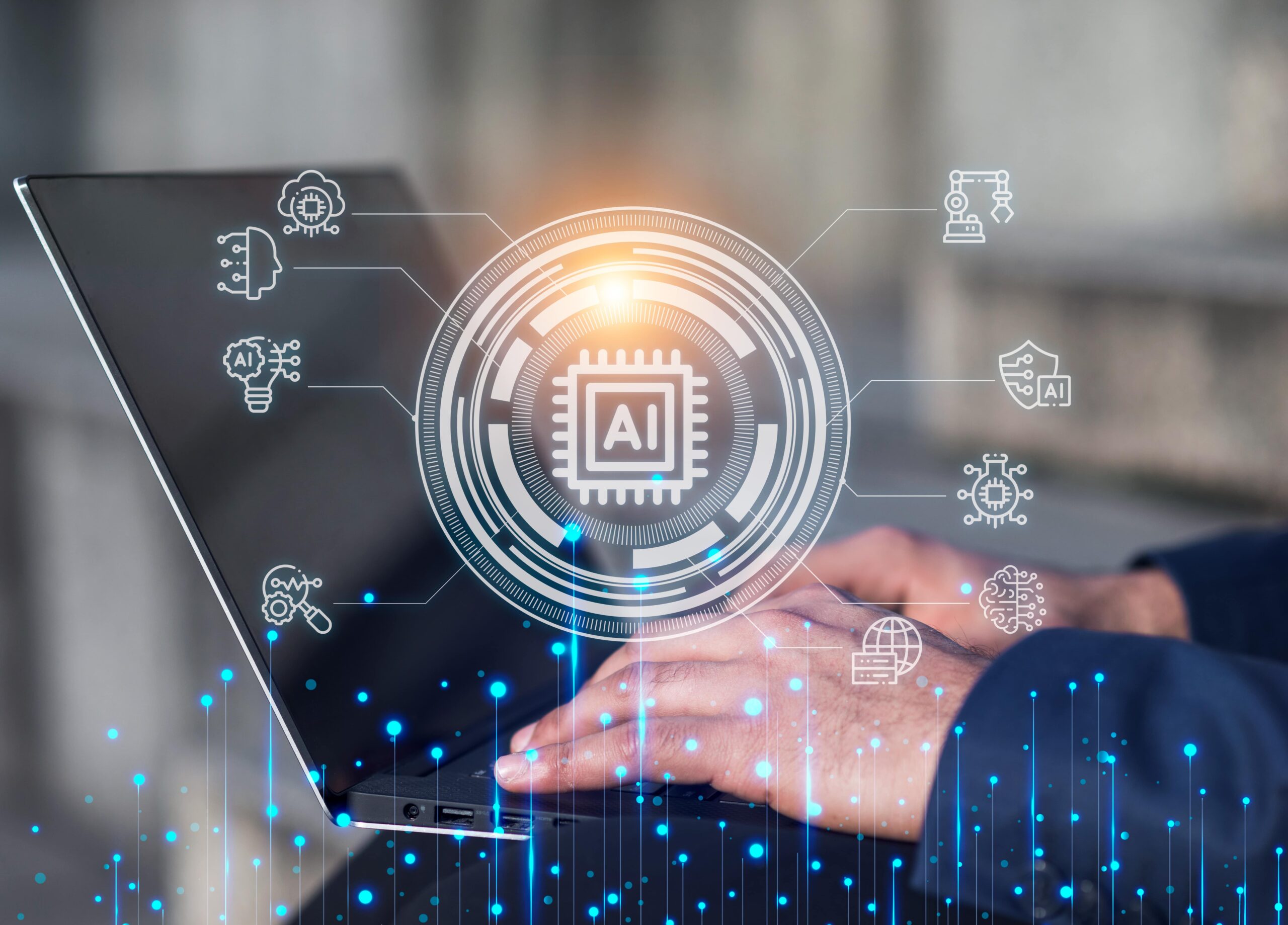
ADAPTING
Adaptive architectures for embedded artificial intelligence
Preview
An approach that goes further than current hardware architectures, with the aim of reaching the next generation of AI applications.
Alberto Bioso, Professor at Ecole Centrale Lyon
Ivan Miro Panades, Research engineer, CEA
The ADAPTING project proposes a new architectural paradigm adaptable to any AI application and its constraints in terms of precision, energy, latency and reliability. The adaptive architecture will be designed to ensure the flexibility, efficiency, durability and reliability of embedded AI. This approach goes beyond current hardware architectures and is aimed at the next generation of AI applications.
Keywords: Edge AI, Energy efficiency, Trusted AI, Computer Architectures, Embedded AI, Reconfigurable architecture, Reliable hardware implementation
Project website : projetAdaptING.ec-lyon.fr
Missions
Our researches
Flexibility
To be able to use the same hardware architectures to run different AI algorithms, taking into account application constraints in terms of accuracy, energy consumption and reliability.
On-chip learning
Enable adaptive learning in the cloud or close to the sensor to limit data transfer and preserve confidentiality, while reducing energy consumption.
Energy efficiency
Design frugal computing components and hardware architectures that significantly reduce data transfer between memory and computers.
Reliability
Exploit the specific features of hardware architectures to develop low-cost, real-time fault tolerance strategies for detecting, diagnosing and correcting hardware errors.
Consortium
CEA, Ecole Centrale de Lyon, Sorbonne Université, Université de Rennes, Université de Nantes, Université Bretagne Sud, CNRS, INSA Lyon, Ecole Supérieure de Physique Chimie Electronique de Lyon
- Specifications for the creation of new generations of adaptive hardware architectures for embedded AI.
- Development of virtual platforms to simulate these architectures and evaluate their performance in terms of flexibility, energy consumption, on-chip learning and robustness.
- Comparison of these architectures with the state-of-the-art.
The proliferation of connected objects is generating a growing need for artificial intelligence to process data as close as possible to the sensors. This trend entails major constraints in terms of data confidentiality, bandwidth, energy consumption and latency, particularly for critical real-time applications.
