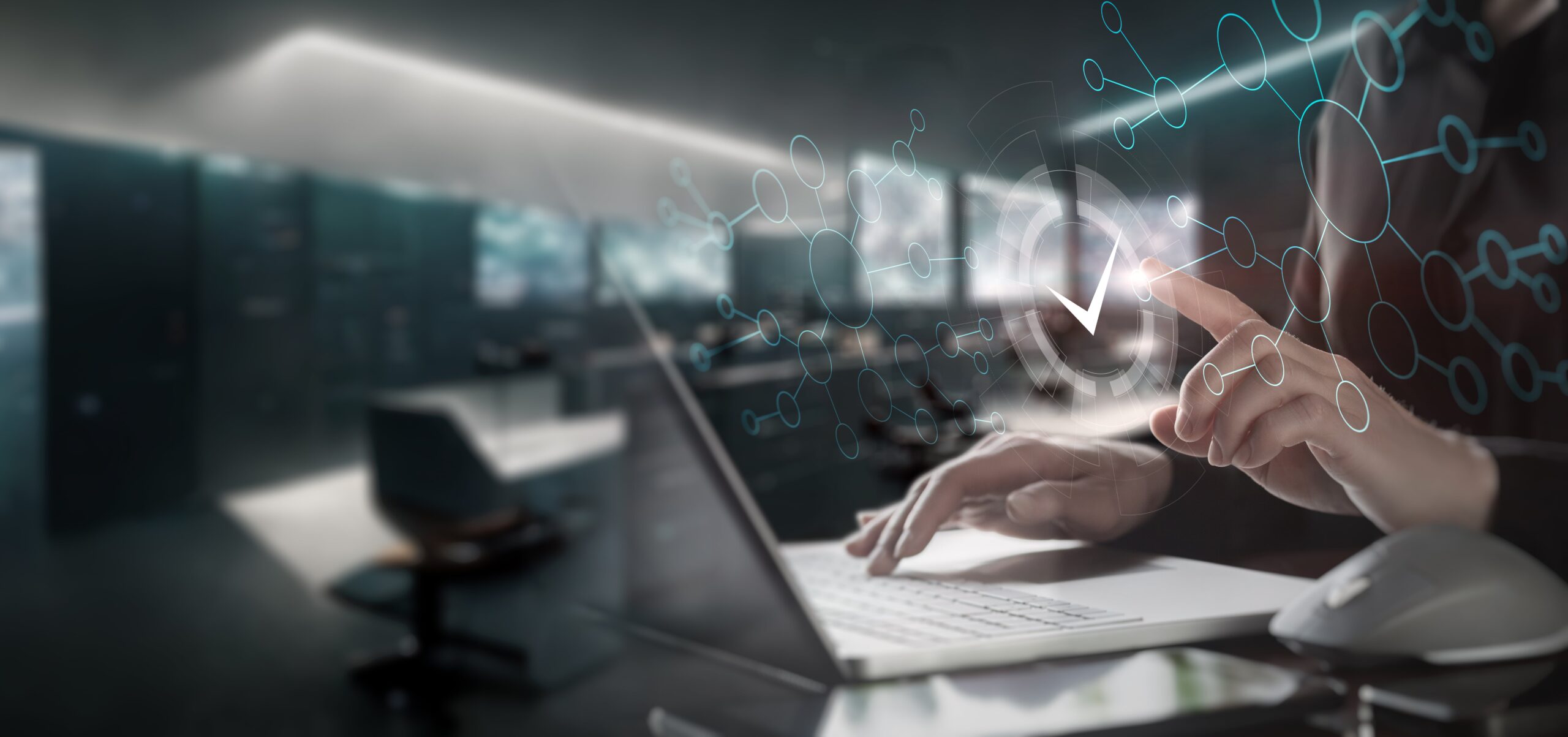
FOUNDRY
The foundations of robustness and reliability in artificial intelligence
Preview
Develop the theoretical and methodological foundations of robustness and reliability needed to build and instill trust in AI technologies and systems.
Panayotis Mertikopoulos, Research Director at CNRS
The core vision of FOUNDRY is that robustness in AI – a desideratum which has eluded the field since its inception – cannot be achieved by blindly throwing more data and computing power to larger and larger models with