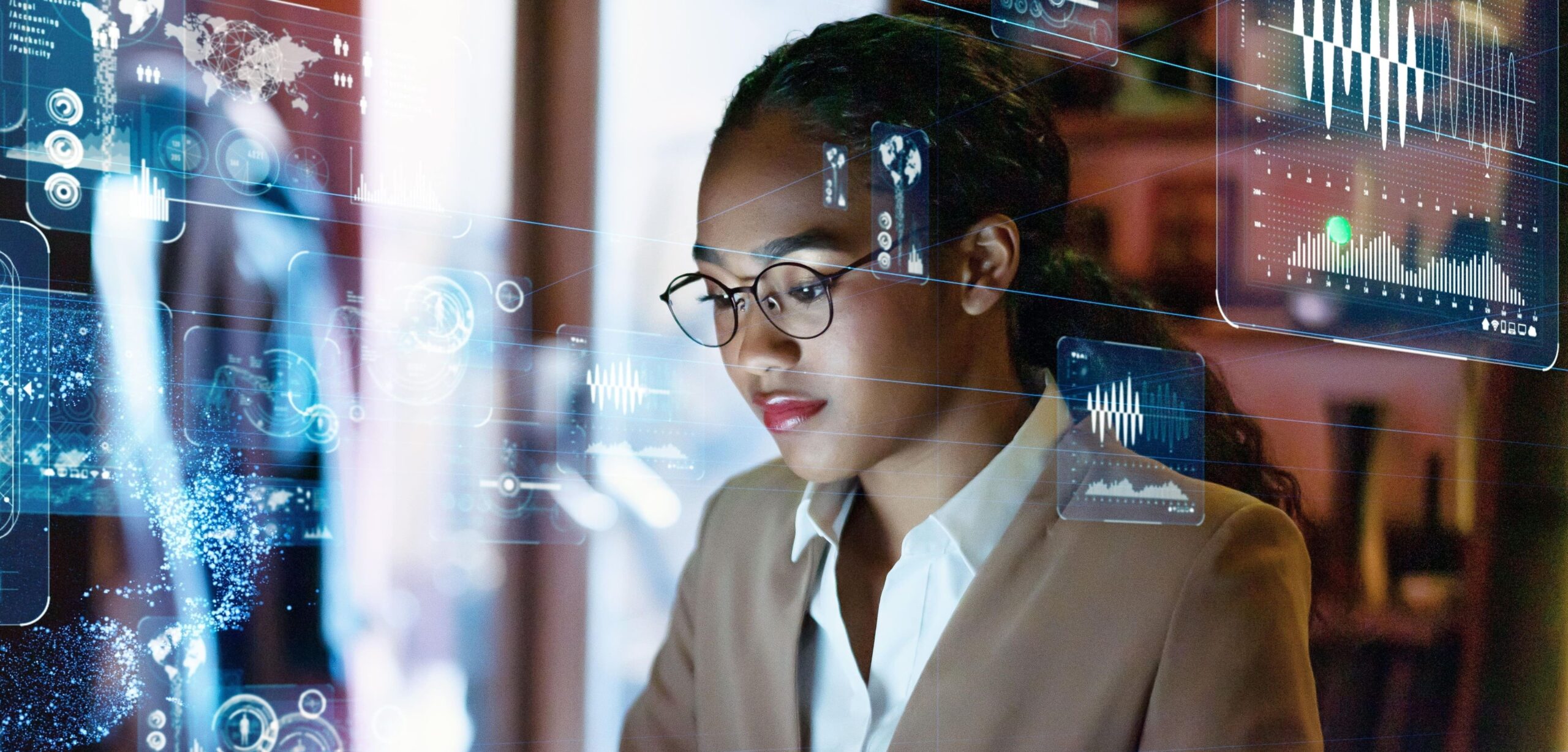
SAIF
Safe AI through formal methods
Preview
Use the vast knowledge accumulated over decades in formal methods to rethink them and address the new safety concerns raised by AI revival.
Caterina Urban, Research manager Inria
Zakaria Chihani, Deputy Director LAB, CEA List
The SAIF project aims to specify the behavior of ML-based systems, to develop methodologies to validate their large-scale performance, and to guide their design using formal approaches, in order to guarantee their safety, reliability and explicability.
Keywords : Machine learning, neural networks, reinforcement learning, recurrent networks, graph networks, Transformers, interpretability, robustness, constraint satisfaction, stability, fairness, explicability, reliability
Missions
Our researches
Develop the specification of ML-based systems
Develop formal methods to specify the behavior of ML systems, including the exploration of extensional specifications (defining global robustness properties), and intentional specifications (identifying recurring patterns).