The ADAPTING project proposes a new, scalable architectural paradigm for designing integrated AI hardware solutions that are flexible, efficient, durable and reliable.
A community of 68 permanent researchers, research professors and engineers, plus 25 PhD students, 2 post-docs, 3 contract research engineers, 1 work-study student and 10 interns as the project progresses.
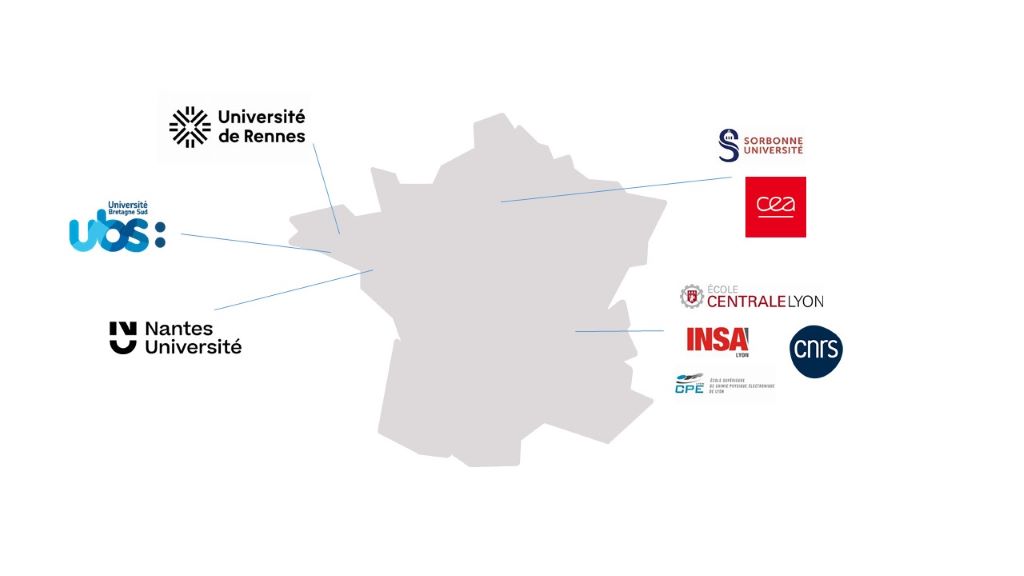
Publication
Autres projets
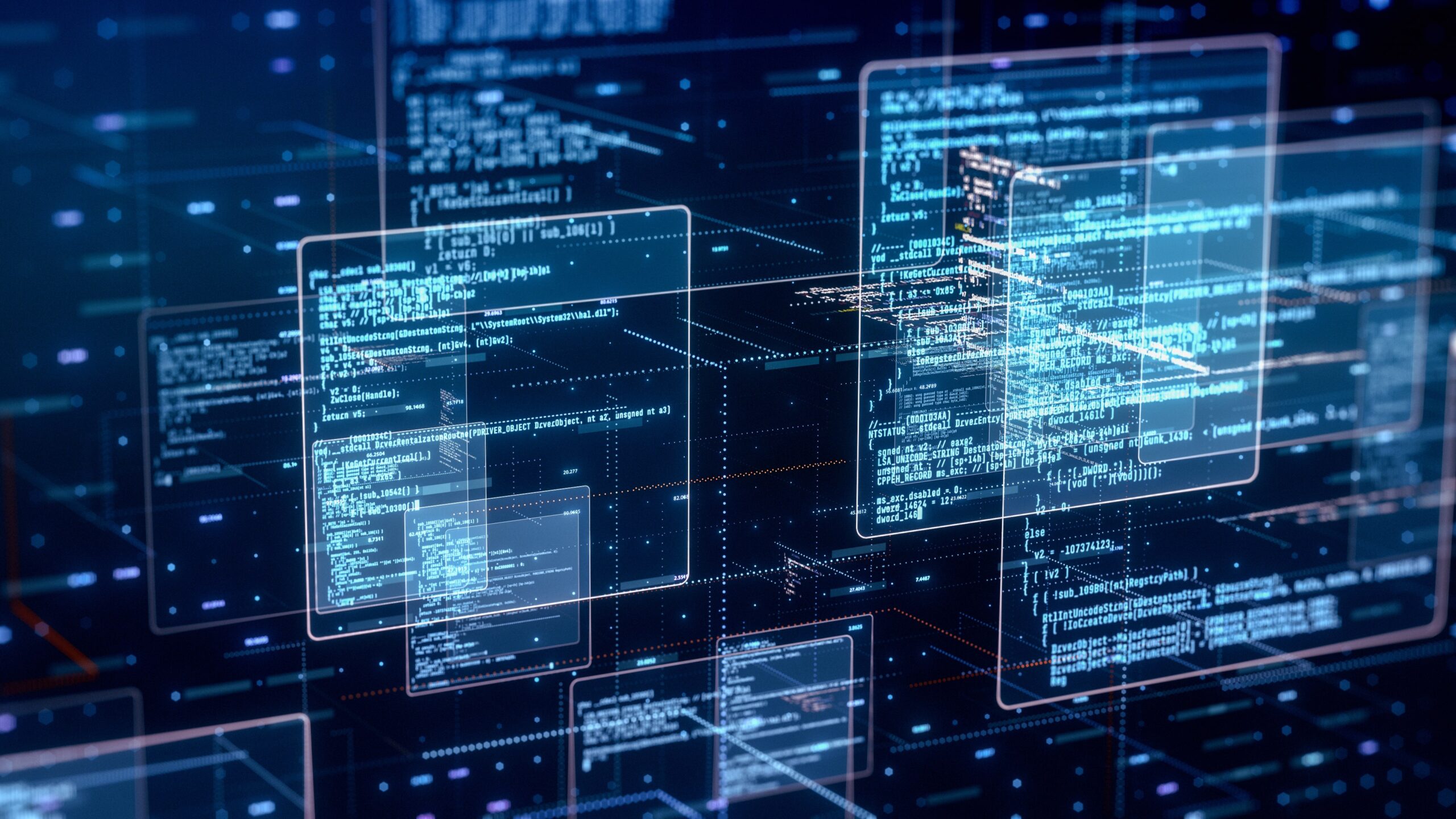
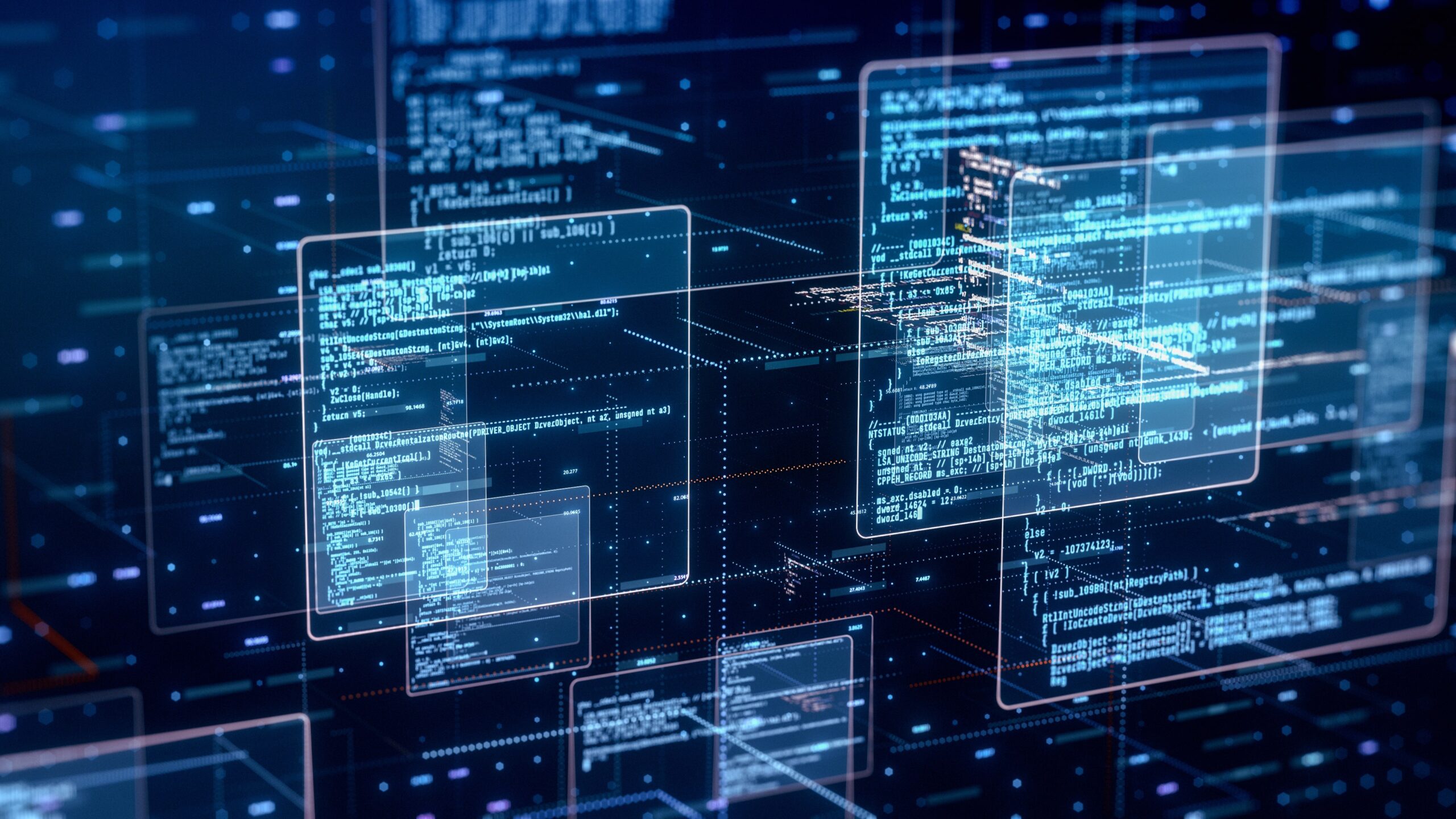
