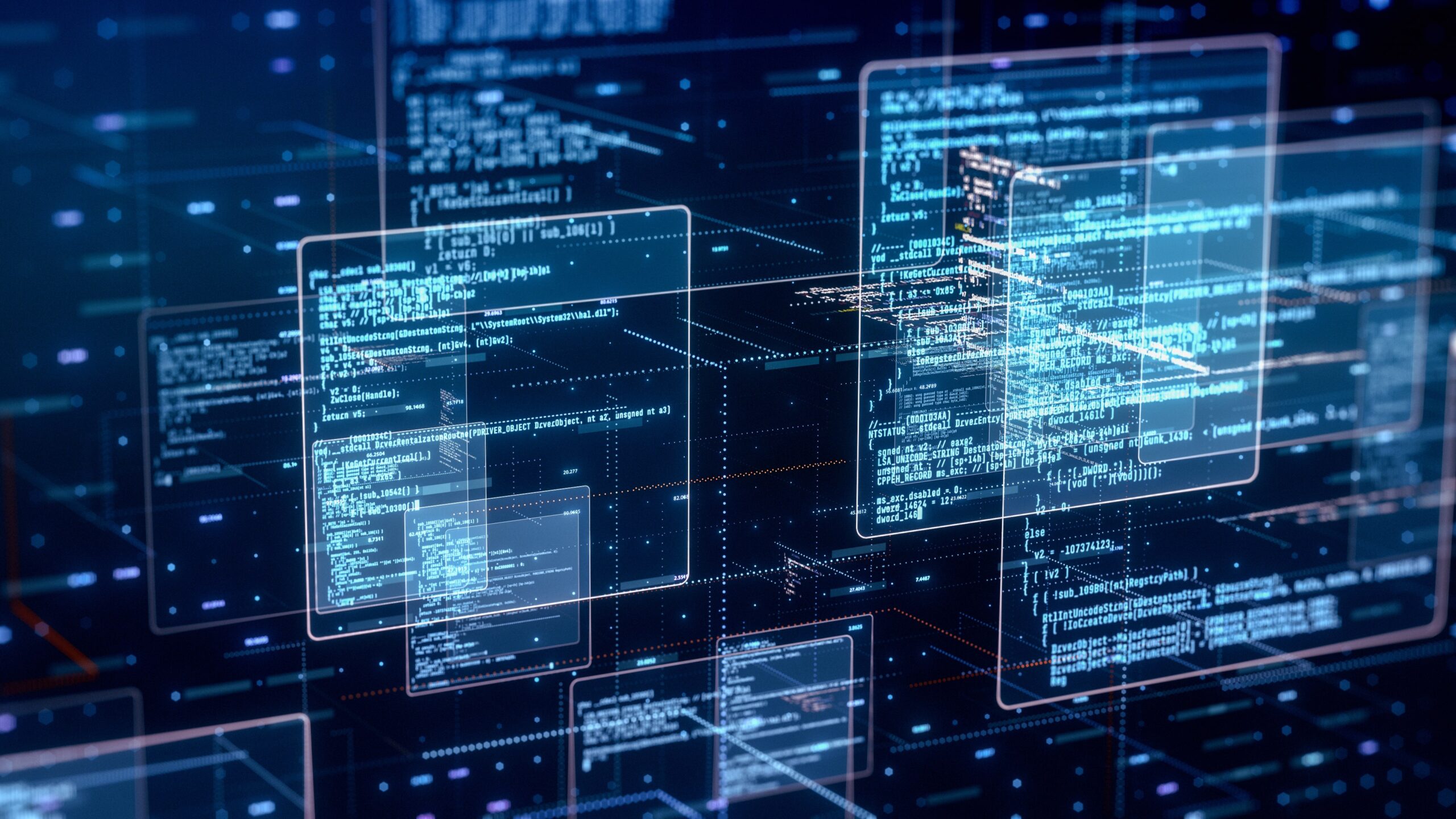