Develop the validation of ML-based systems
Design methodologies to extend formal verification to large-scale systems, improving verification efficiency and accuracy while studying more complex architectures (e.g., inferring invariants of complex architectures such as recurrent neural networks).
Develop the design of ML-based systems
Use formal methods to automatically build ML components from proven specifications, developing monitoring approaches to maintain their reliability and facilitate their post-validation.
Consortium
CEA, Inria, Université de Bordeaux, Université Paris-Saclay, Institut Polytechnique de Paris
SAIF aims to revolutionize the entire development process for machine learning-based systems, from design to deployment. This involves integrating practical formal methods-based solutions to guarantee their security and reliability. Emphasis is placed on the development of explanatory approaches to facilitate exchanges with machine learning experts who are not necessarily familiar with formal methods.
In addition to publications and technical reports, the project aims to make the resulting software and demonstrators available as Open-Source software to the wider community. Scientific and interdisciplinary collaborations will be established at national and international level to promote the dissemination and adoption of the results.
SAIF will have a major societal impact by rigorously ensuring the safety of AI, which is necessary to fulfil its positive potential in society while minimizing potential risks. By developing Open-Source tools to assess and guarantee the reliability of ML systems, particularly in critical areas such as health and safety, the project will help to standardize and streamline the verification and validation processes required for the social acceptance of AI.
From an economy standpoint, SAIF will offer innovative methodologies for the