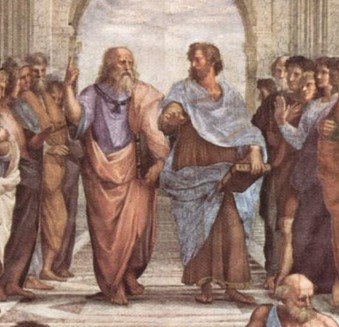
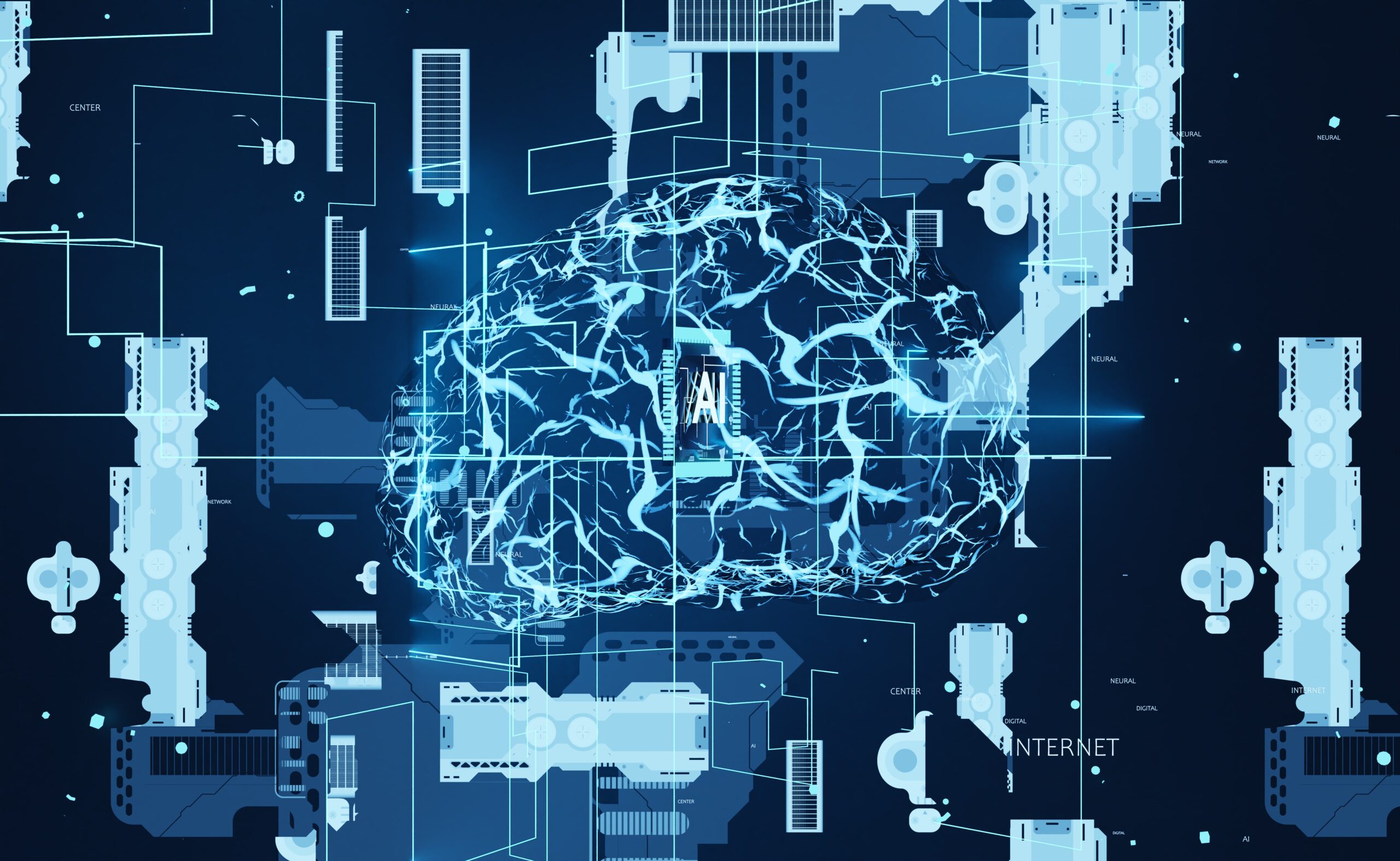
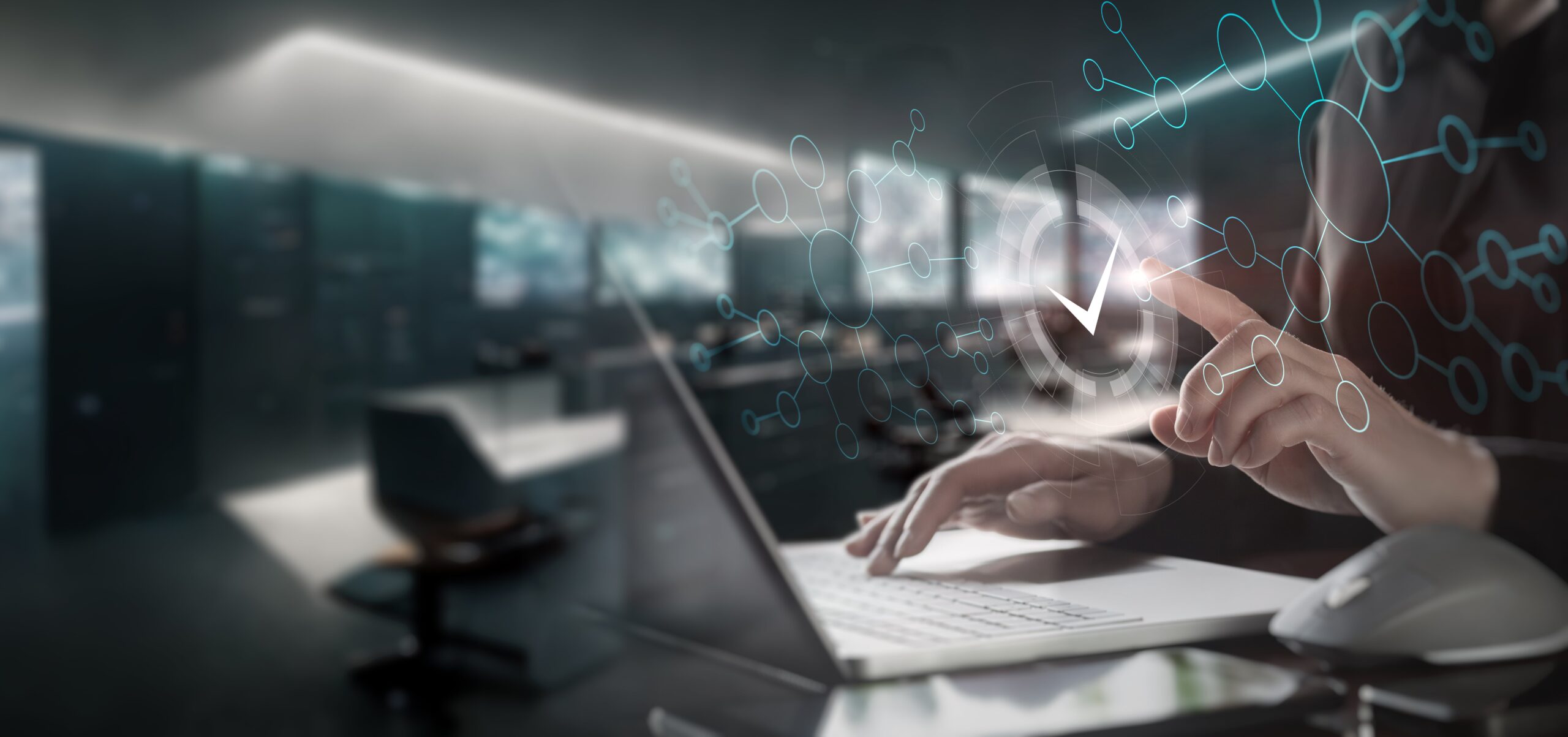