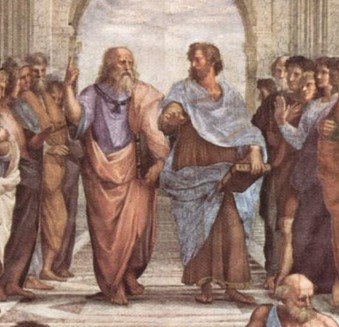
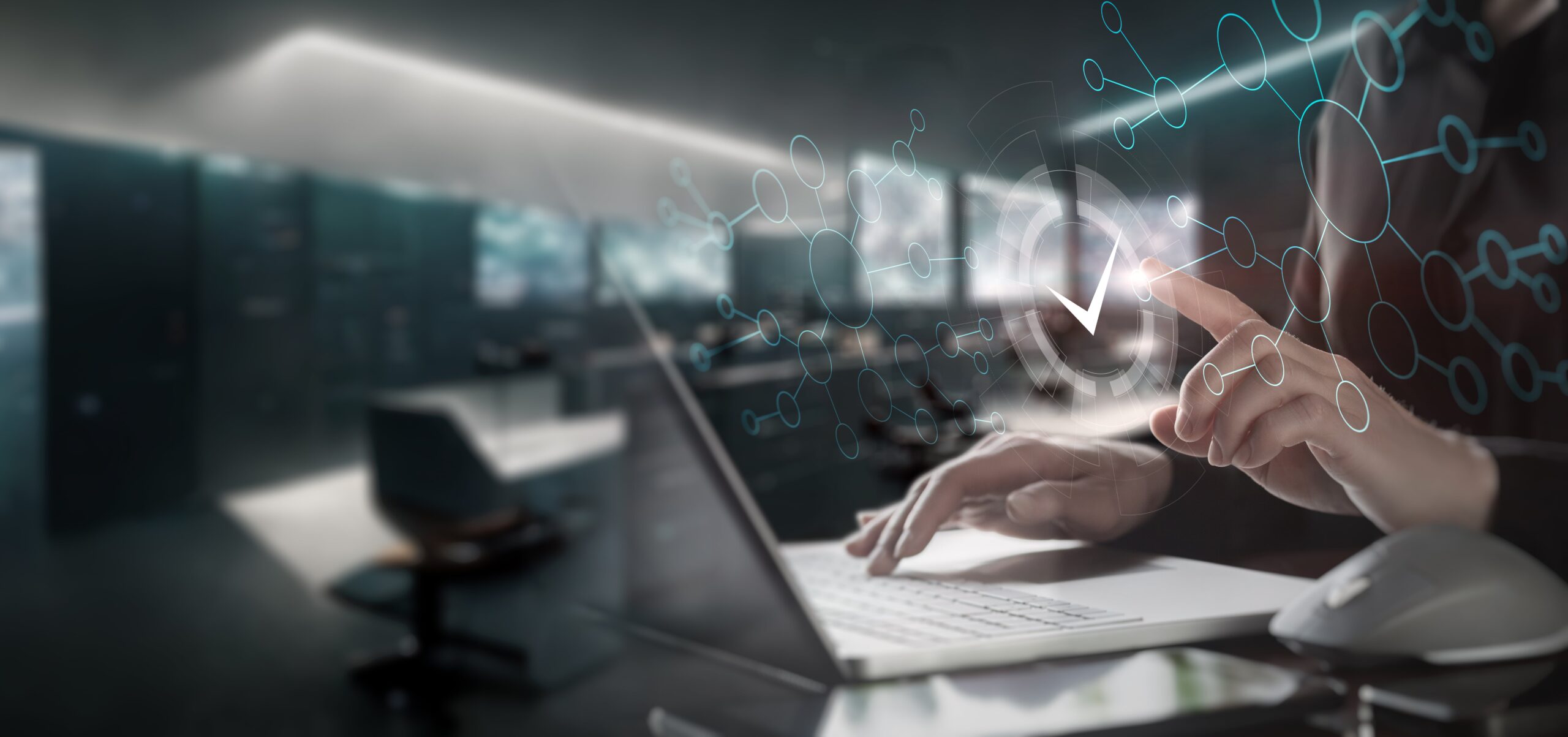