exponentially growing energy requirements. Instead, we intend to rethink and develop the core theoretical and methodological foundations of robustness and reliability that are needed to build and instill trust in ML-powered technologies and systems from the ground up.
Keywords : Robustness, reliability, game theory, trust, fairness, privacy
Project website : In progress
Missions
Our researches
Achieving resilience to data-centric impediments
Develop algorithms and methodologies for overcoming shortfalls in the models’ training set (outliers, incomplete observations, label shifts, poisoning, etc.), as well as fortifying said models against impediments that arise at inference time.
Adapting to unmodeled phenomena and the environment
Develop the required theoretical and technical tools for AI systems that are able to adapt “on the fly” to non-stationary environments and gracefully interpolate from best- to worst-case guarantees .
Attaining robustness in the presence of concurrent aims and goals
Delineate how robustness criteria interact with standard performance metrics (e.g., a model’s predictive accuracy) and characterize the fundamental performance limits of ML models when the data are provided by selfishly-minded agents.
Consortium
CNRS, Université Paris-Dauphine, INRIA, Institut Mines Télécom, Ecole normale supérieure de Lyon, Université de Lille, ENSAE Paris, Ecole Polytechnique Palaiseau
Accelerate fundamental research related to the design of robust and reliable AI systems.
The results of the project will have a significant impact on the state of the art in handling corrupted data, robust learning, and incorporating