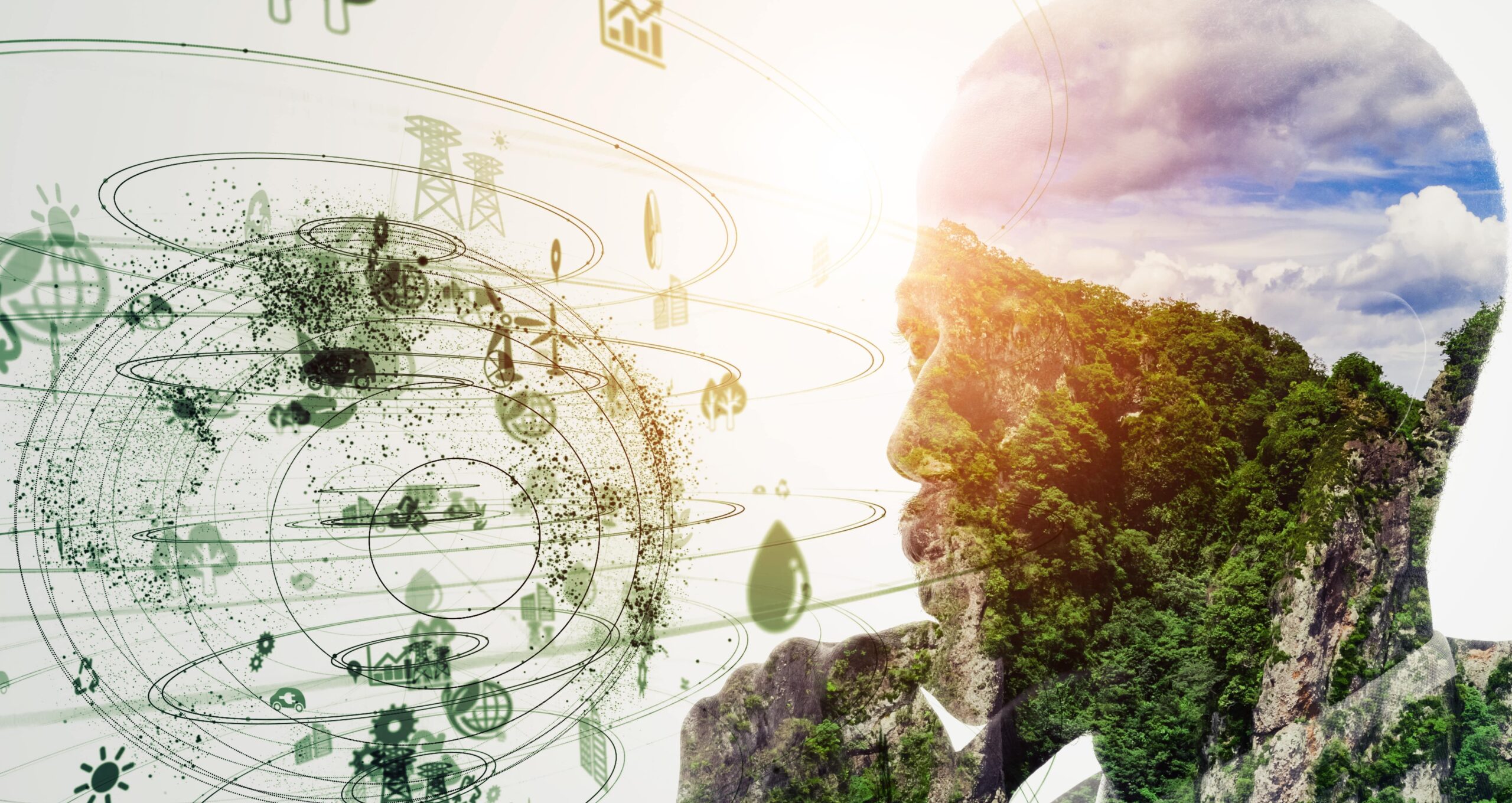
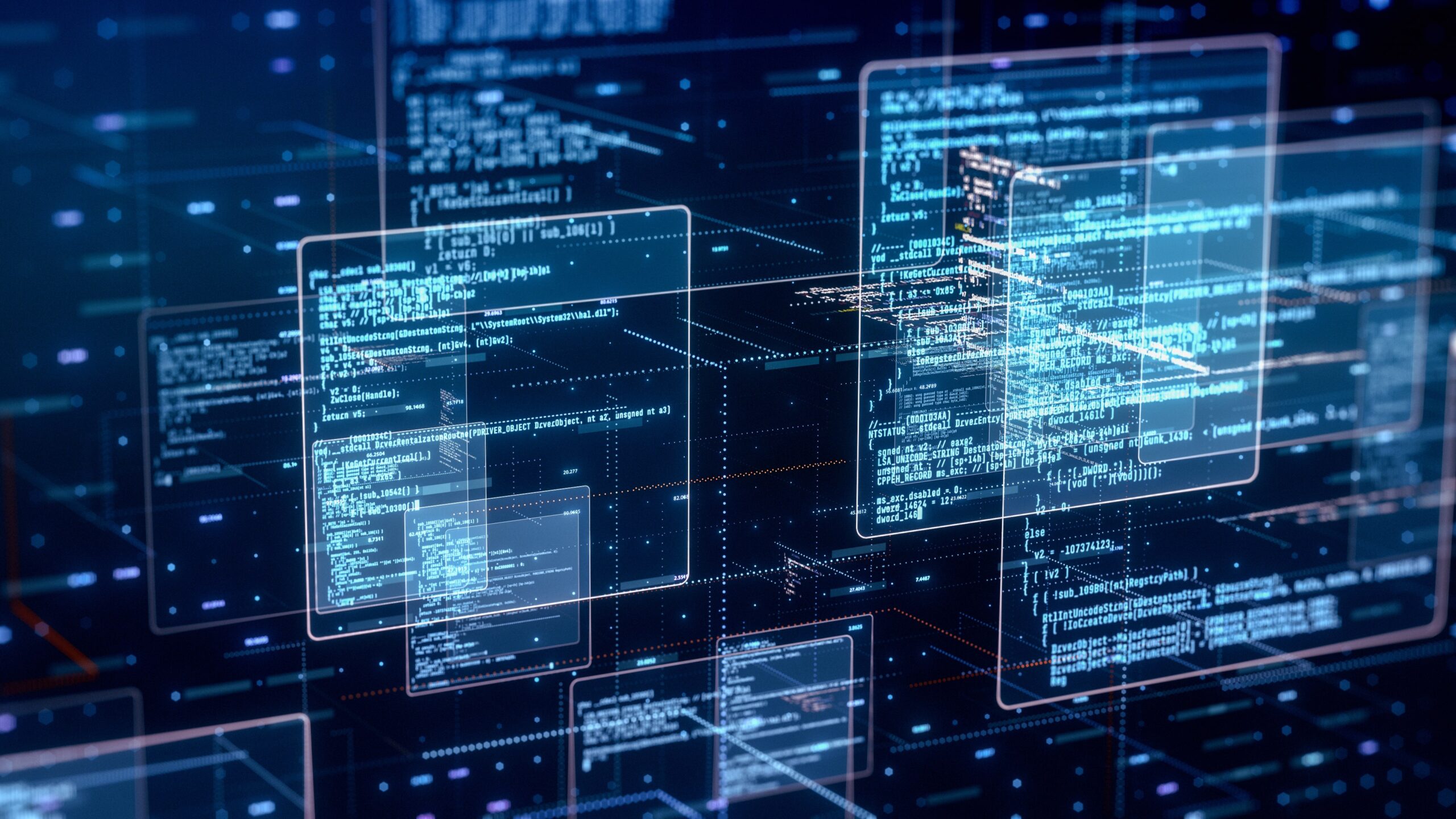
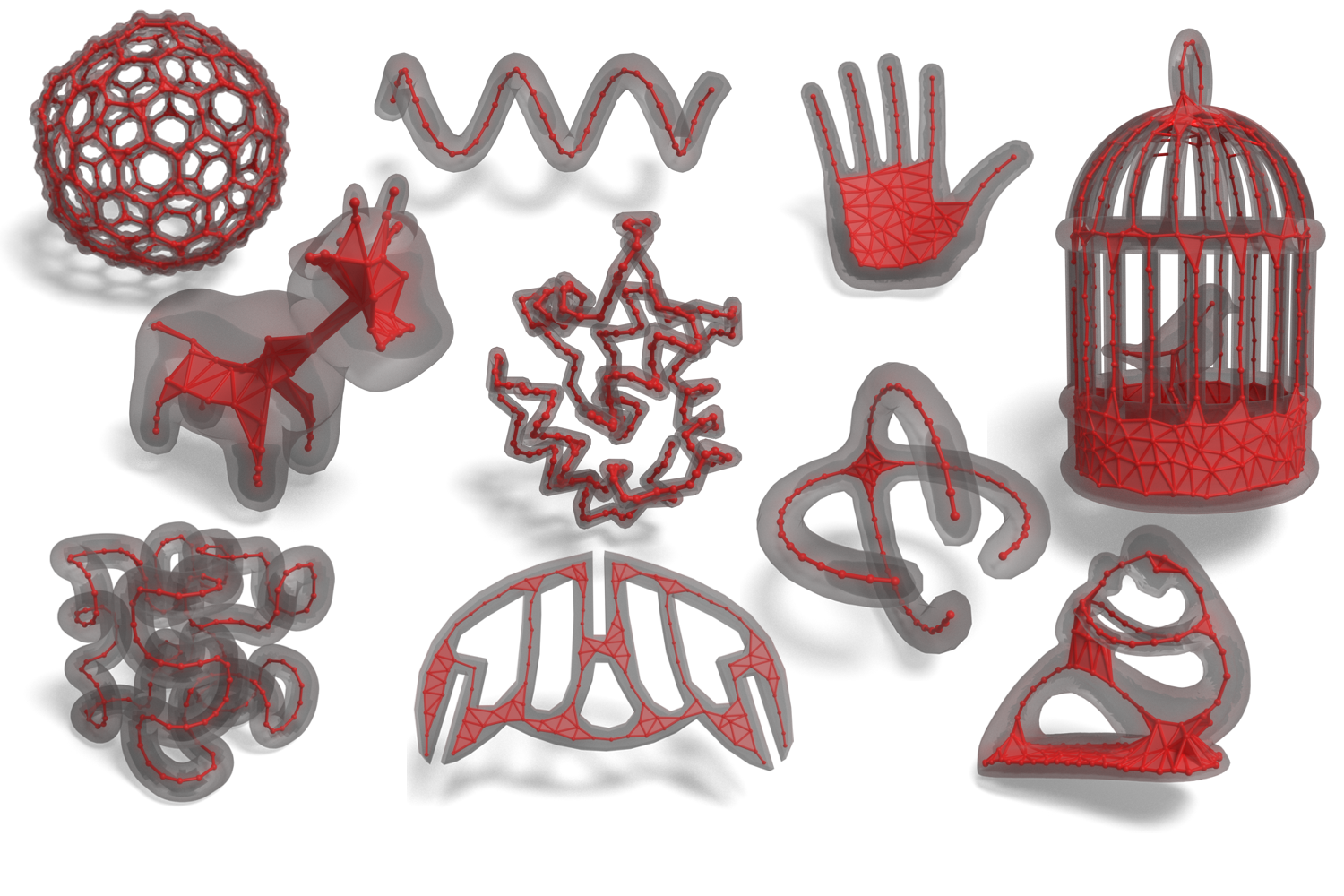