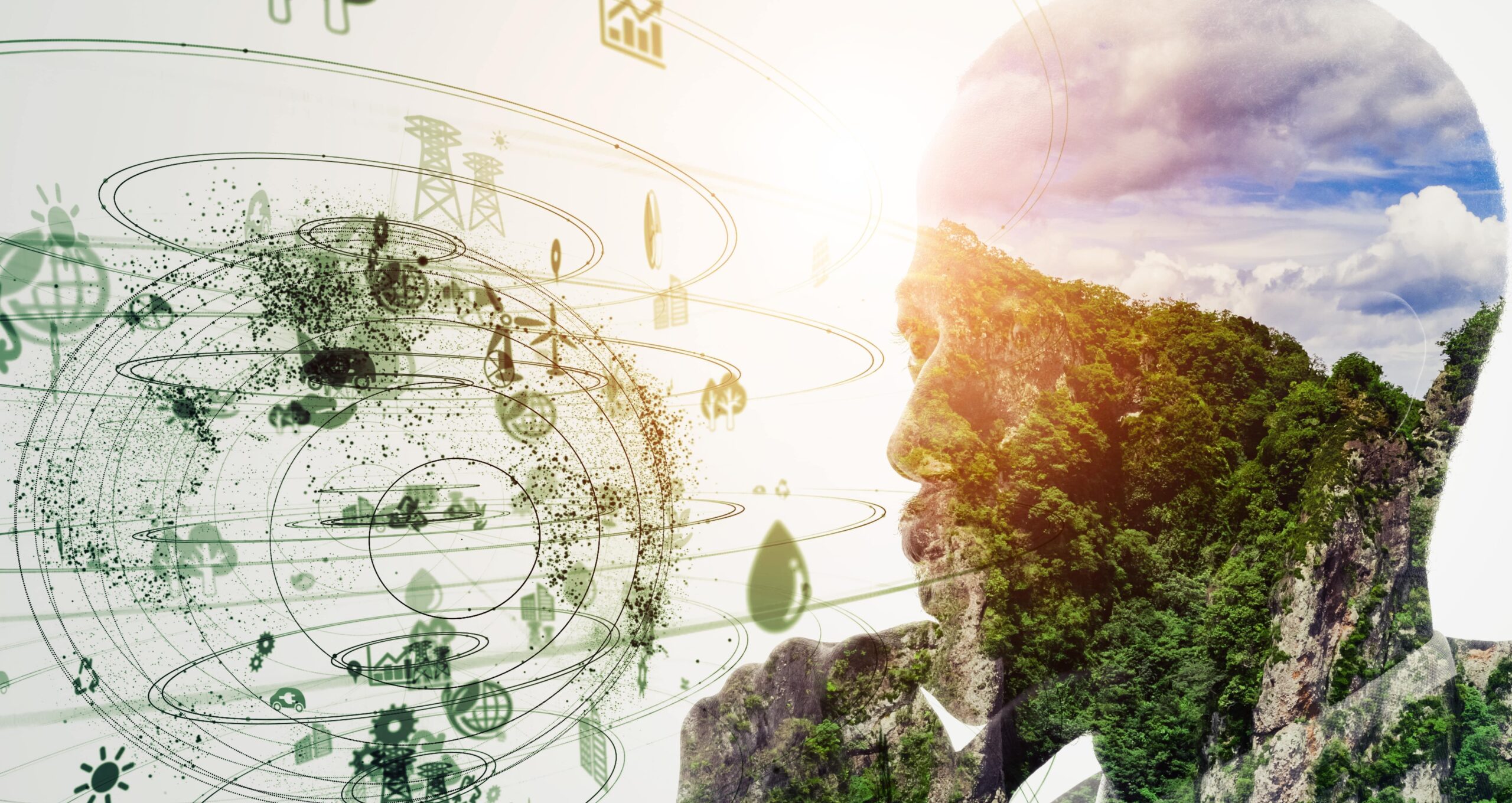