fairness and privacy guarantees into current and emerging machine learning models without sacrificing predictive accuracy or other performance metrics.
From automated hospital admission systems powered by machine learning (ML), to flexible chatbots capable of fluent conversations and self-driving cars, the wildfire spread of artificial intelligence (AI) has brought to the forefront a crucial question with far-reaching ramifications for the society at large: Can ML systems and models be relied upon to provide trustworthy output in high-stakes, mission- critical environments?
This project aims to provide the theoretical and mathematical underpinnings to make these applications reliable and trustworthy, and in part address these societal concerns about the widespread use of machine learning models and predictions.
The project therefore also aims to stimulate innovation for large companies, SMEs and start-ups, by fostering collaboration between industrial and academic stakeholders.
A community of around 25 permanent researchers, university professors, and engineers, as well as 20 PhD students, 10 post-docs and 5 contract research engineers as the project progresses.
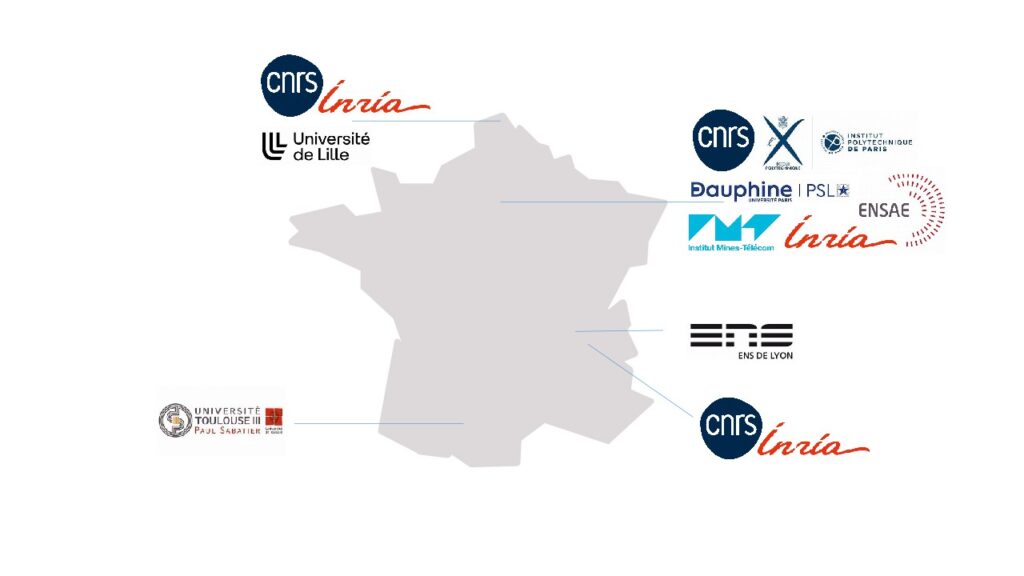
Publication
Autres projets
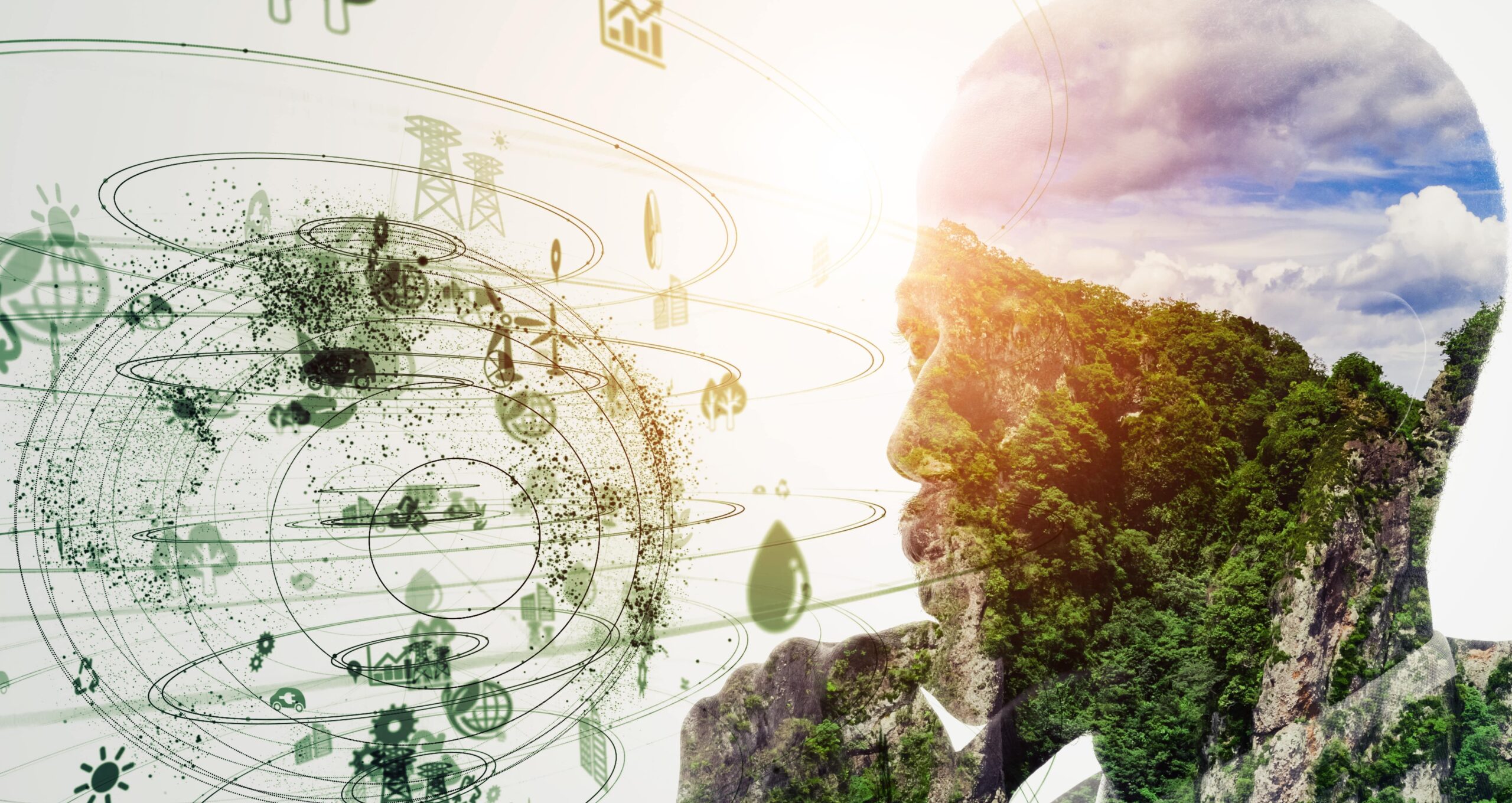
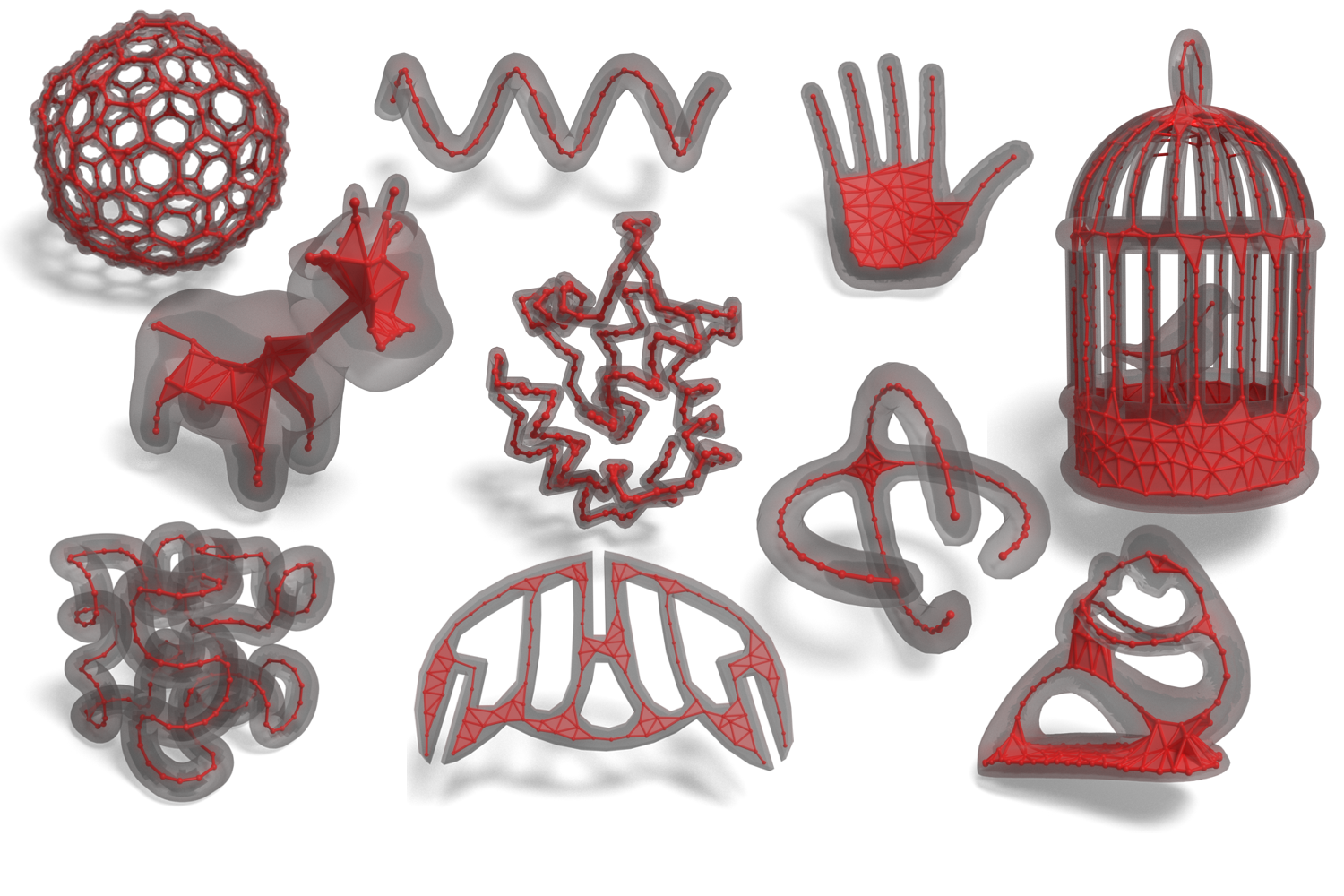
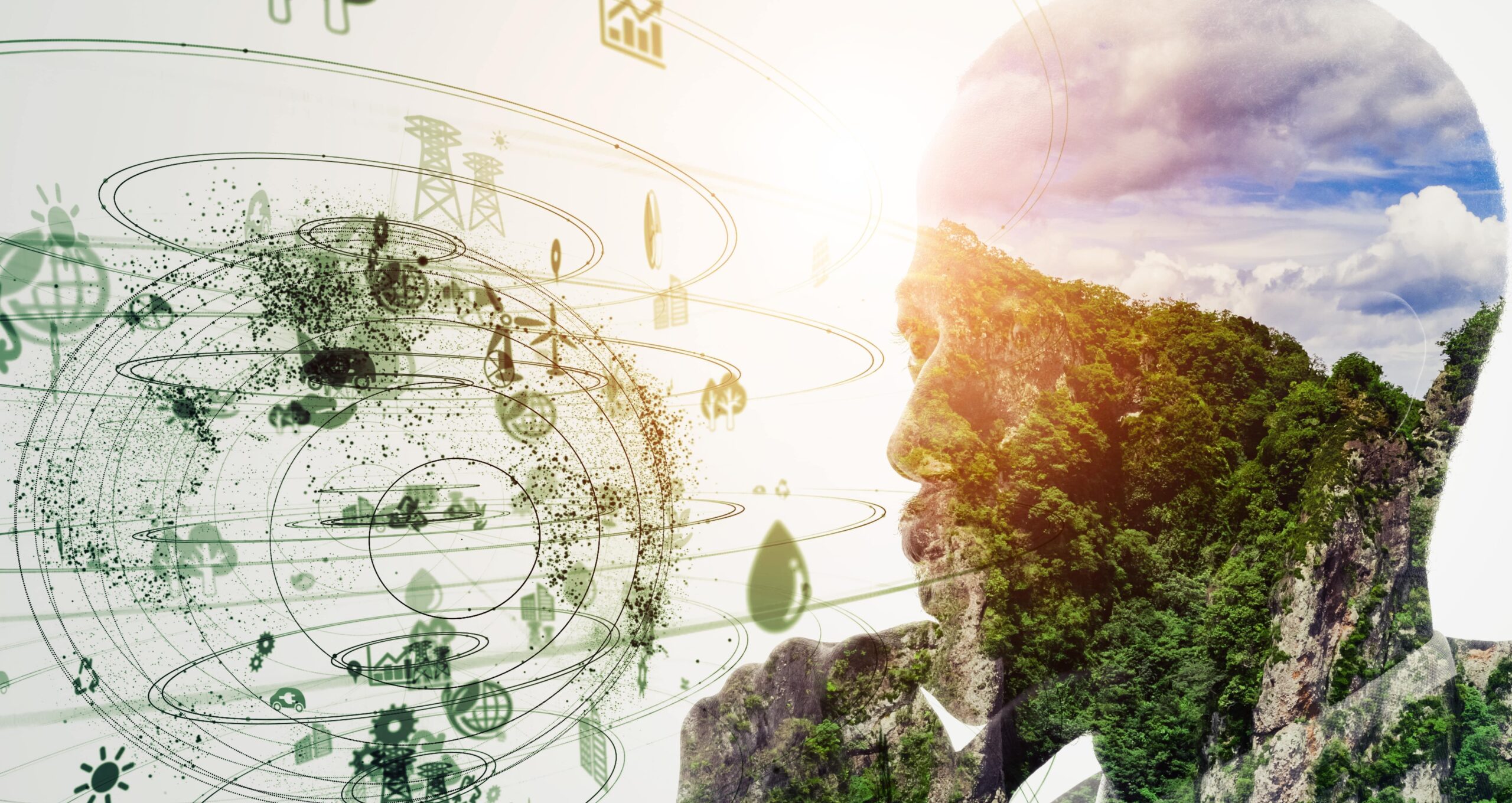
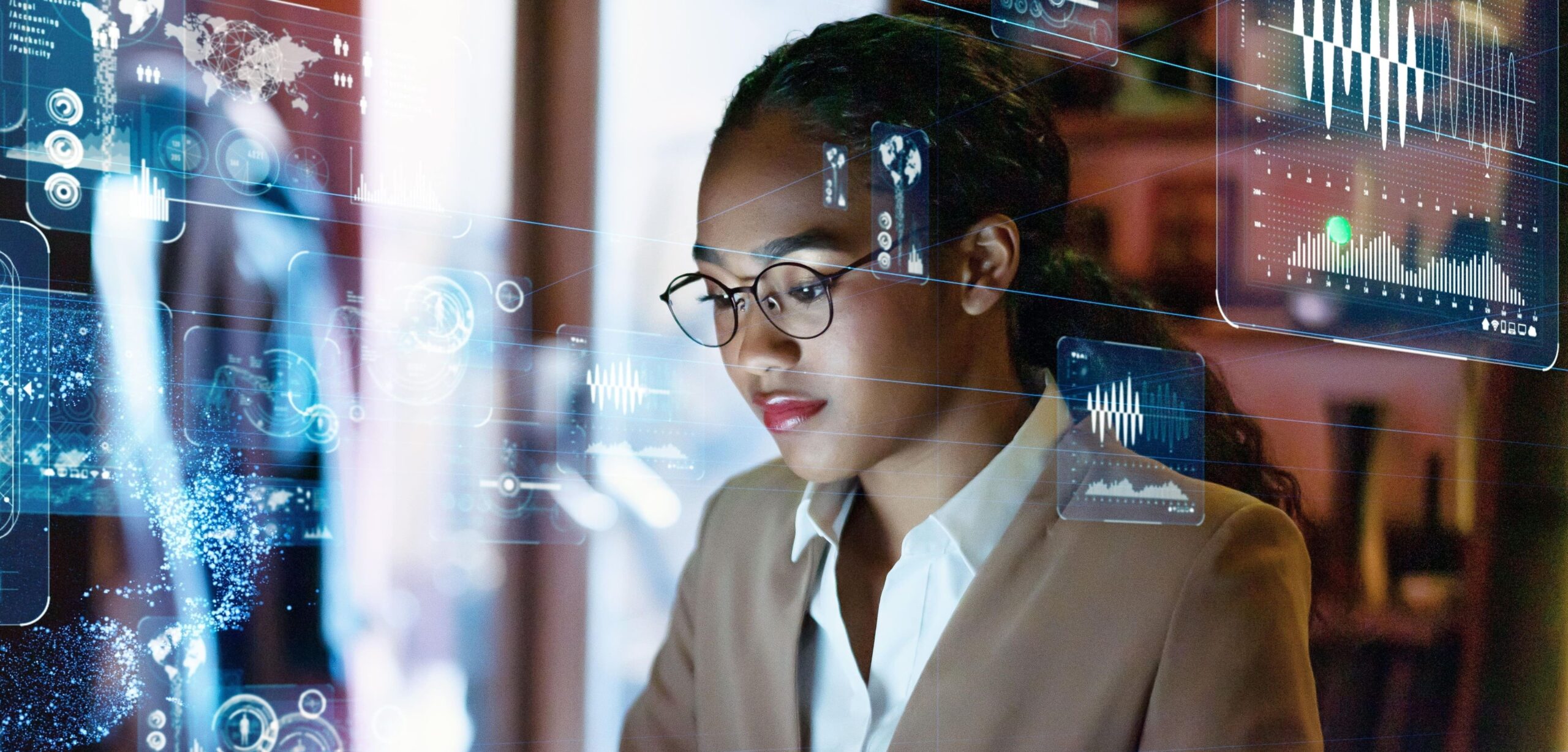
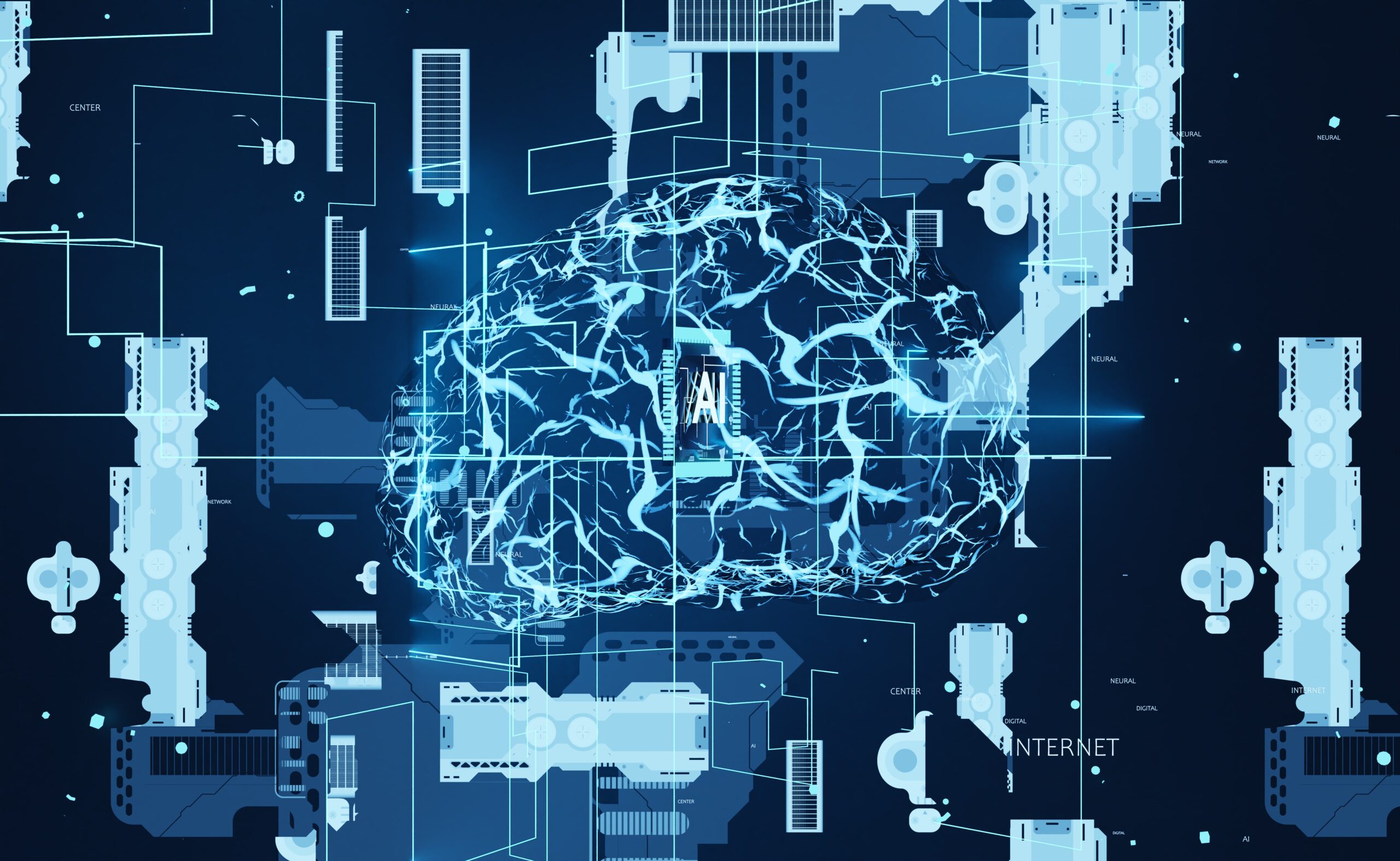
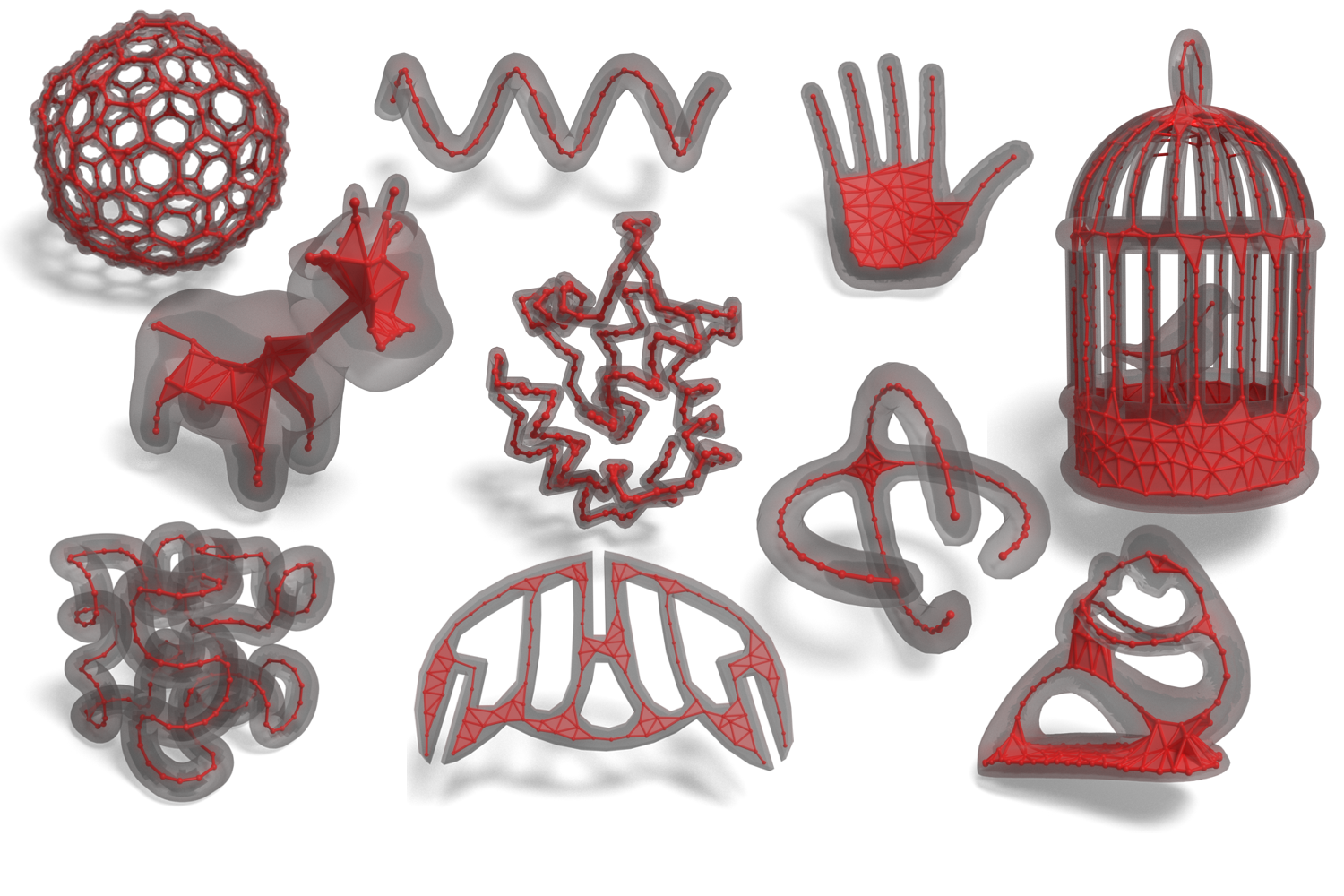
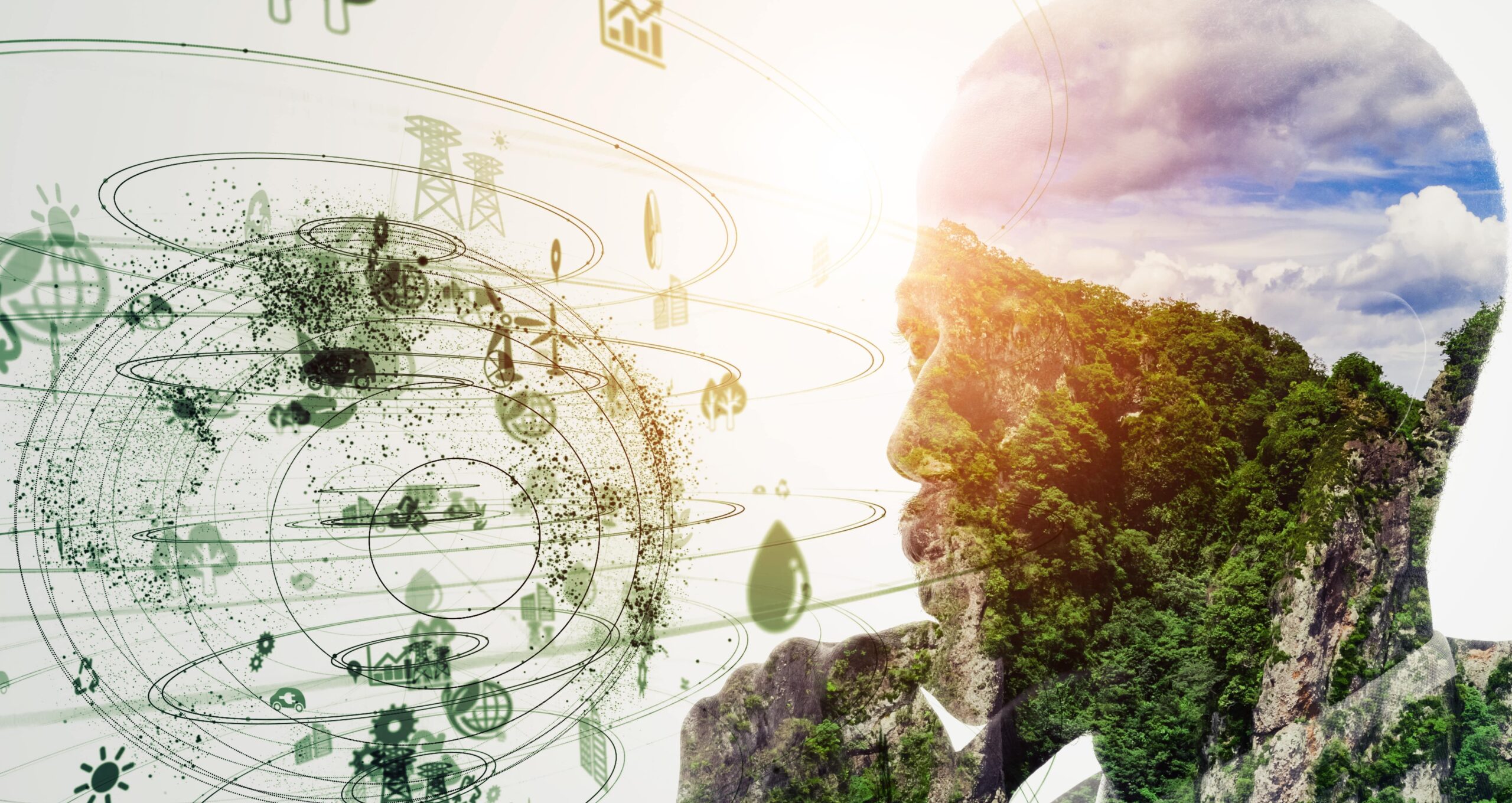
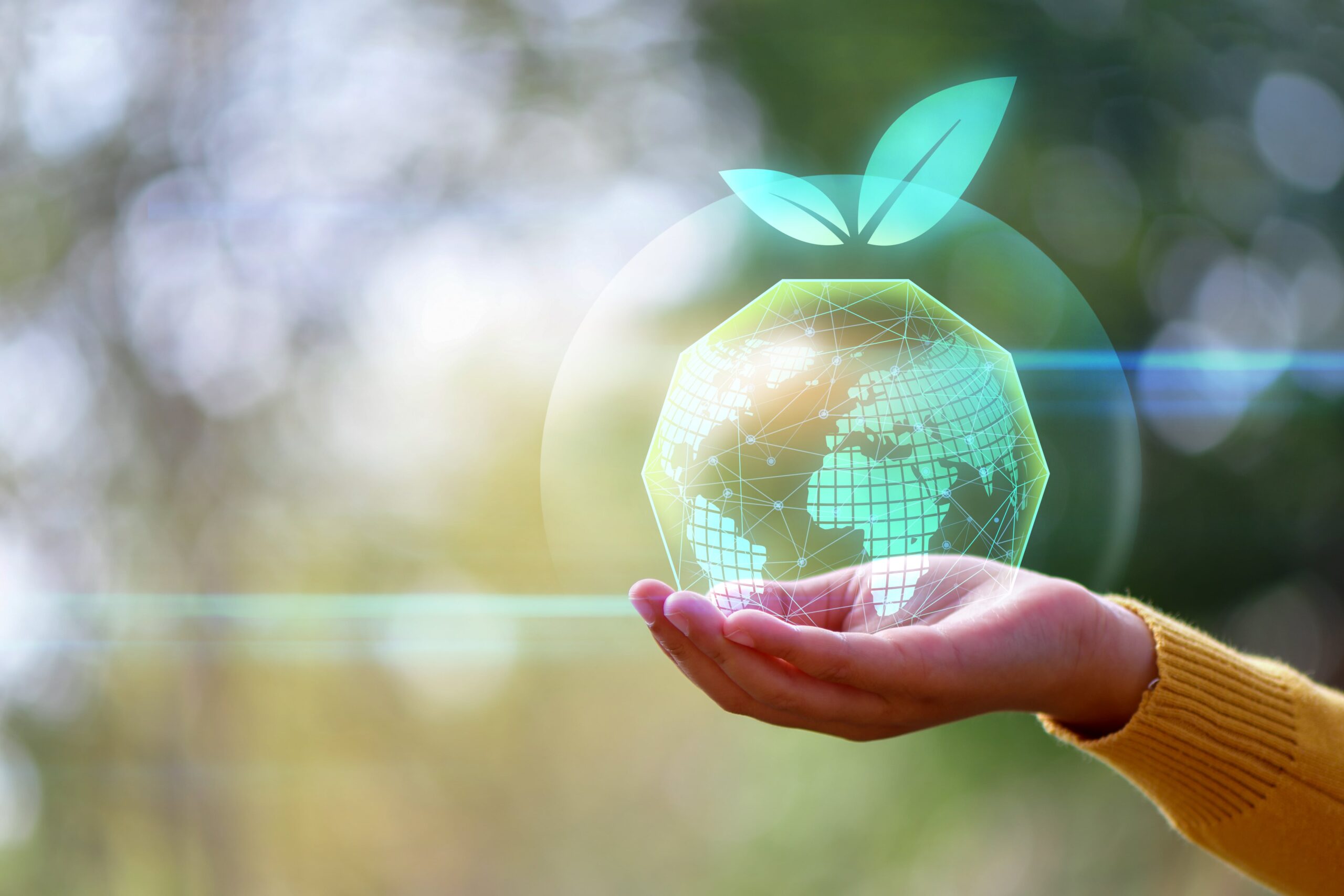
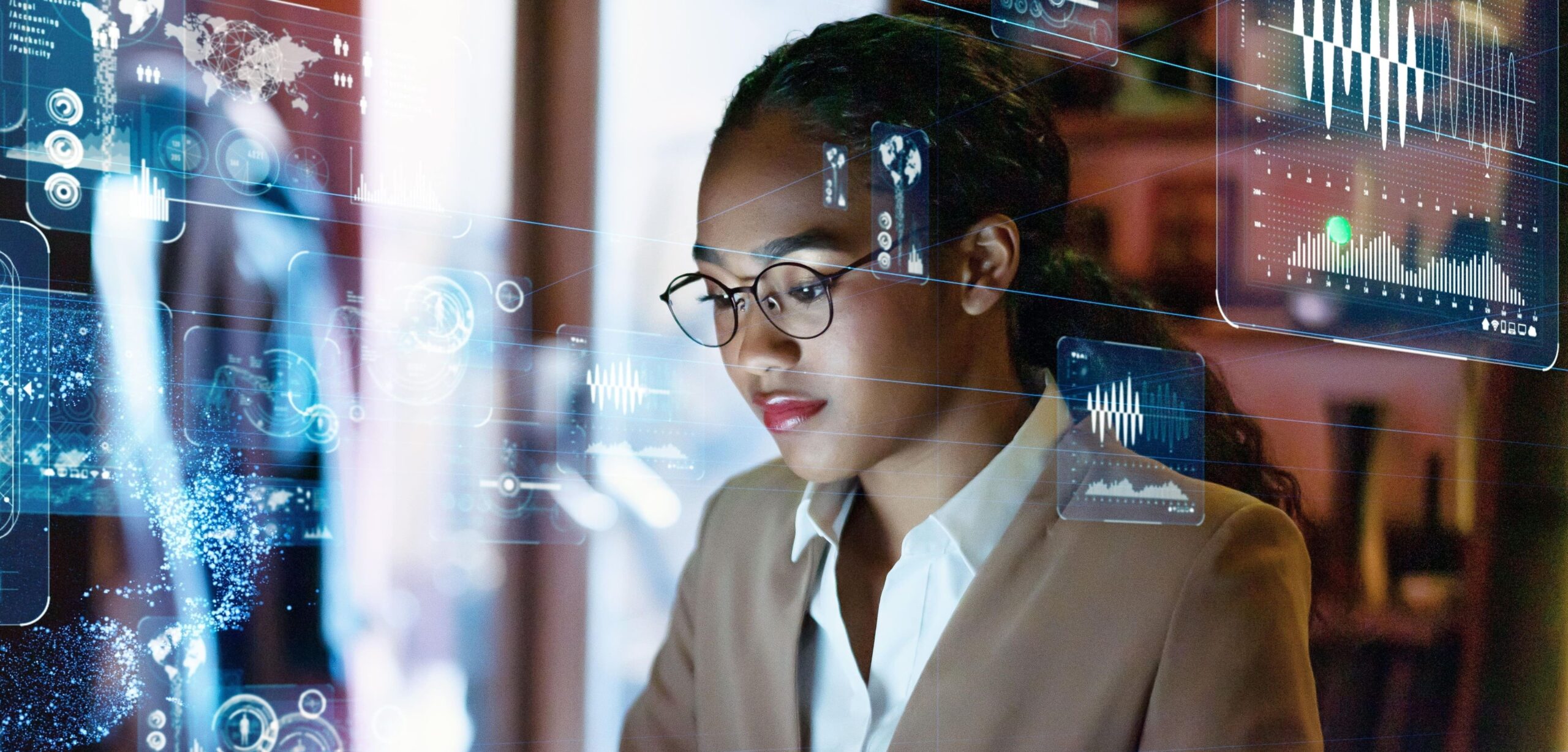
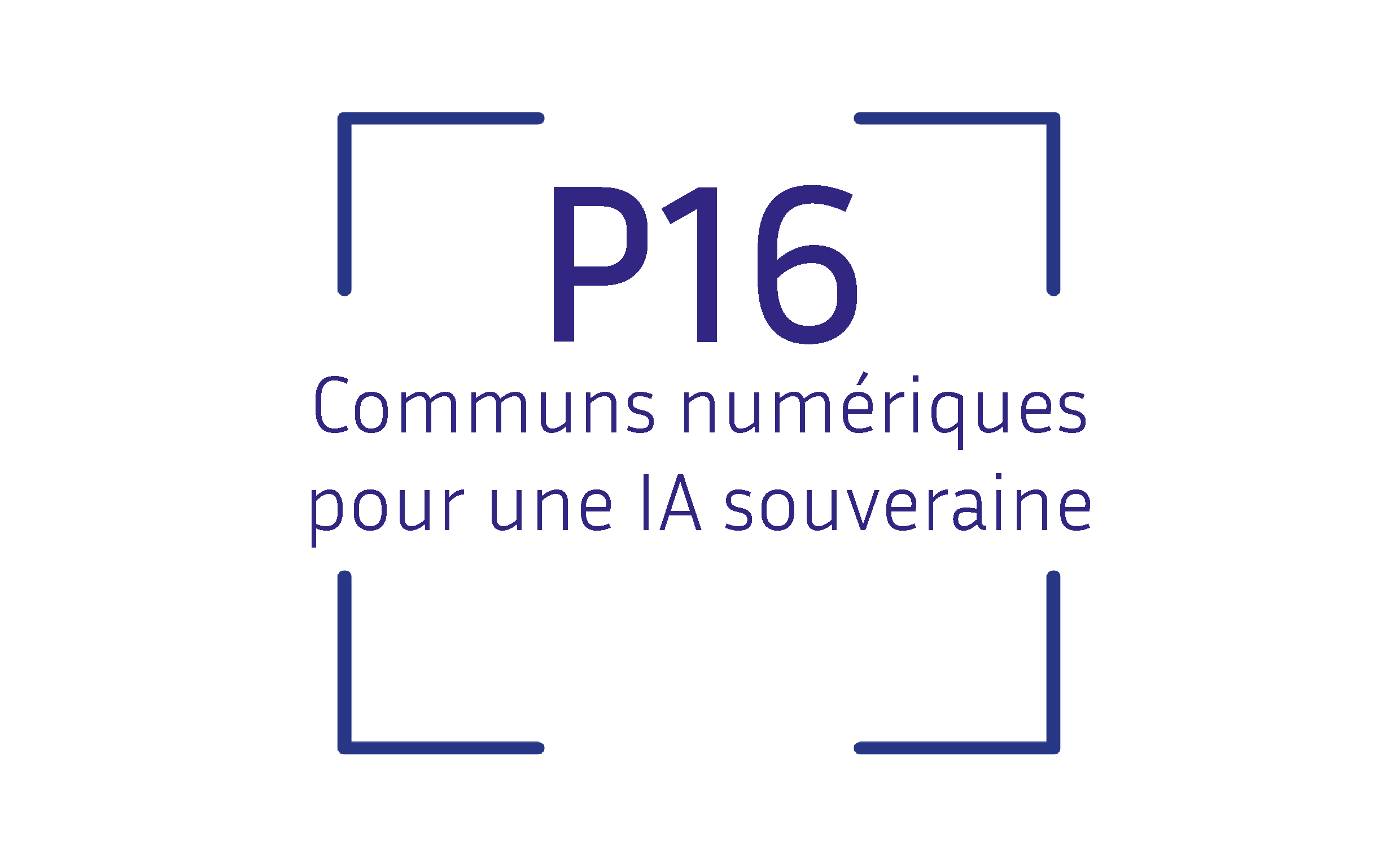