design of ML systems that are better suited to verification, without compromising the accuracy of the models. This will open up new prospects for the use of AI in industries with high levels of technical requirements.
A community of 25 researchers, teaching researchers and permanent engineers, in addition to 17 PhD students, 8 post-docs and 3 contractual research engineers.
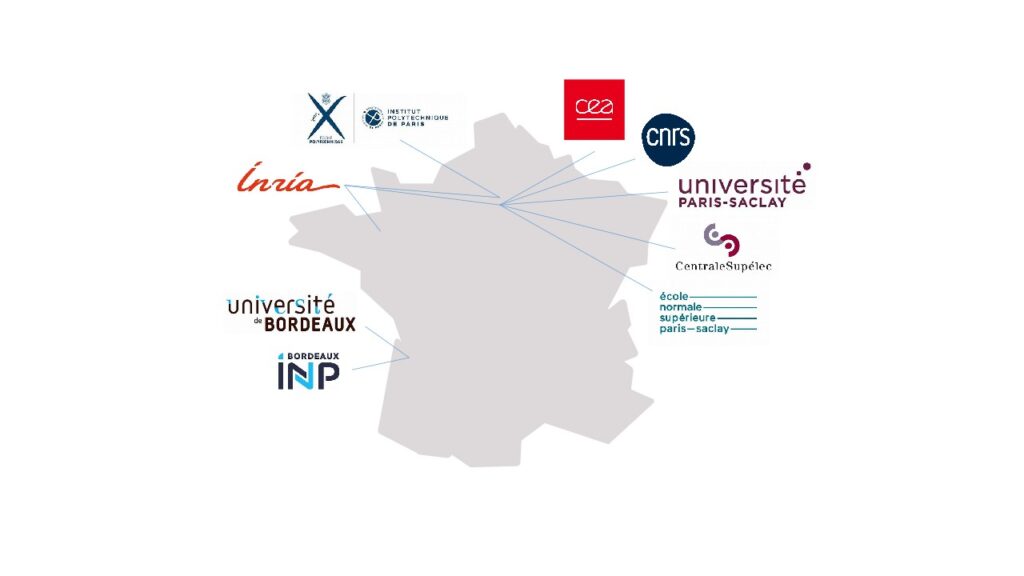
Autres projets
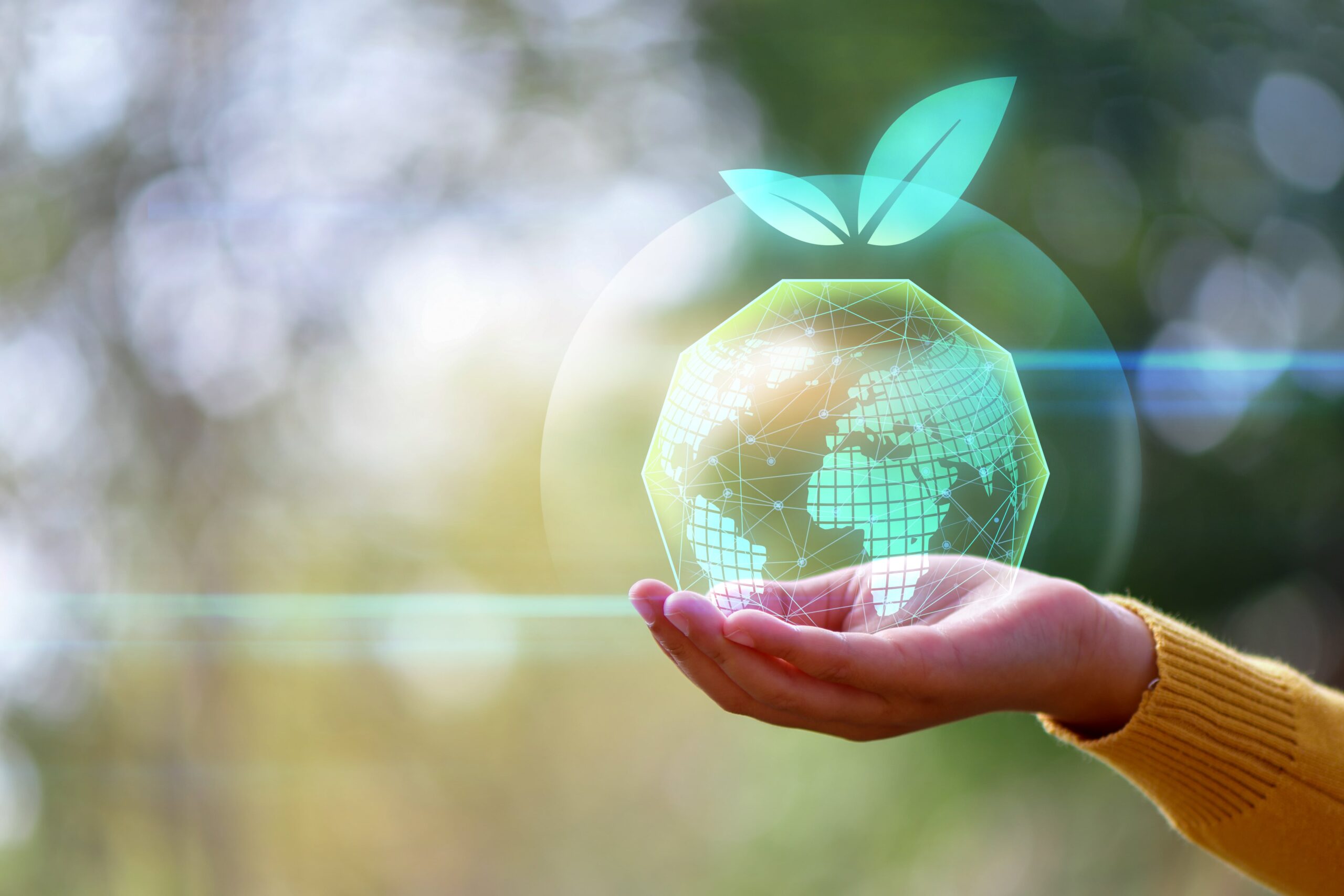
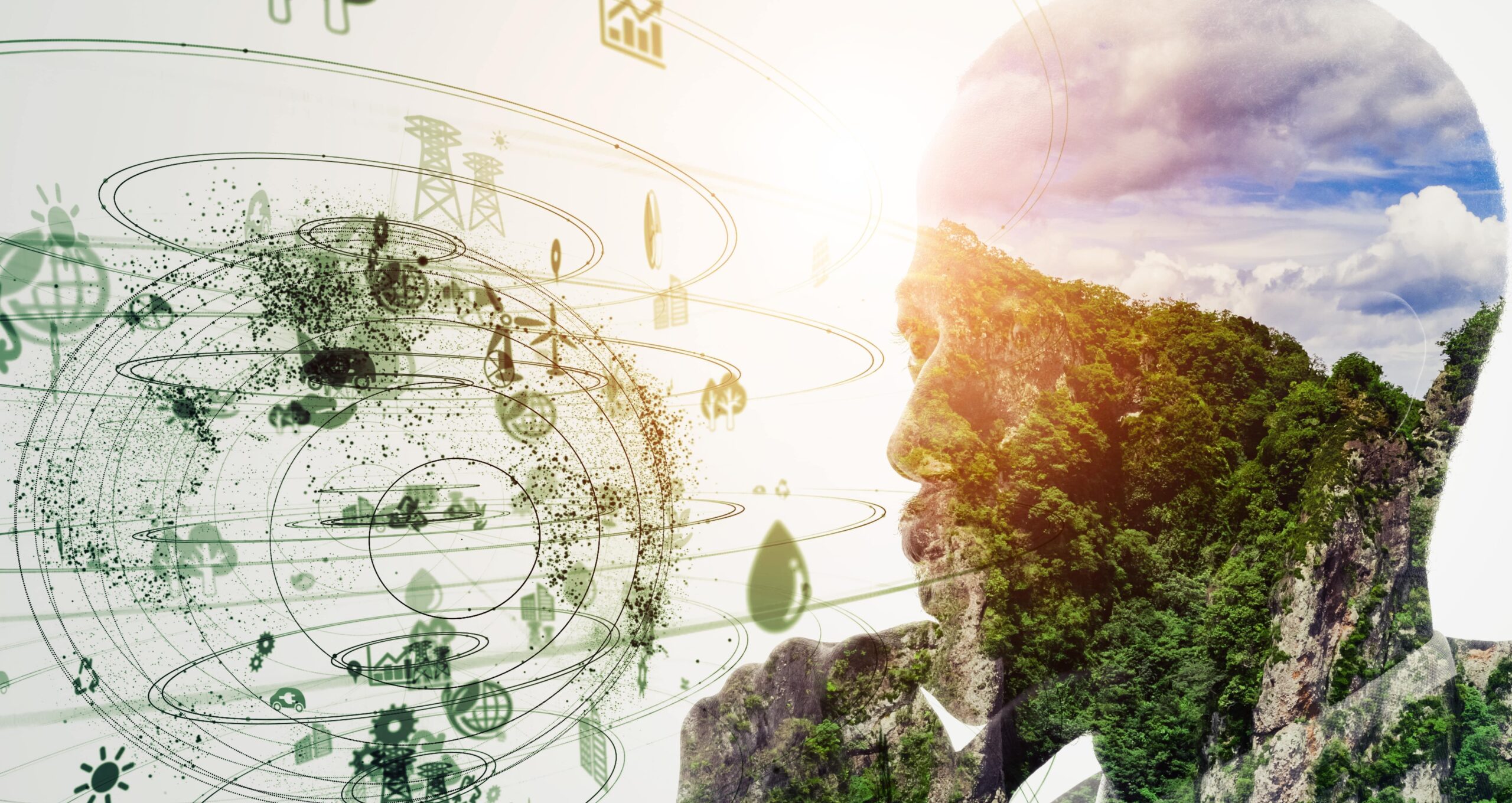
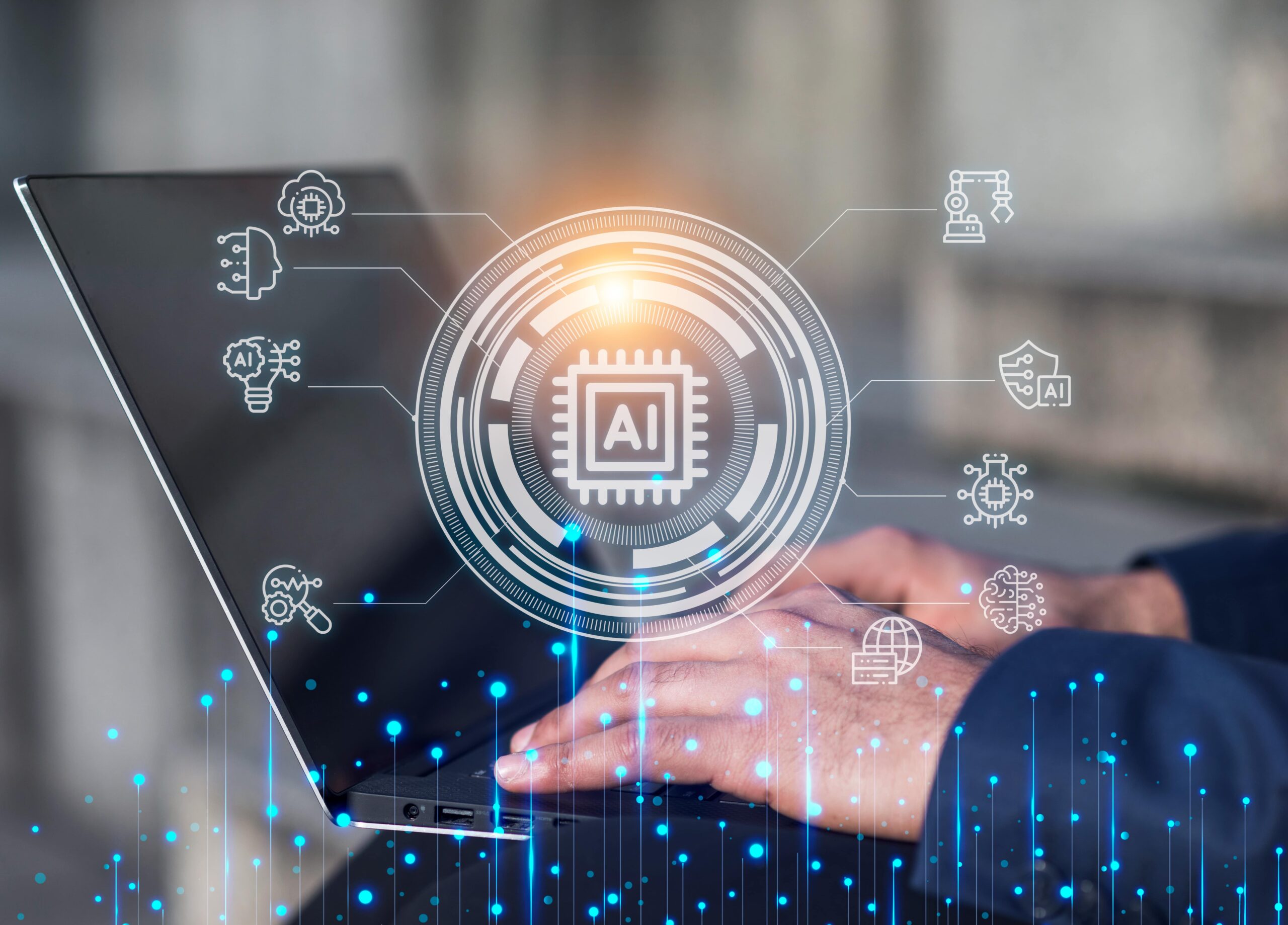
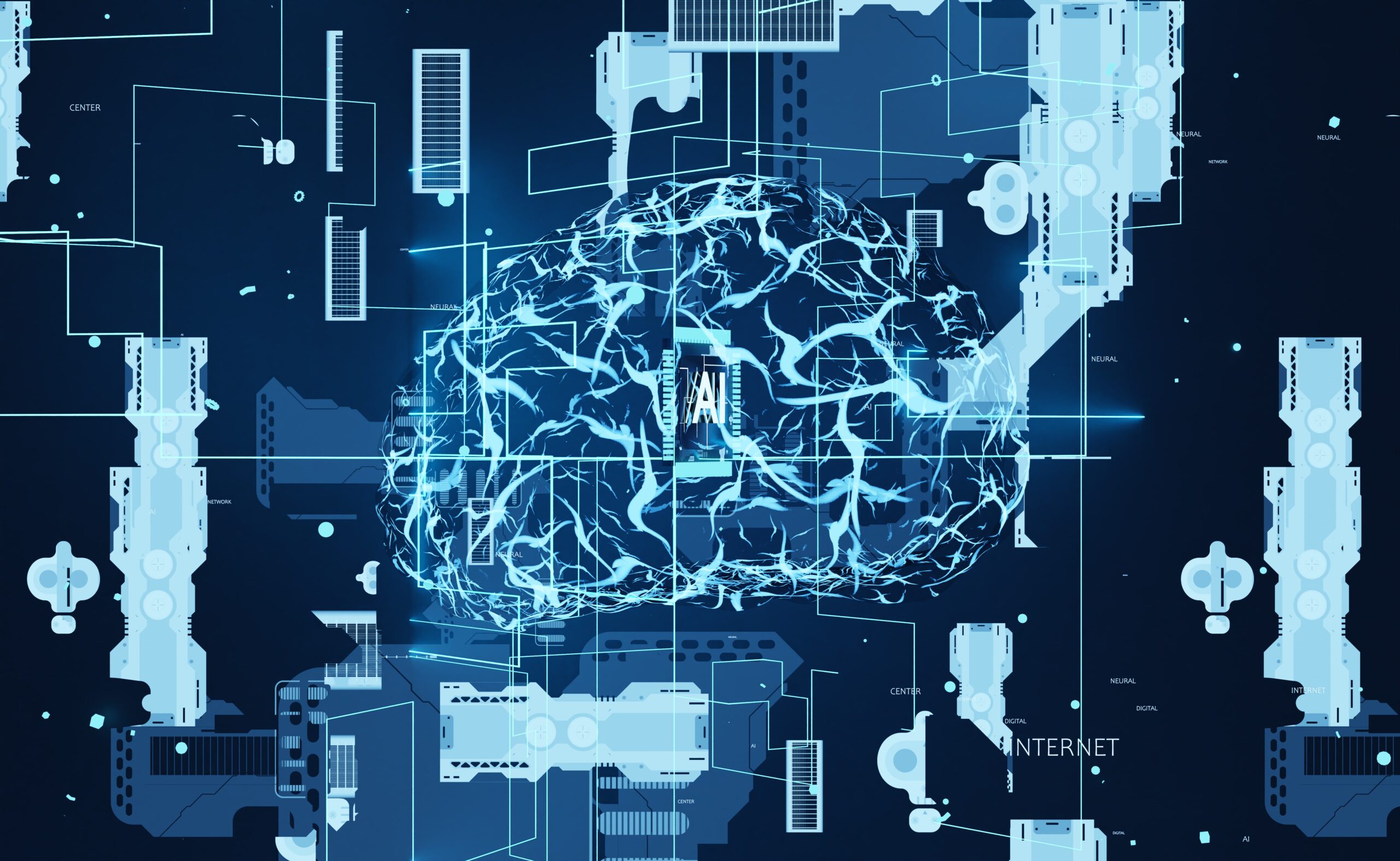
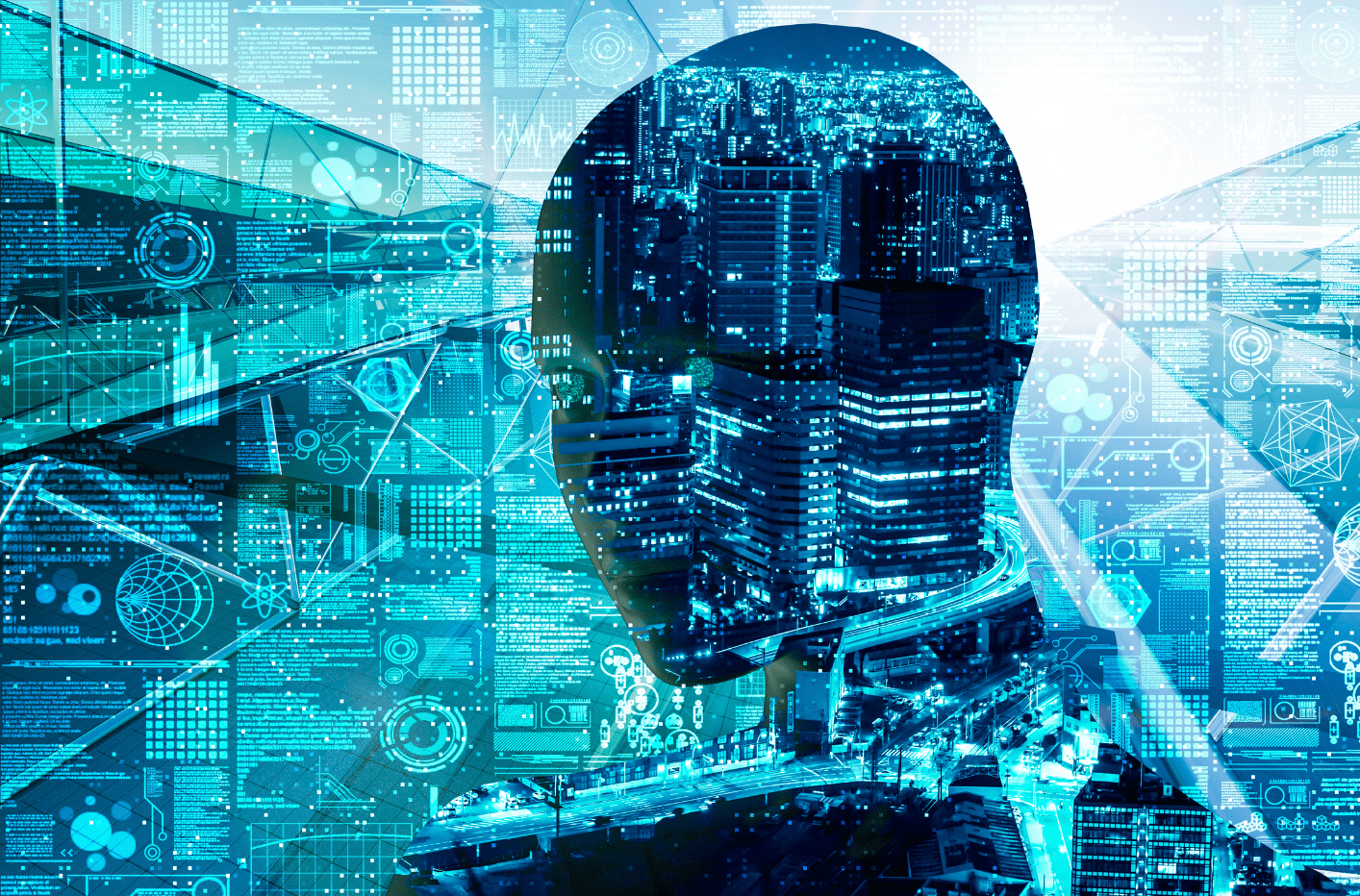
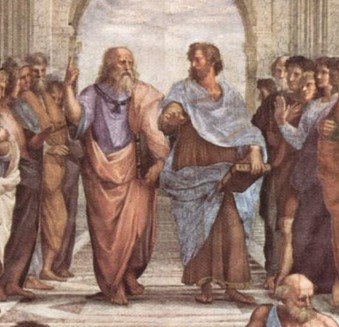
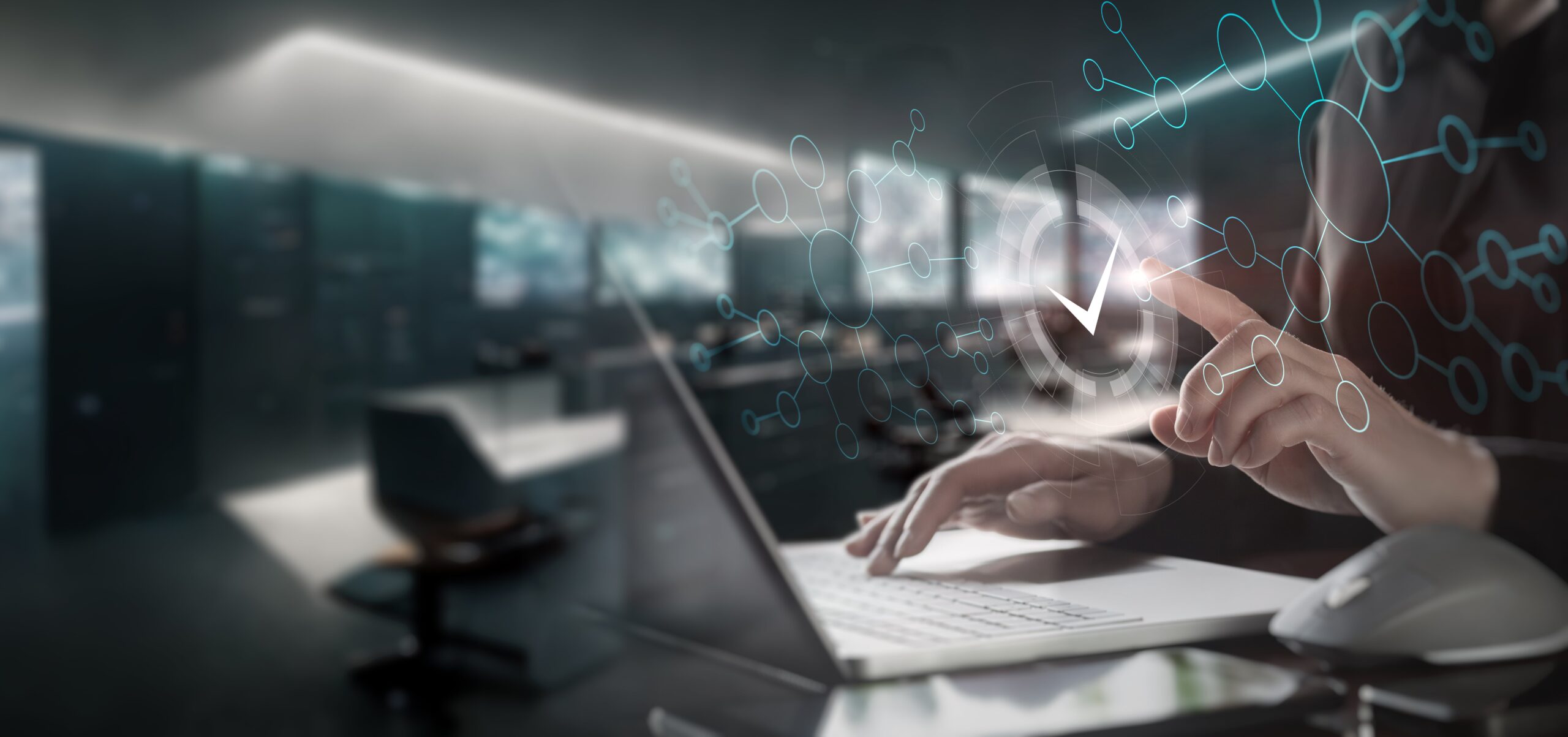
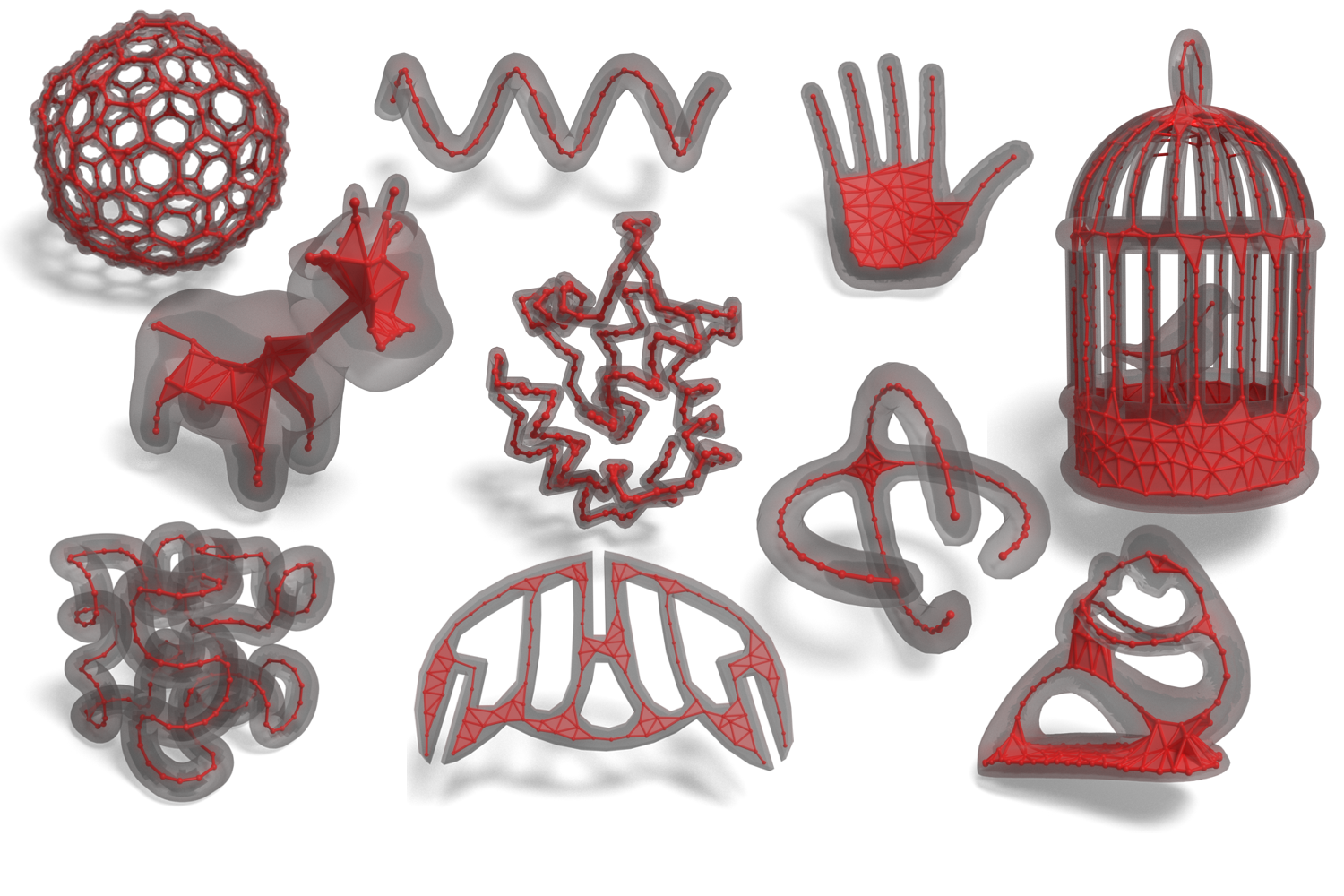