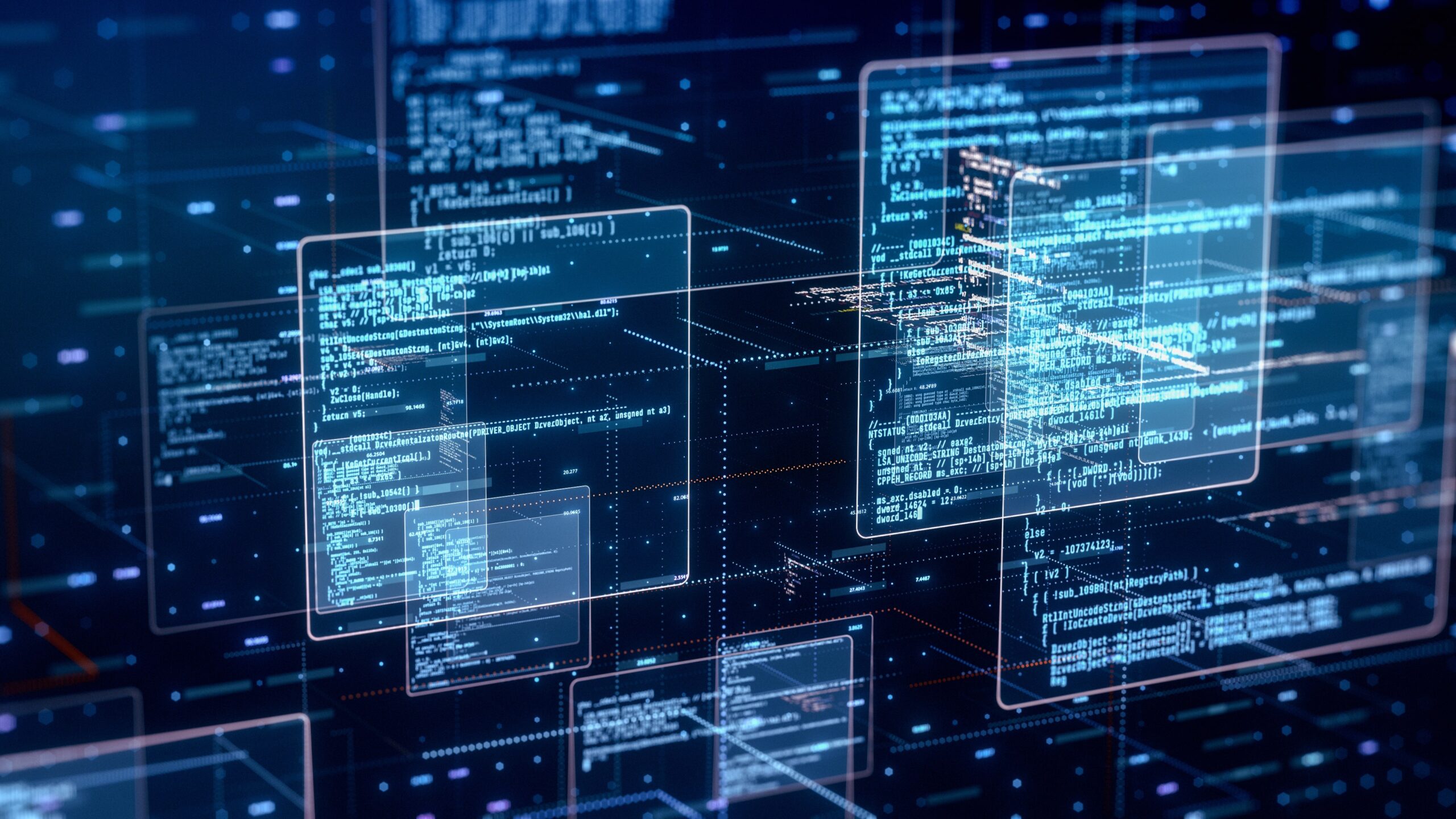
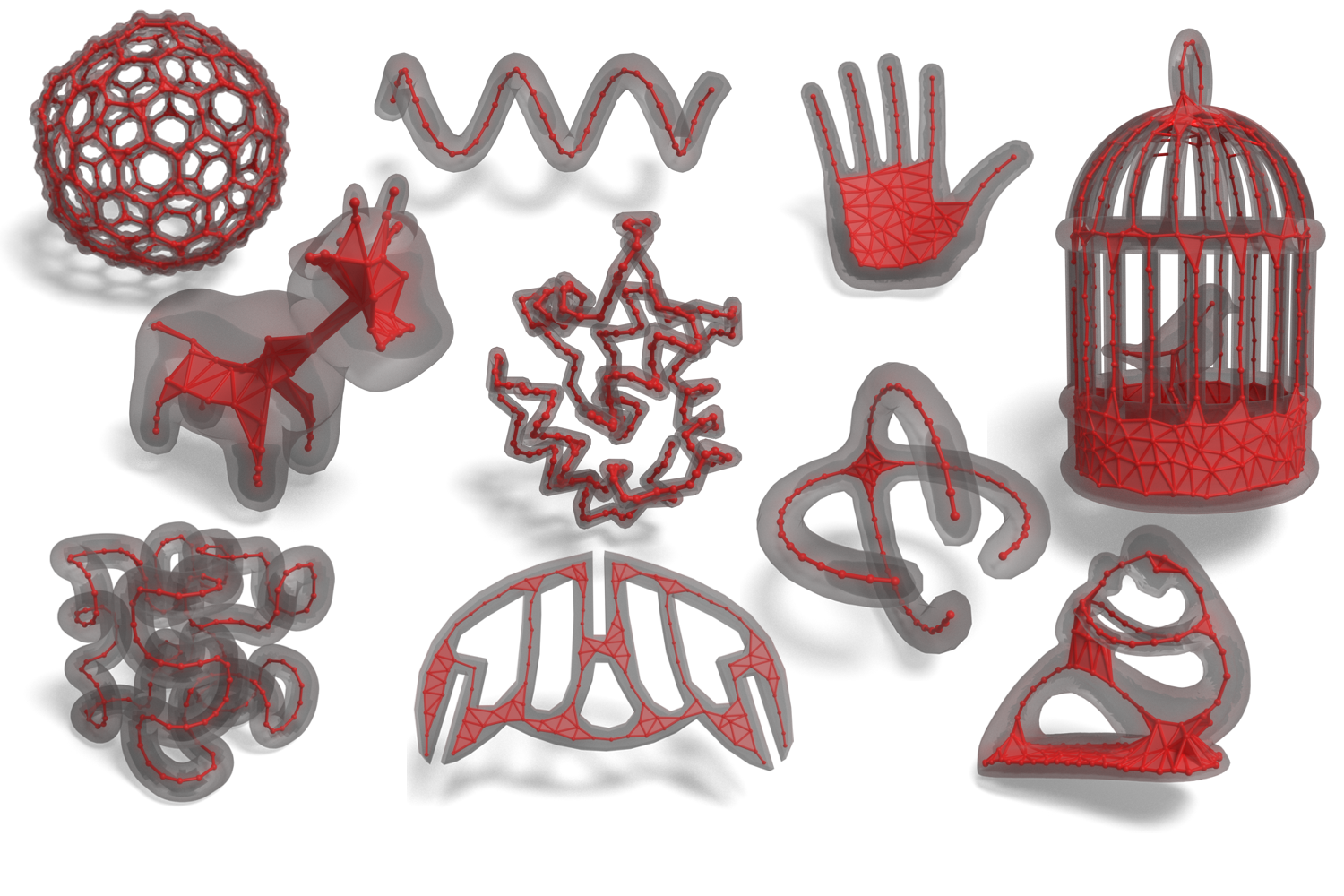