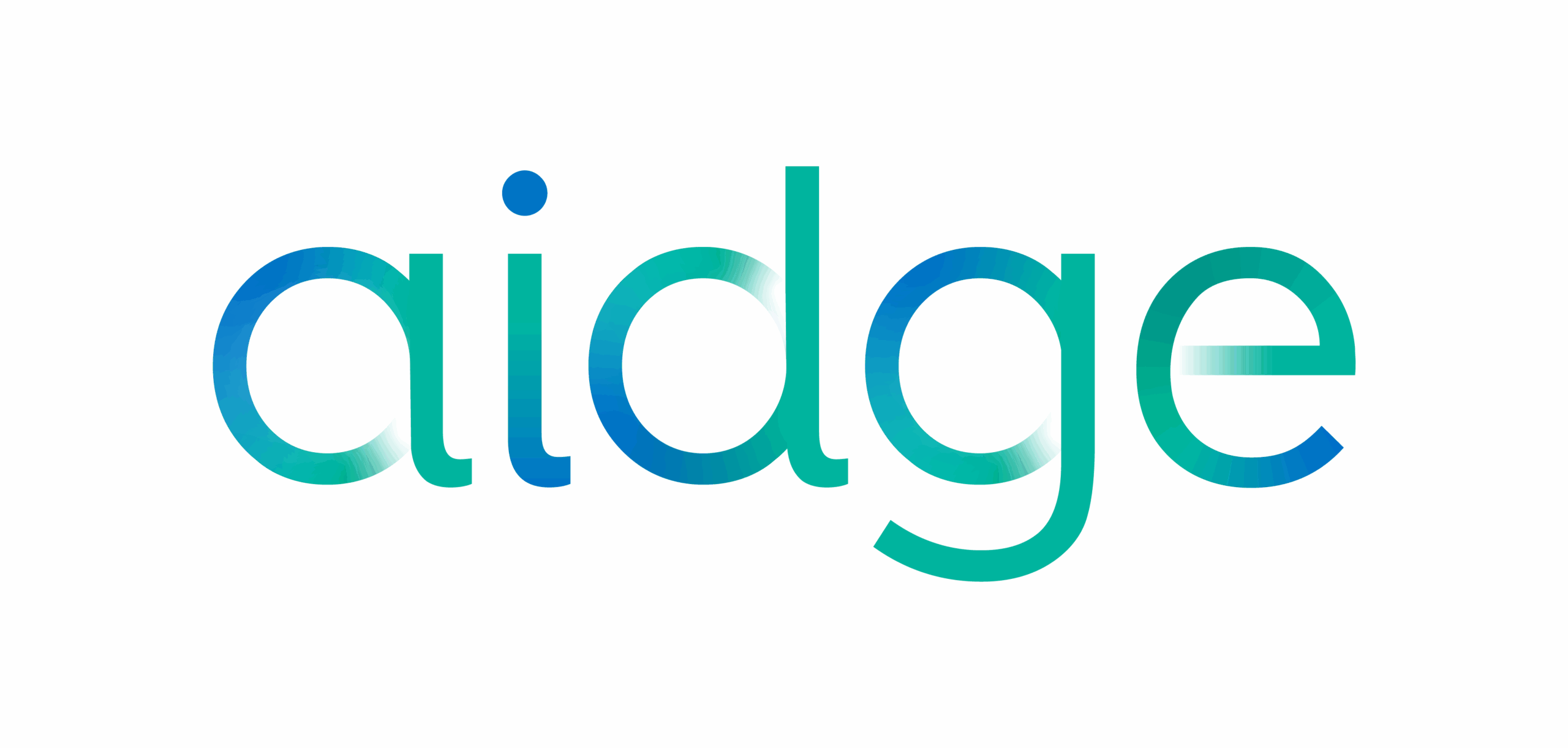
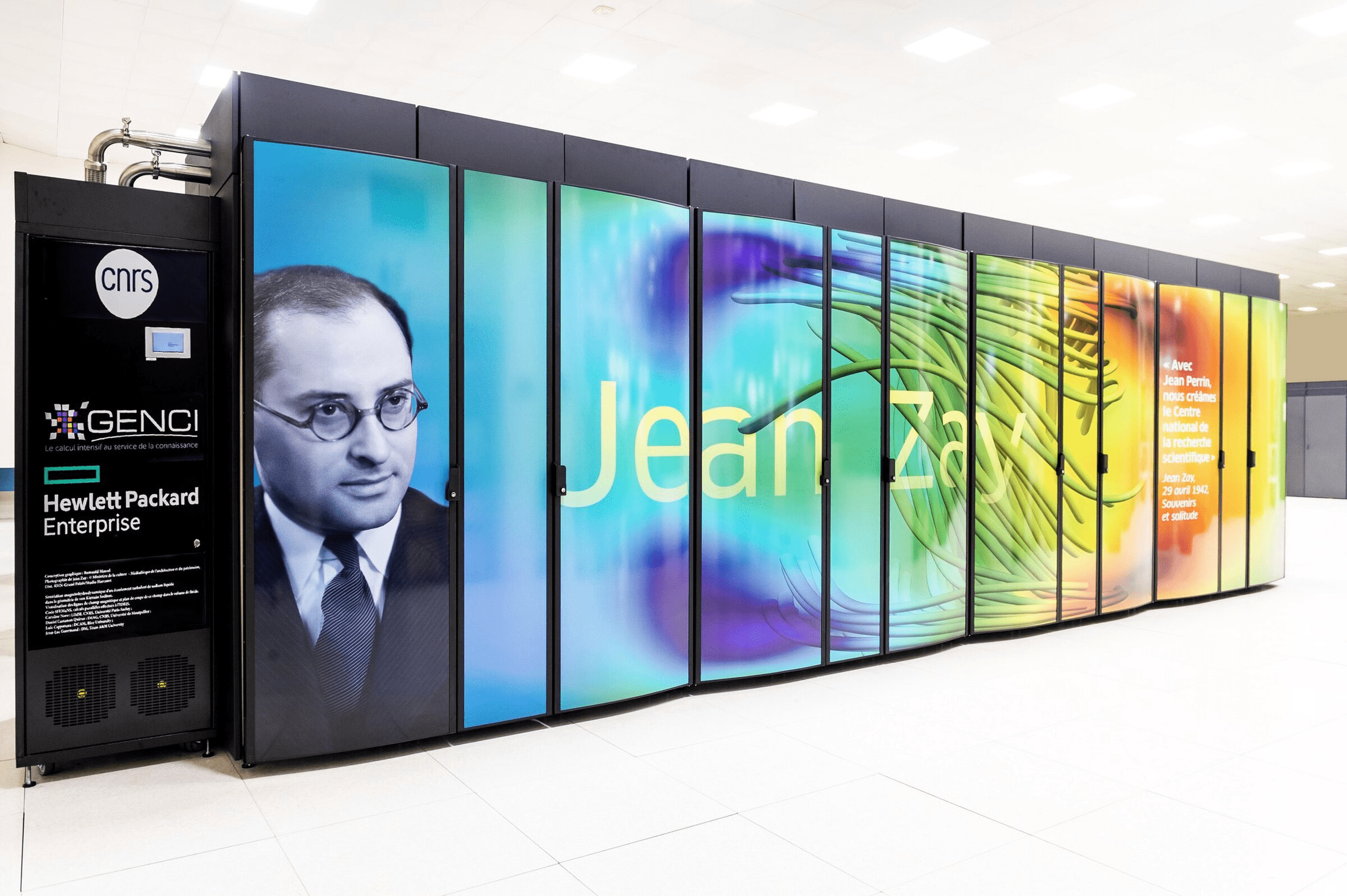
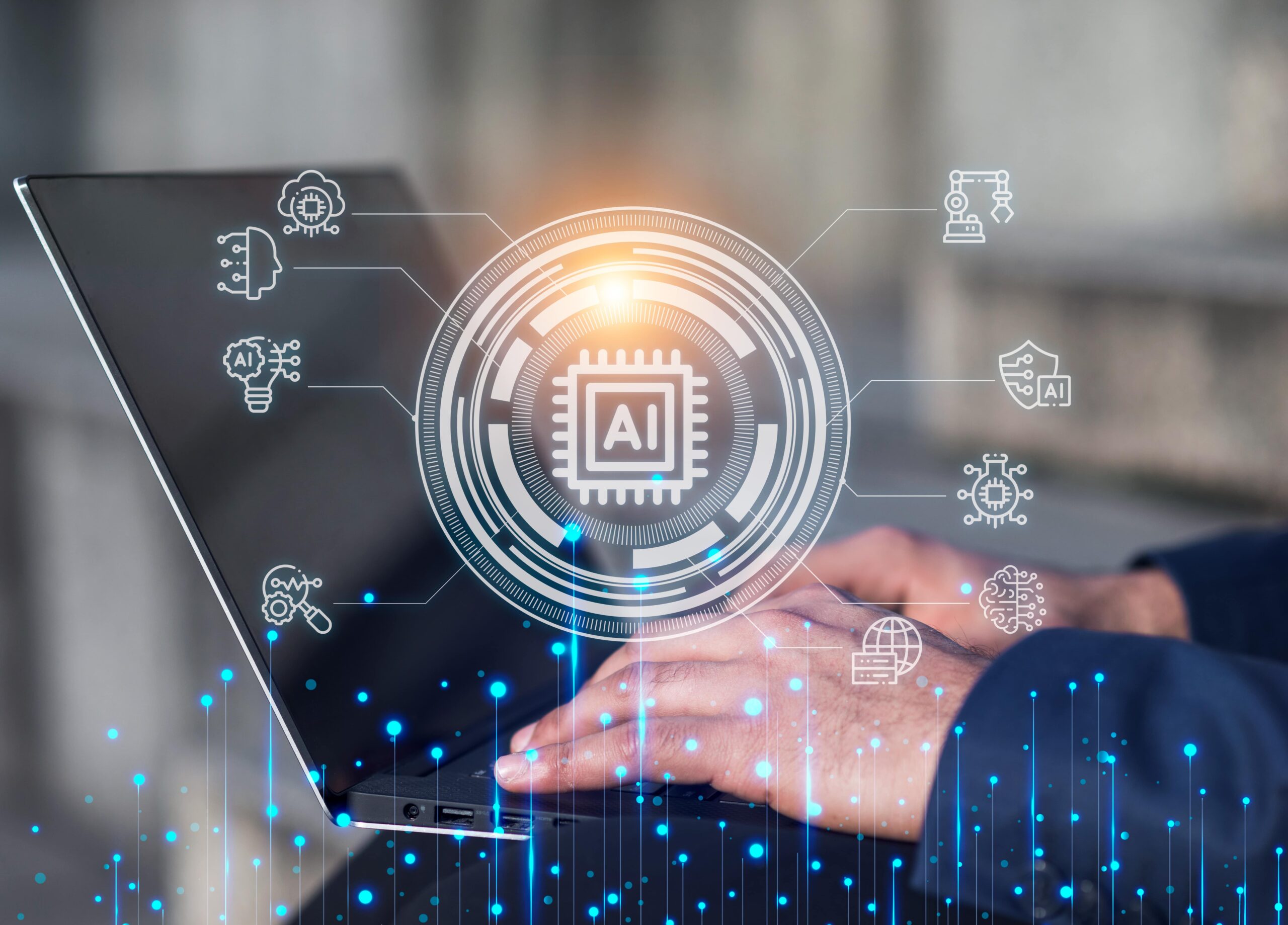
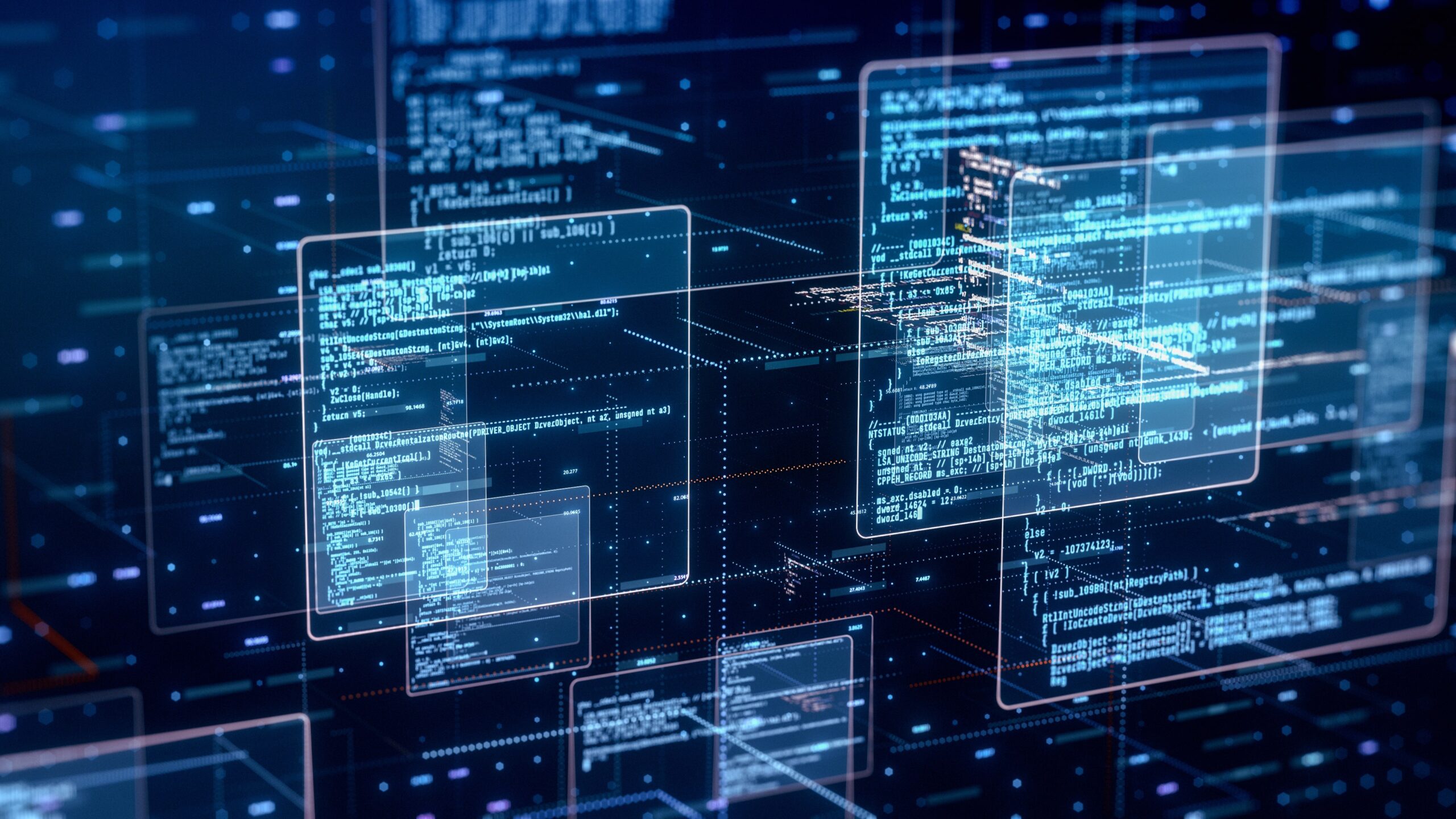
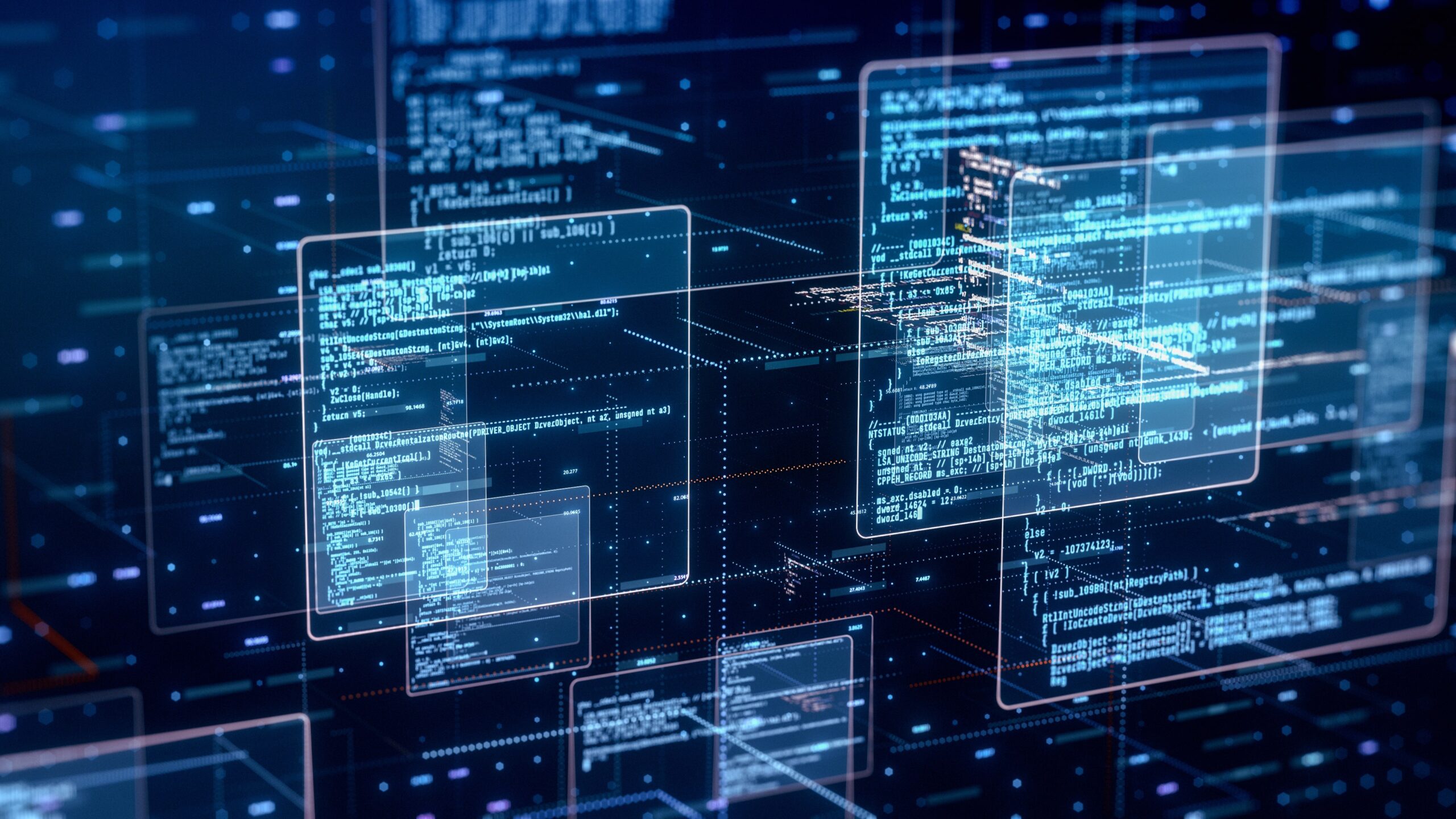
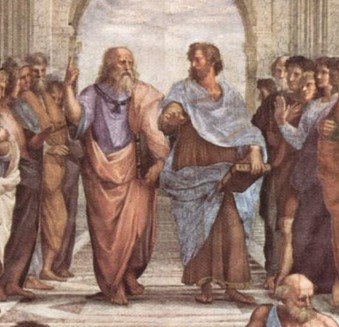
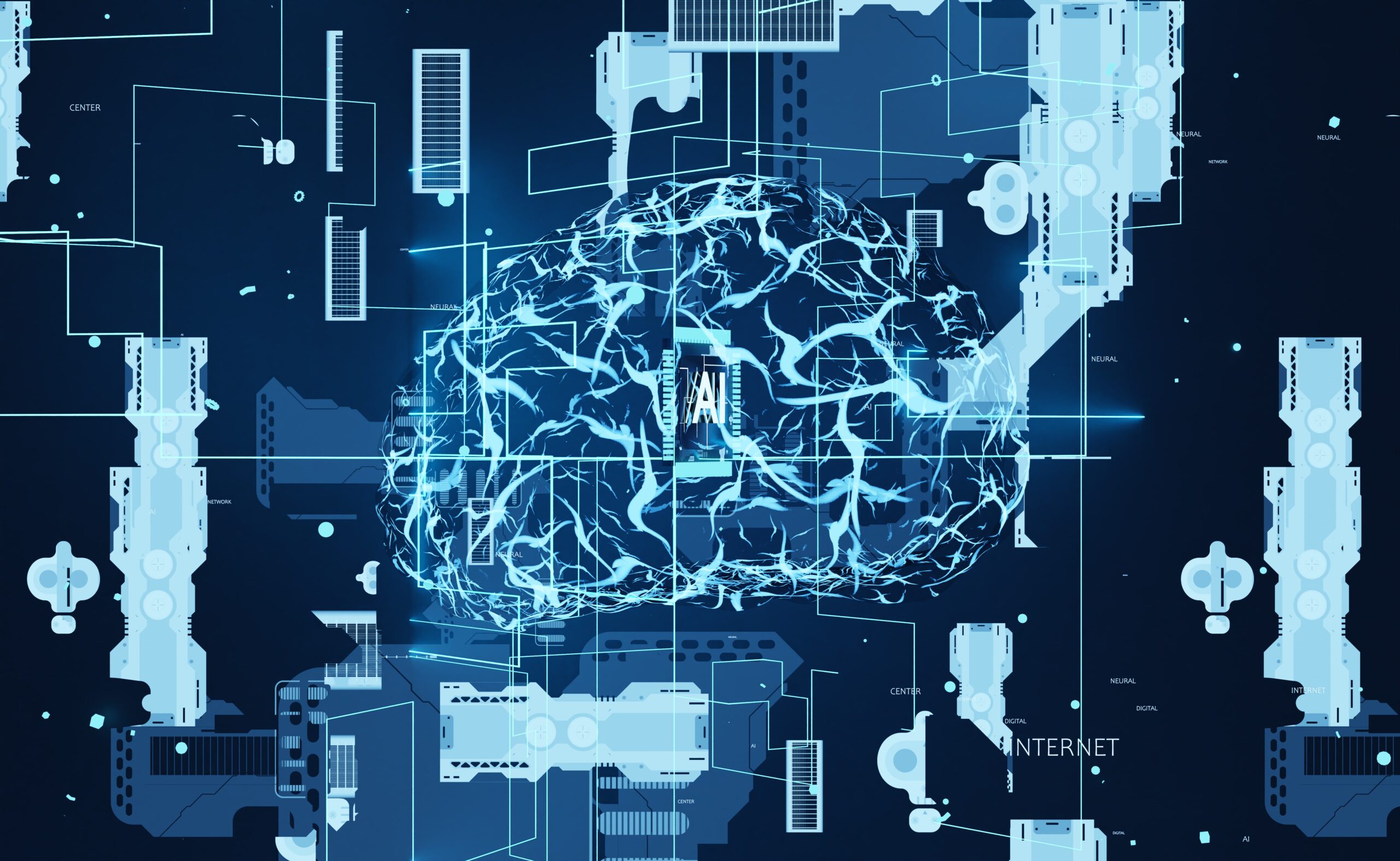
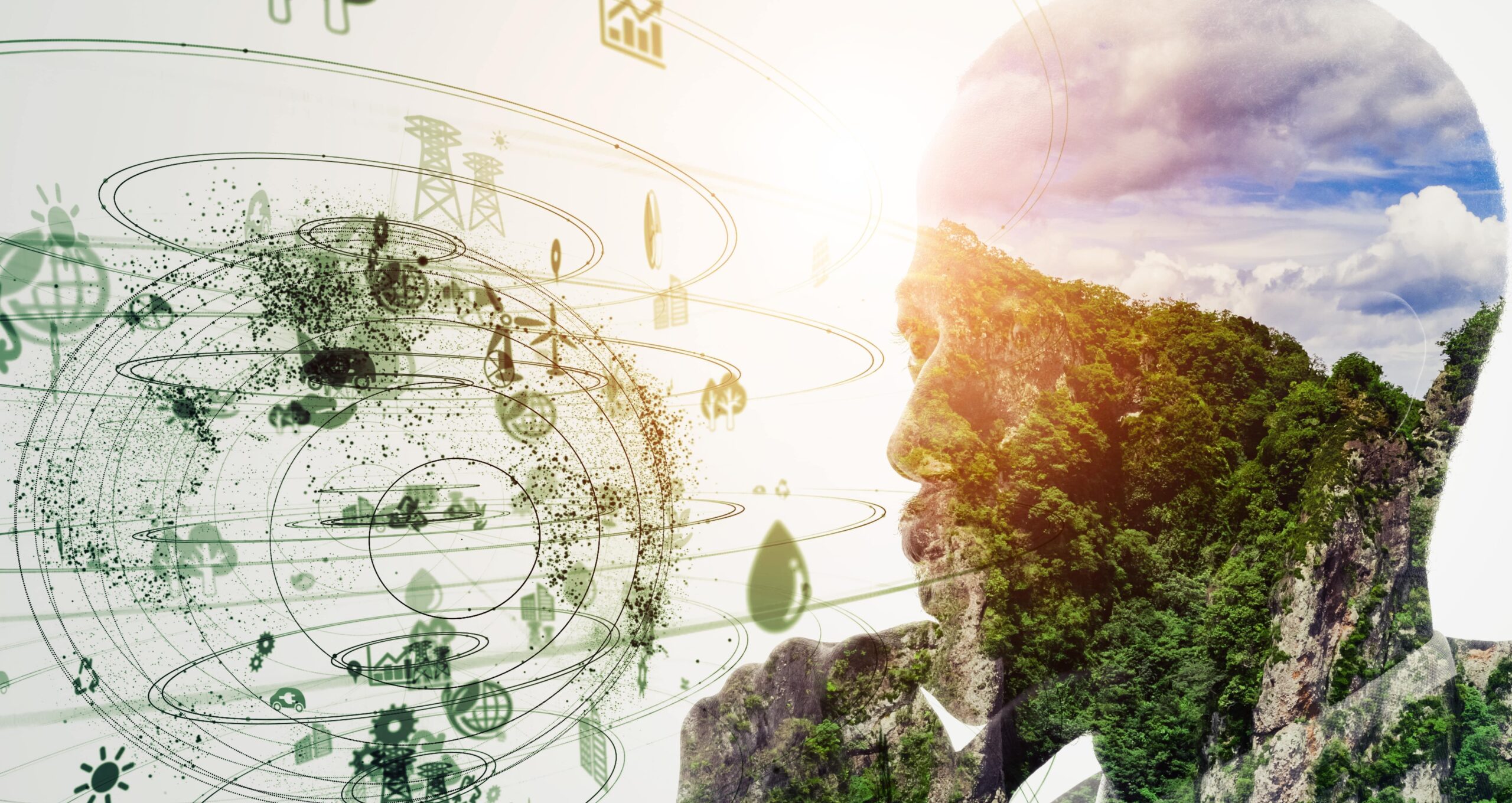
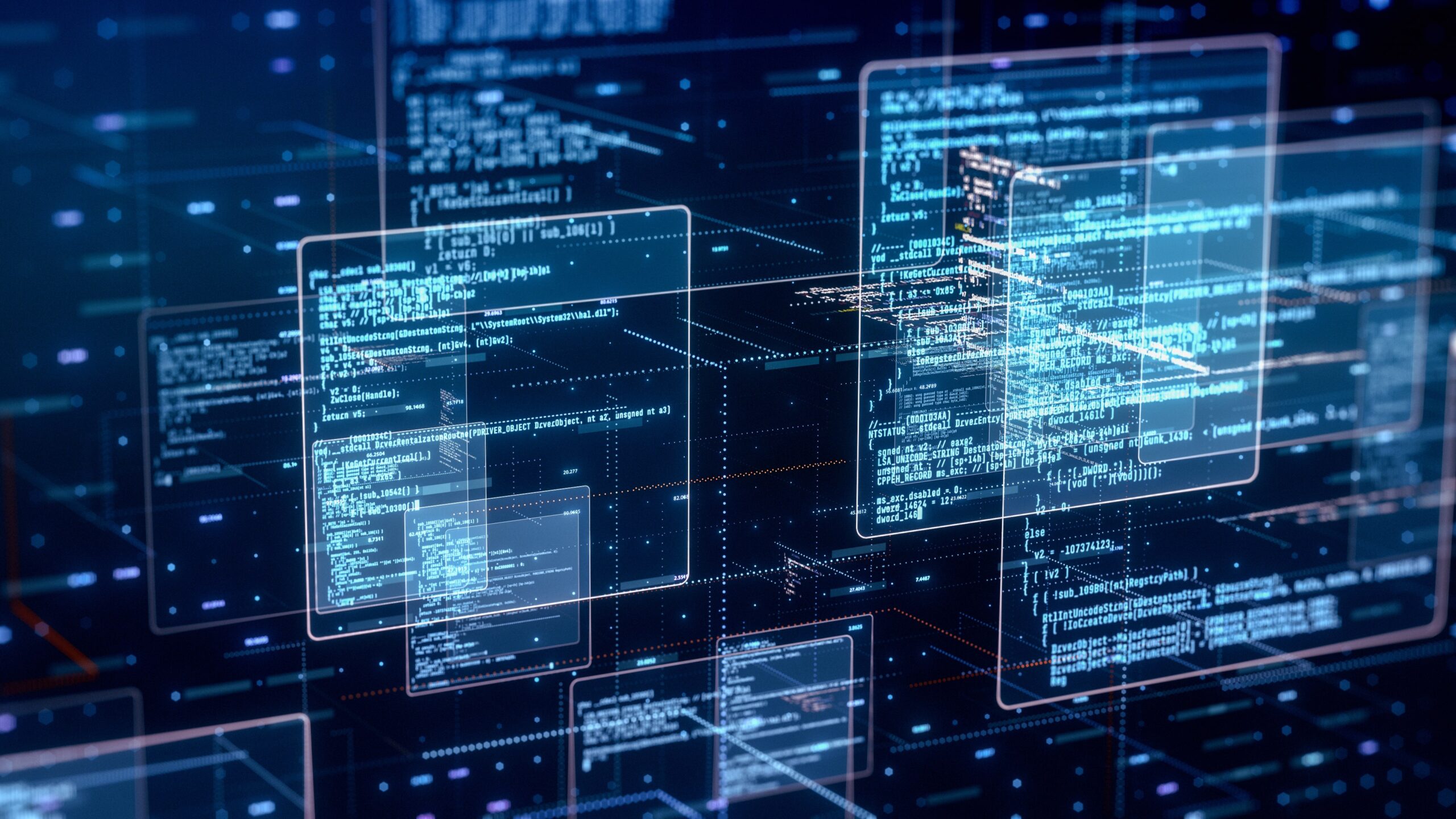
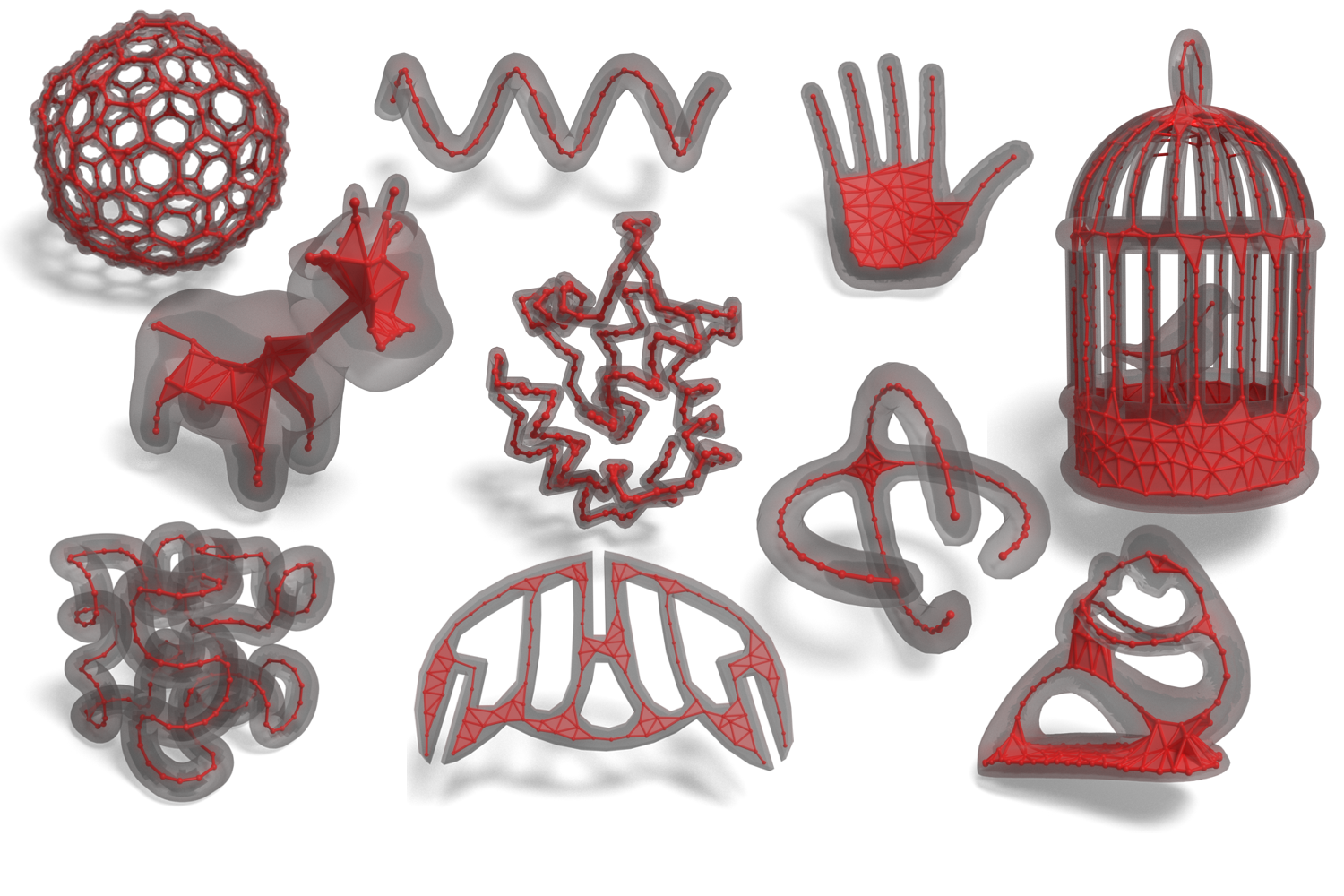
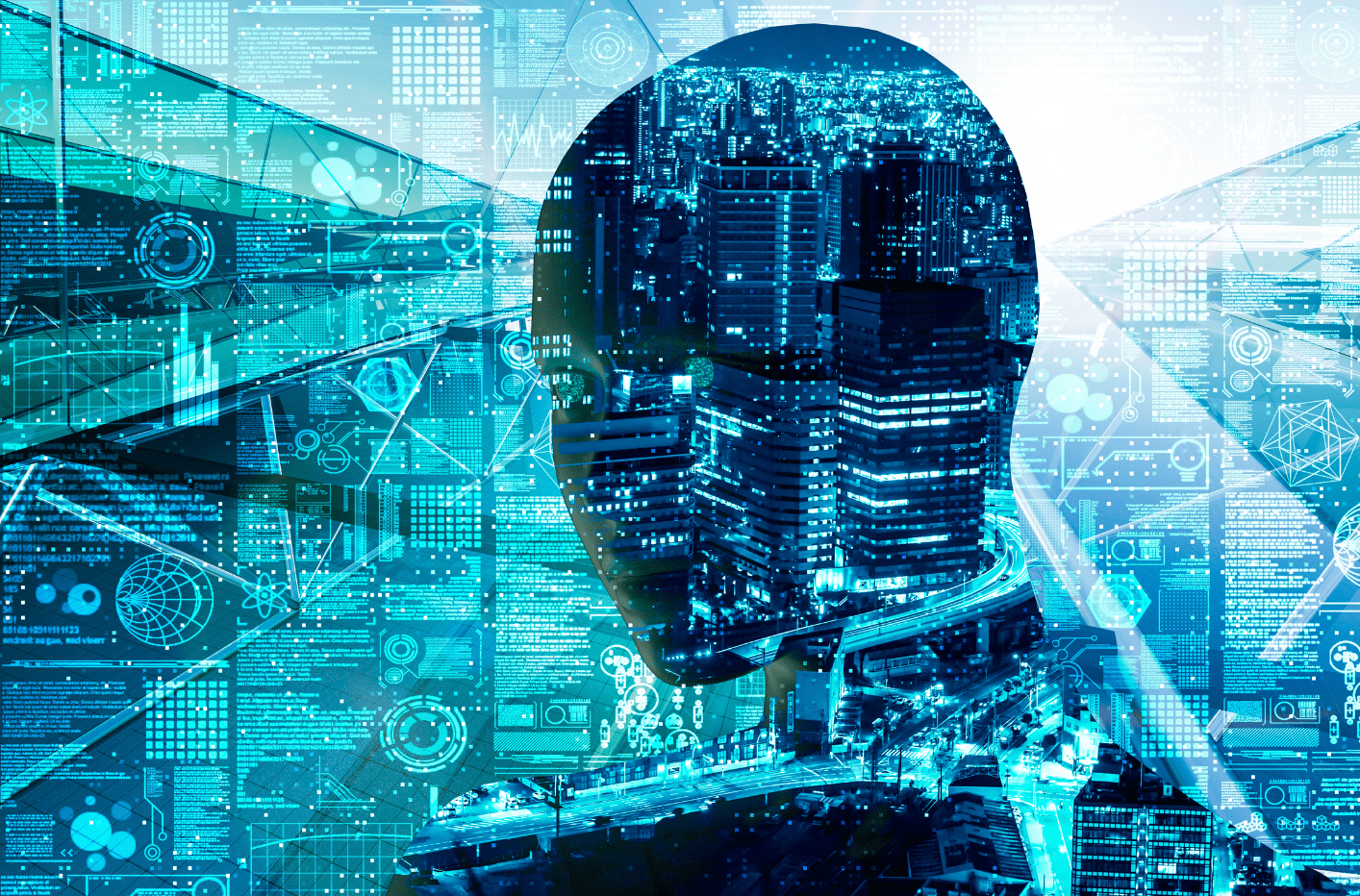
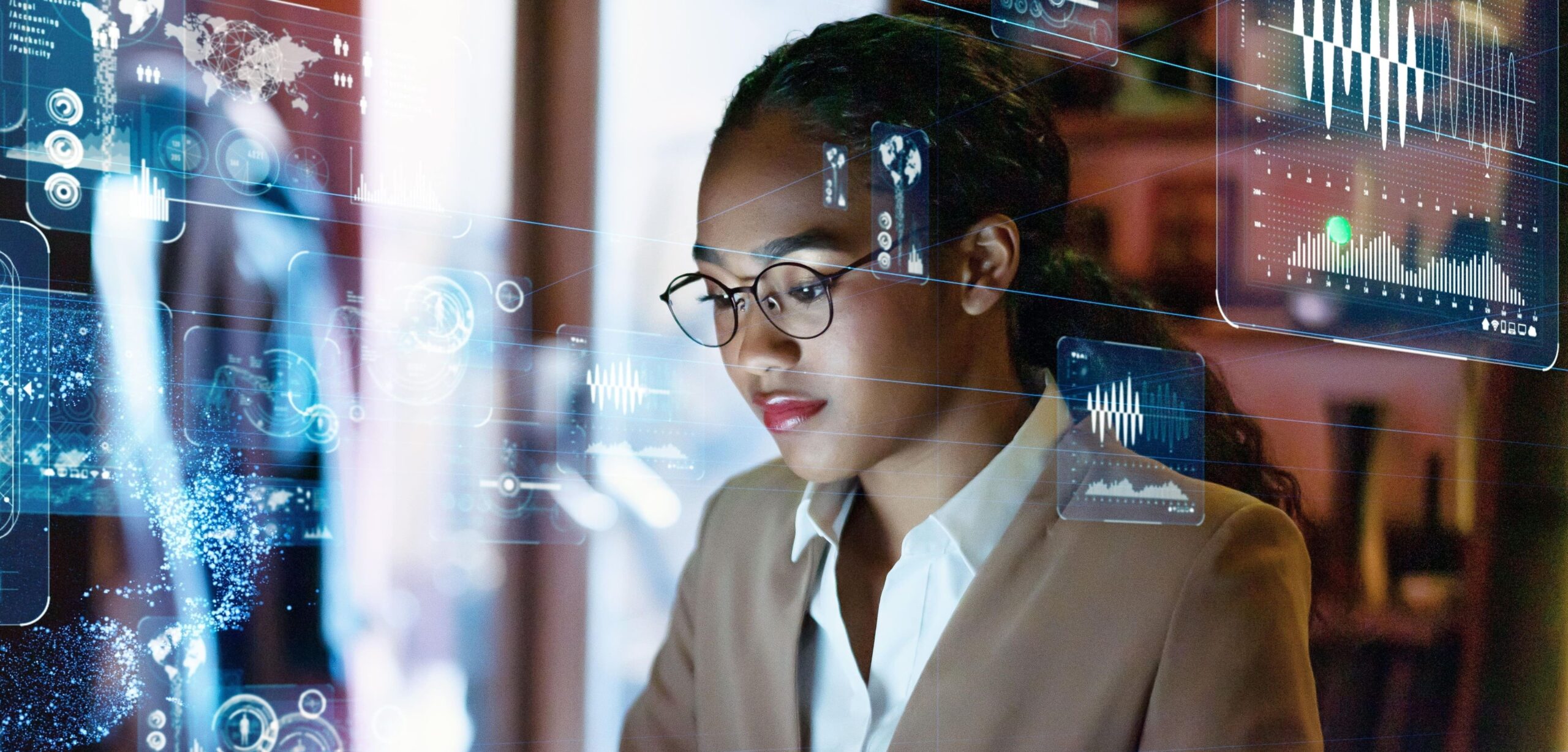
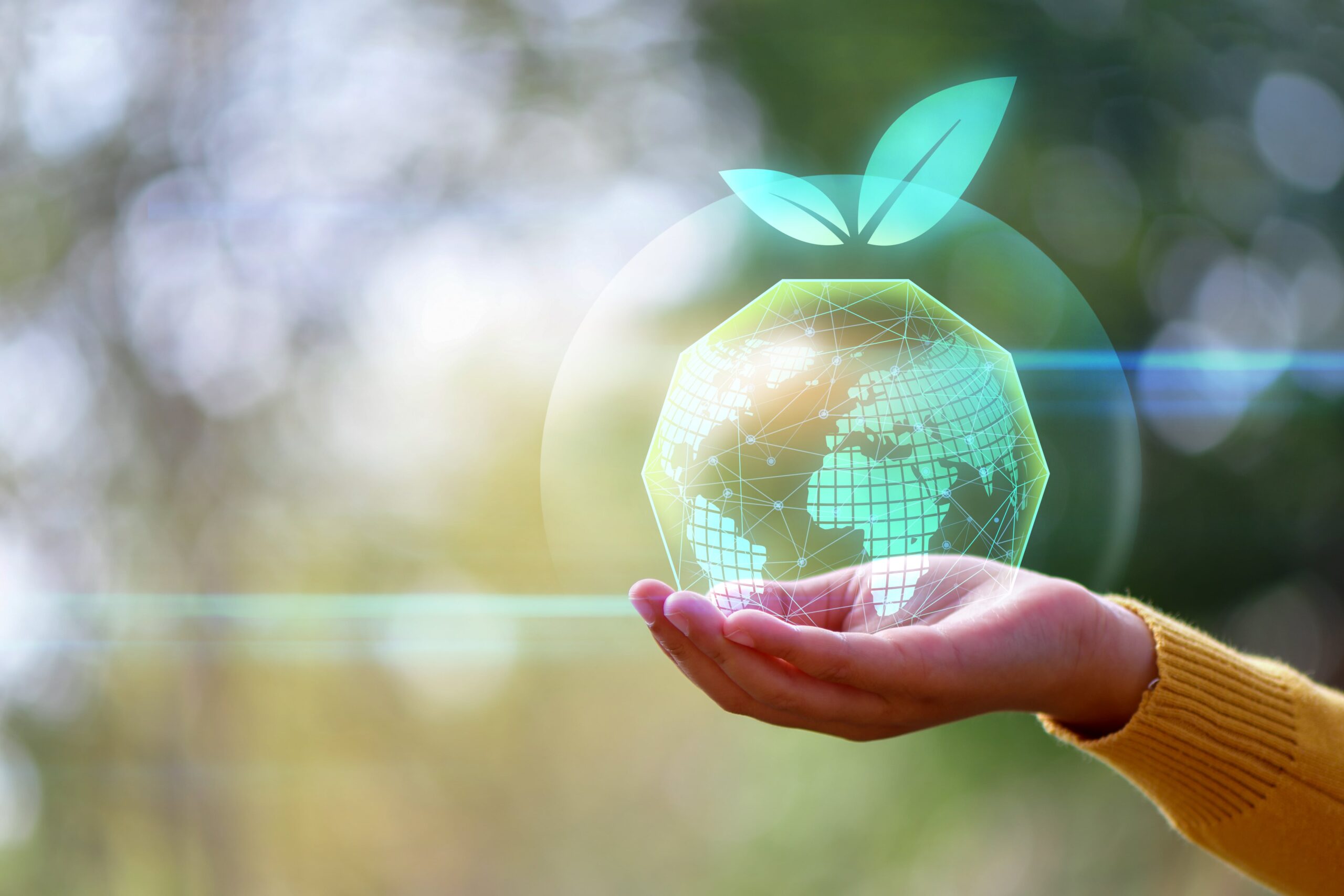