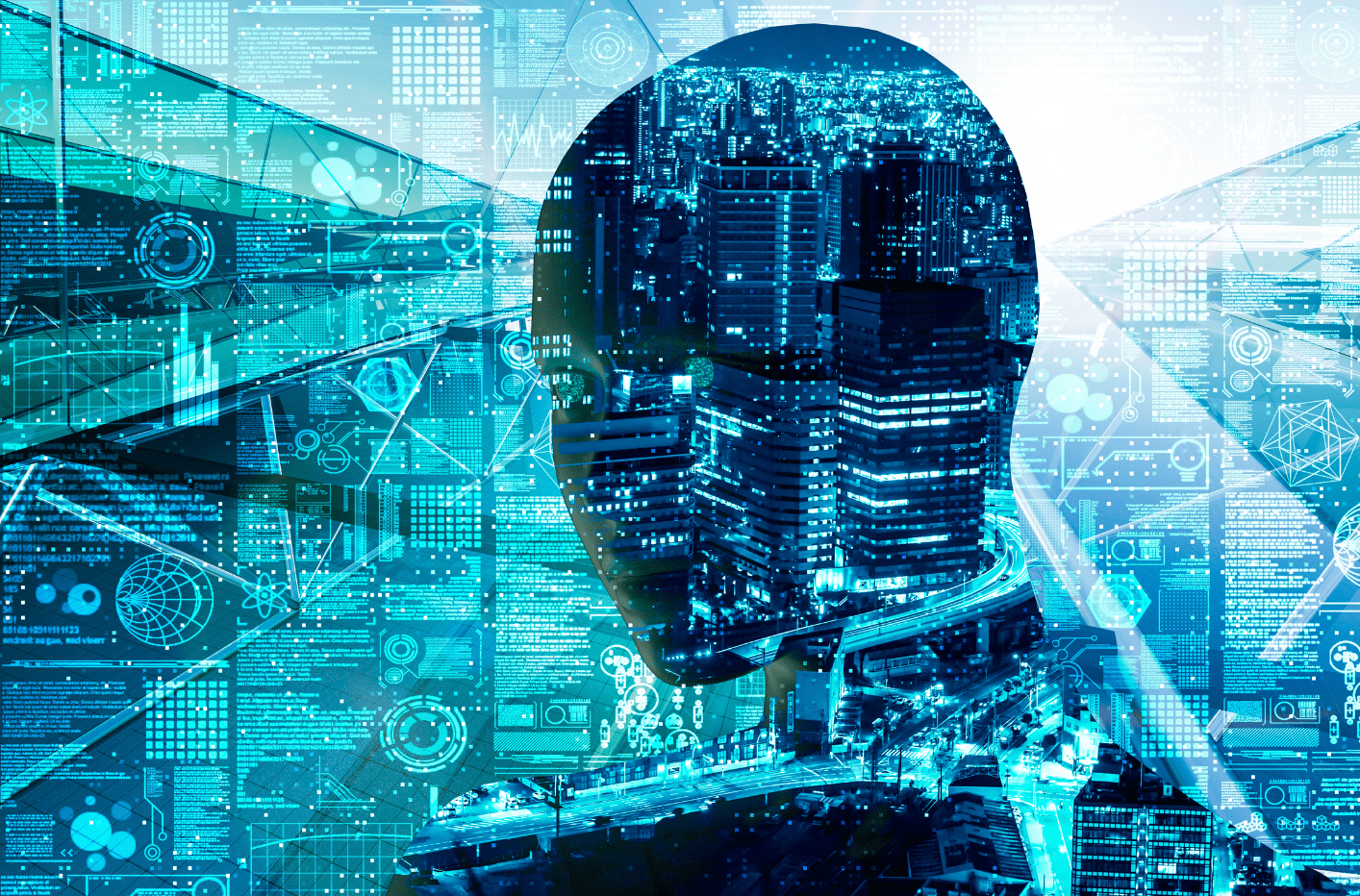
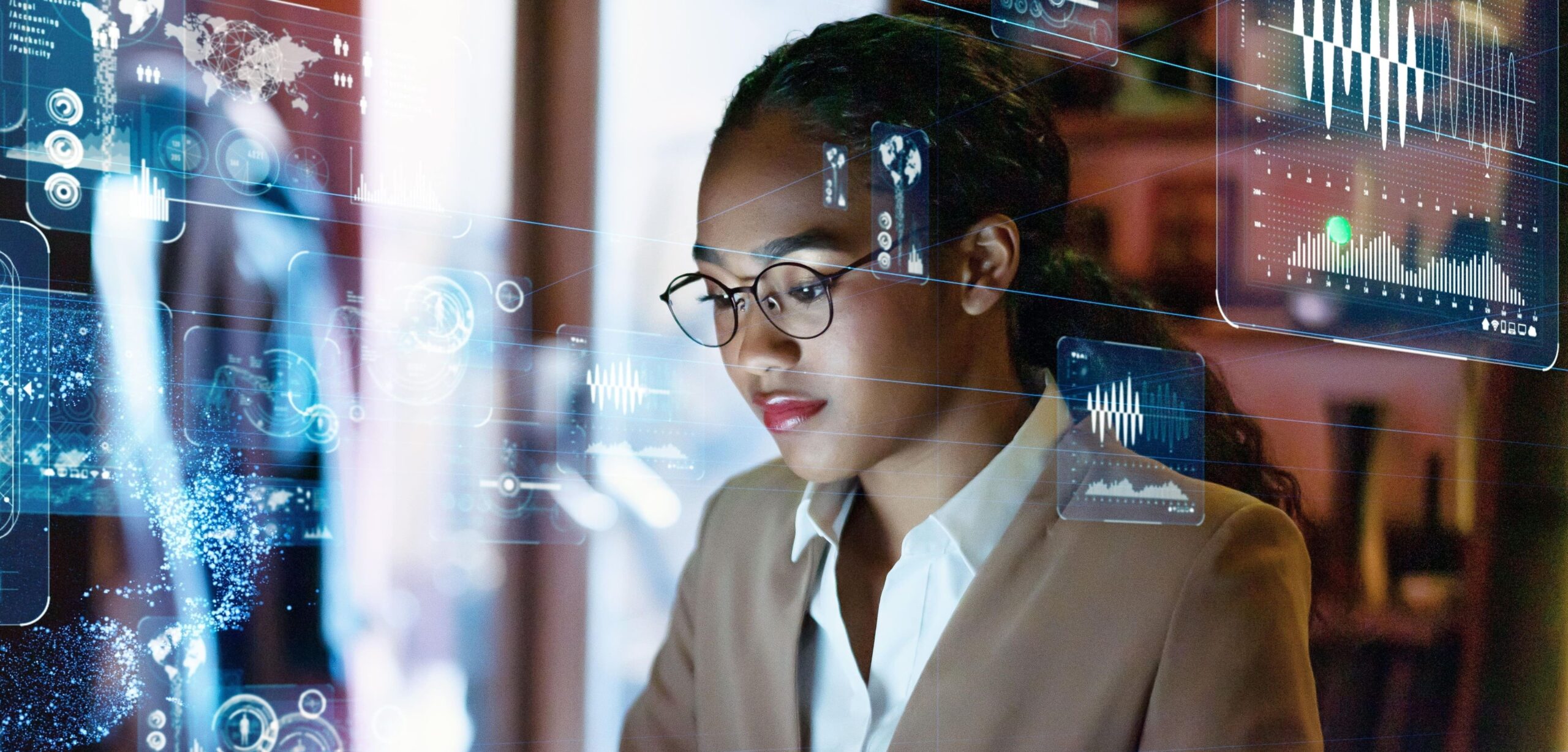
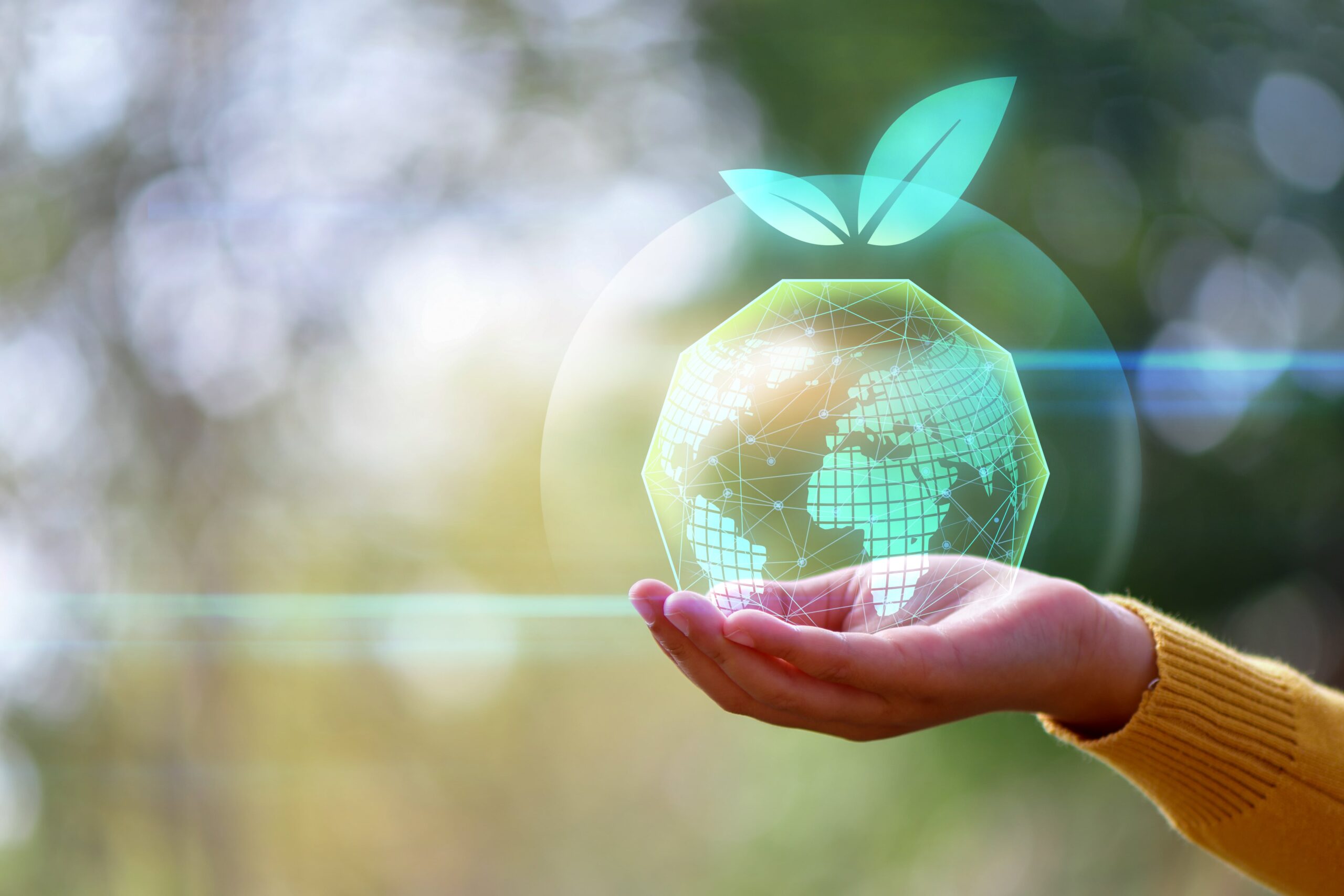