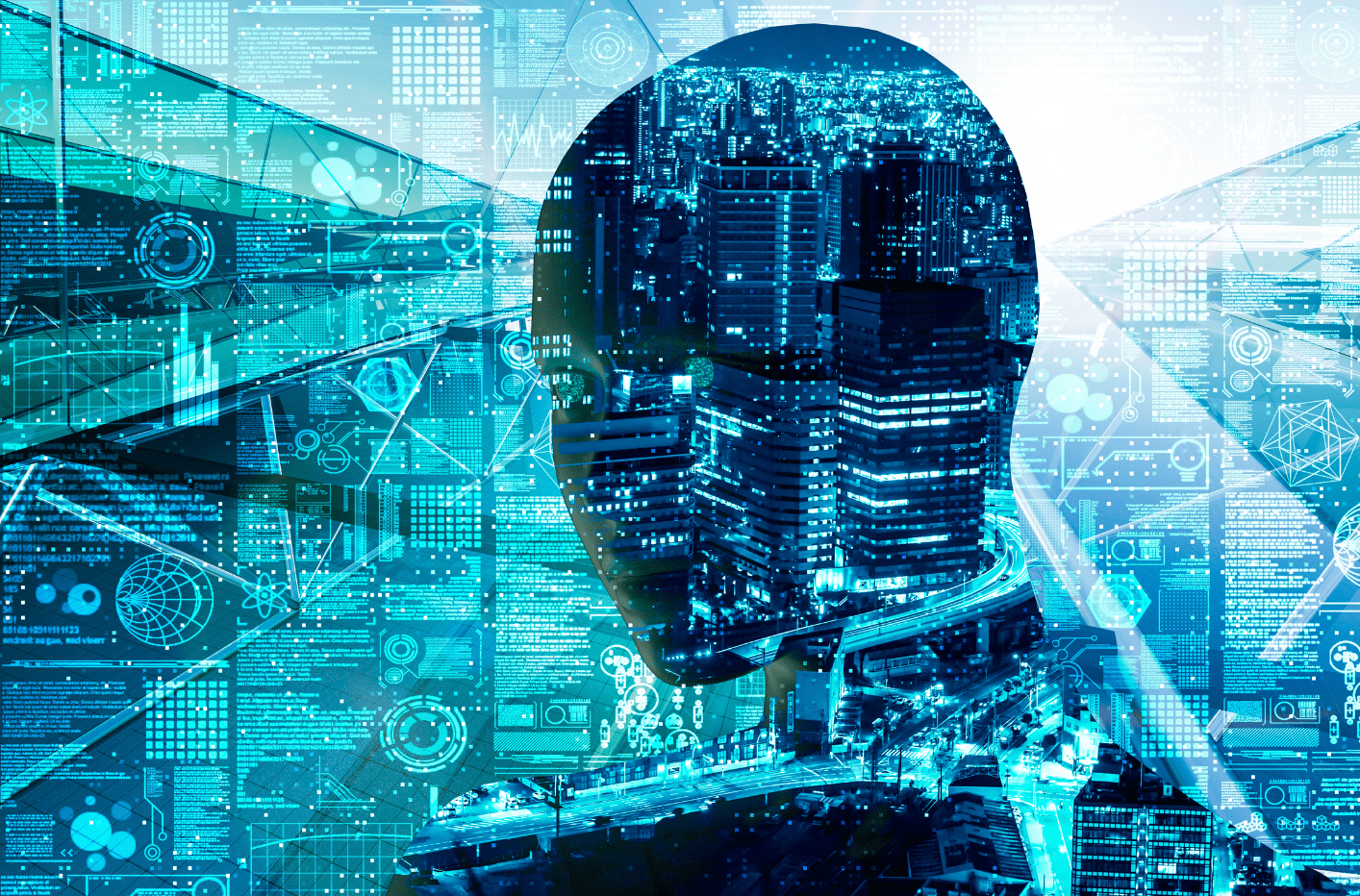
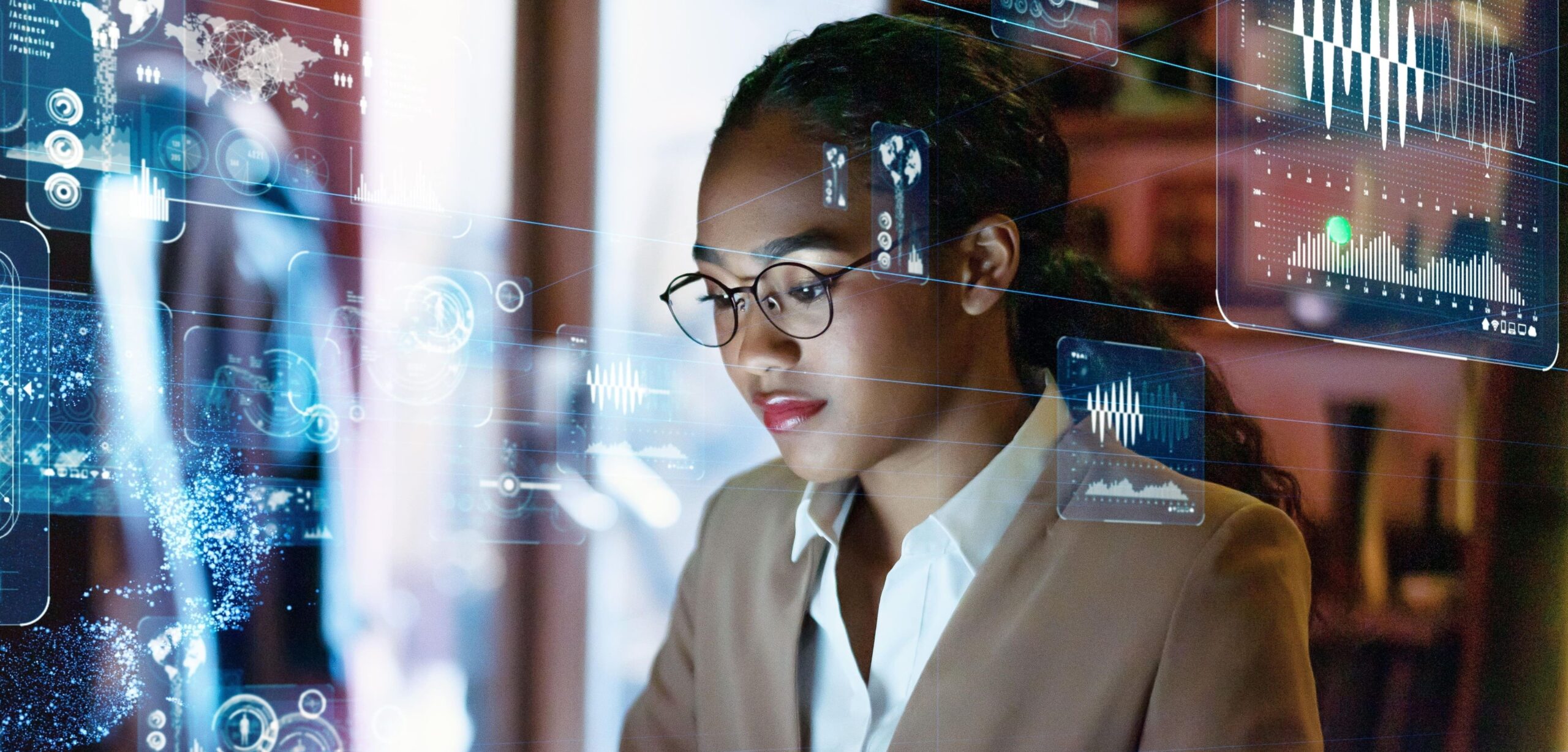
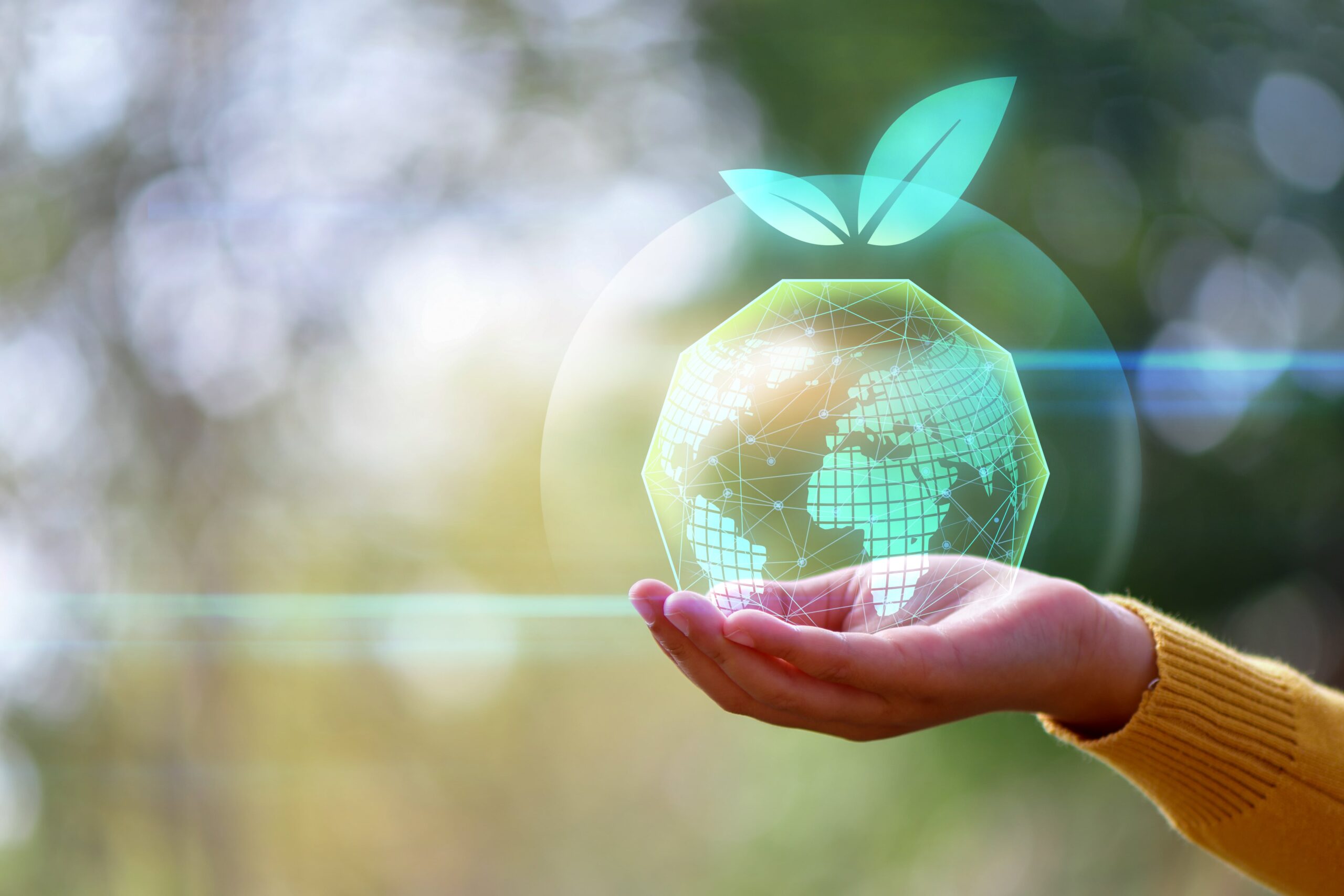